ARC-learning: A Self-tuning Cache Policy under Dynamic Popularity
2022 IEEE 8th International Conference on Computer and Communications (ICCC)(2022)
摘要
With the development of content applications, CDN servers have begun to carry more and more network traffic. Cache policy determines which object to store plays an essential role in the edge server's performance, and, in recent years, people have begun to focus on cache design through learning-based algorithms to adapt to the more varied scene and achieve better performance than heuristic algorithms. However, these algorithms have assumed that the objects they predicted have sufficient past information to use, which leads to poor performance when the load popularity rapidly changes. Motivated by this scene, we propose a cache strategy combining a learning-based method with a self-tuning strategy to improve the poor performance of the learning-based method under the above situation. We verify our strategy using real traces (from YouTube and Wikipedia) and synthetic traces with different dynamic levels, and the simulation results show that ARC-learning achieves the best performance compared with other algorithms in both static and non-static environments.
更多查看译文
关键词
cache,dynamic popularity,learning-based,self-tuning
AI 理解论文
溯源树
样例
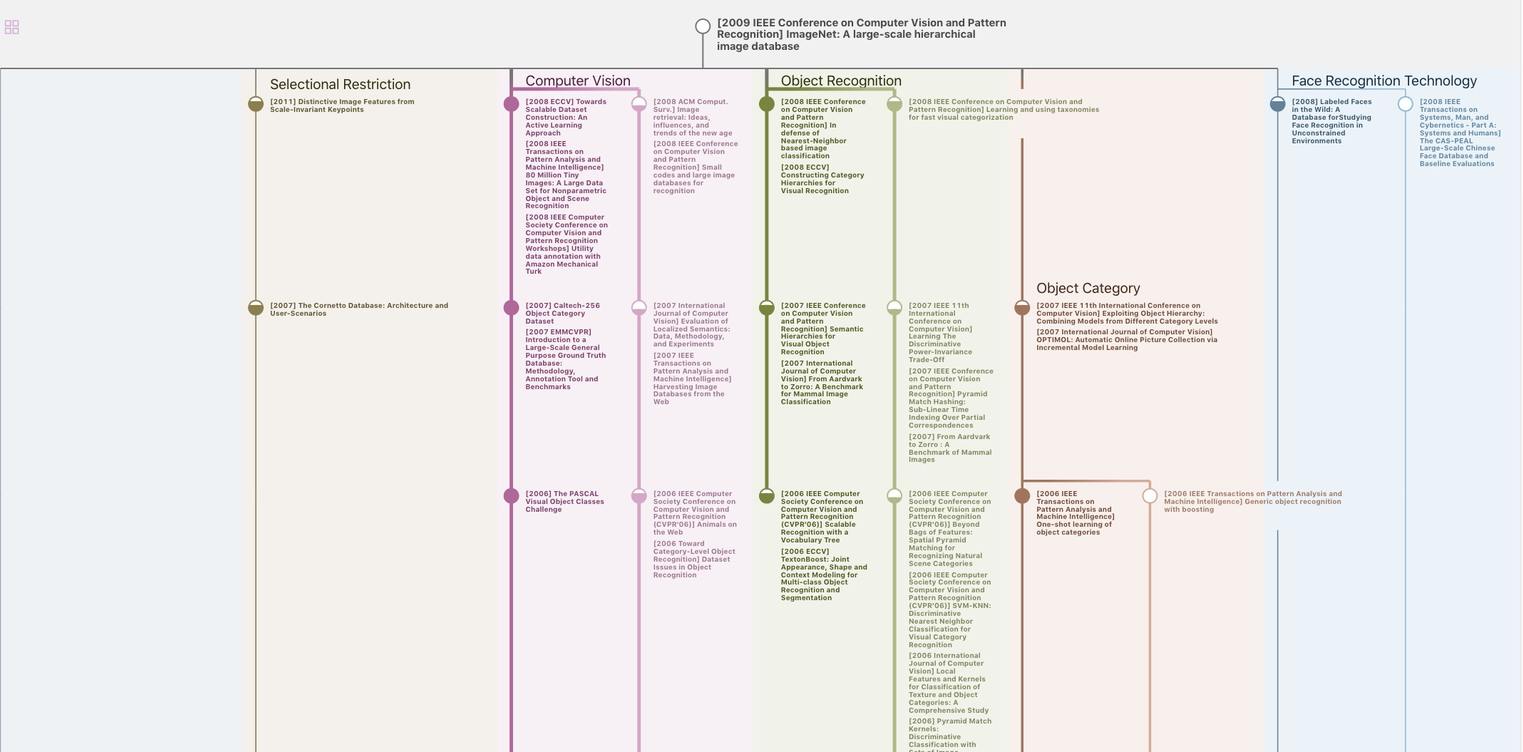
生成溯源树,研究论文发展脉络
Chat Paper
正在生成论文摘要