Research on Network Traffic Anomaly Detection Method Based on Temporal Convolutional Network
2022 IEEE 8th International Conference on Computer and Communications (ICCC)(2022)
摘要
In recent years, with the exponential growth of network traffic and the increase of network attacks, anomaly detection of network traffic has always been a hot research issue. Many network traffic anomaly detection studies focus on the temporal characteristics of network traffic. However, most of these studies start from statistical characteristics and pursue the accuracy of network traffic anomaly detection, which lead to the lag of network traffic anomaly detection. In this paper, a network traffic anomaly detection method combining temporal convolution network and dynamic time warping algorithm is proposed to solve the lag problem of network traffic anomaly detection. The predictor trained by temporal convolution network can predict what the user's next benign behavior series should be like from the user's current behavior series, and then the dynamic time warping algorithm can judge the abnormal user by calculating the similarity between the user's actual behavior series and the predicted behavior series. We carried out our verification experiment on the original pcap file of CICIDS2017 dataset, and finally achieved an average negative sample recall rate of 92% and a false alarm rate of less than 40%. This result is ideal on the premise of ensuring timeliness. It can be used as the first level screening system of the intrusion detection system to screen possible criminal attackers in time, so that network security managers can make timely security measures. We will continue to improve our research to reduce false positives.
更多查看译文
关键词
intrusion detection,network traffic,deep learning,timely detection,temporal convolutional network,dynamic time warping
AI 理解论文
溯源树
样例
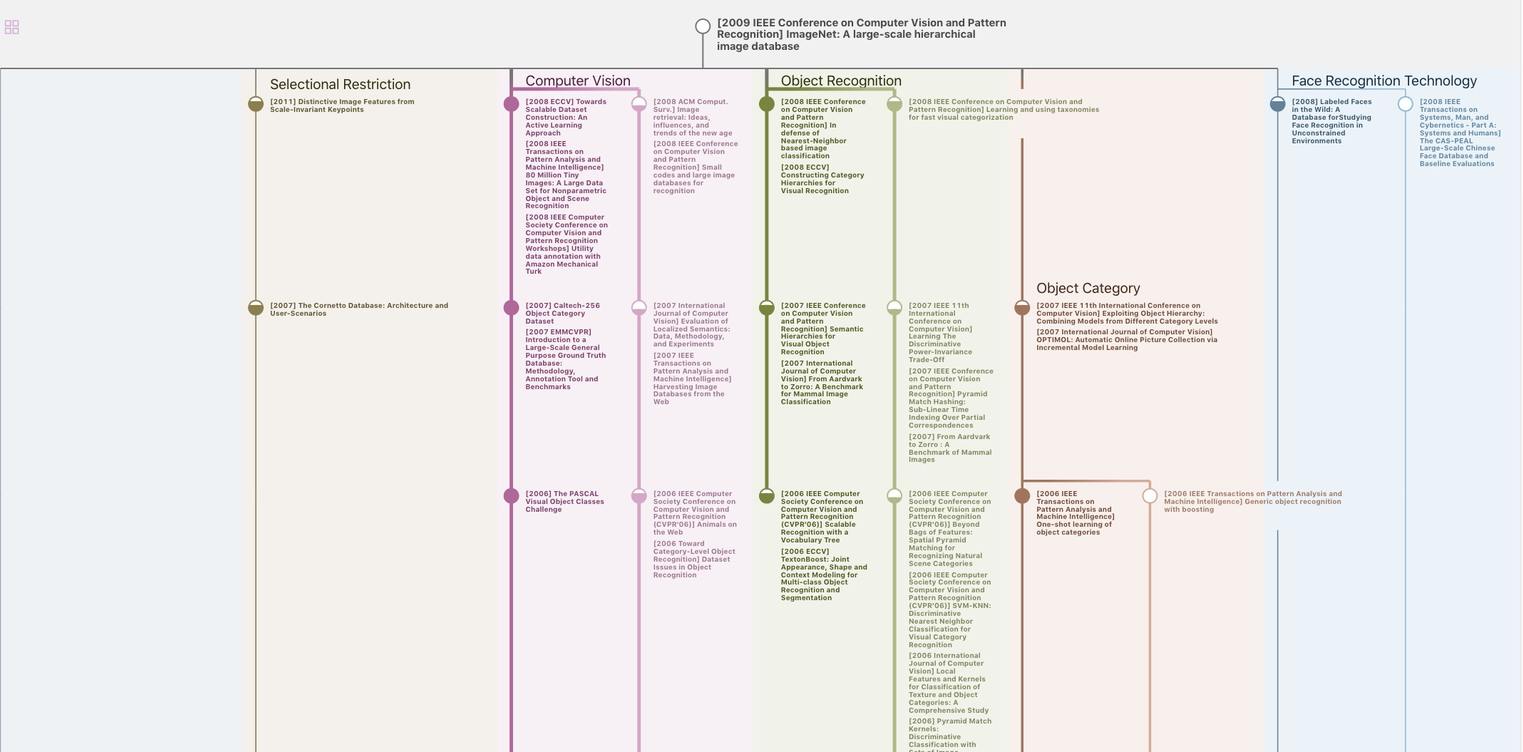
生成溯源树,研究论文发展脉络
Chat Paper
正在生成论文摘要