Benchmarking Artificial Neural Network Models for Design Technology Co-optimization
2023 International Symposium of Electronics Design Automation (ISEDA)(2023)
摘要
Device modeling with artificial neural networks (ANN) has been explored in the community. However, figure of merits of ANN models in application scenarios have not been fully validated. In this work, benchmarking of ANN models for design technology co-optimizations of emerging device technologies is performed, in terms of model accuracy, simulation accuracy and speed. First, an approach to address the model discontinuity at the boundary between forward and reverse transistor operation mode in ANN-based compact model (CM) is developed, enhancing the ANN model to pass the Gummel symmetry test (GST). Novel loss functions are then developed for current and charge models with improved accuracy, i.e. within 2% error. Finally, designs and characterizations of GAA standard cell libraries are performed with the ANN model in comparisons to physics-based models. It is found that simulations with the ANN model achieve high accuracy in delay and power consumption predictions within 1% error and speedup by up to 4 times.
更多查看译文
关键词
Compact model,Artificial neural network,Semiconductor device simulation
AI 理解论文
溯源树
样例
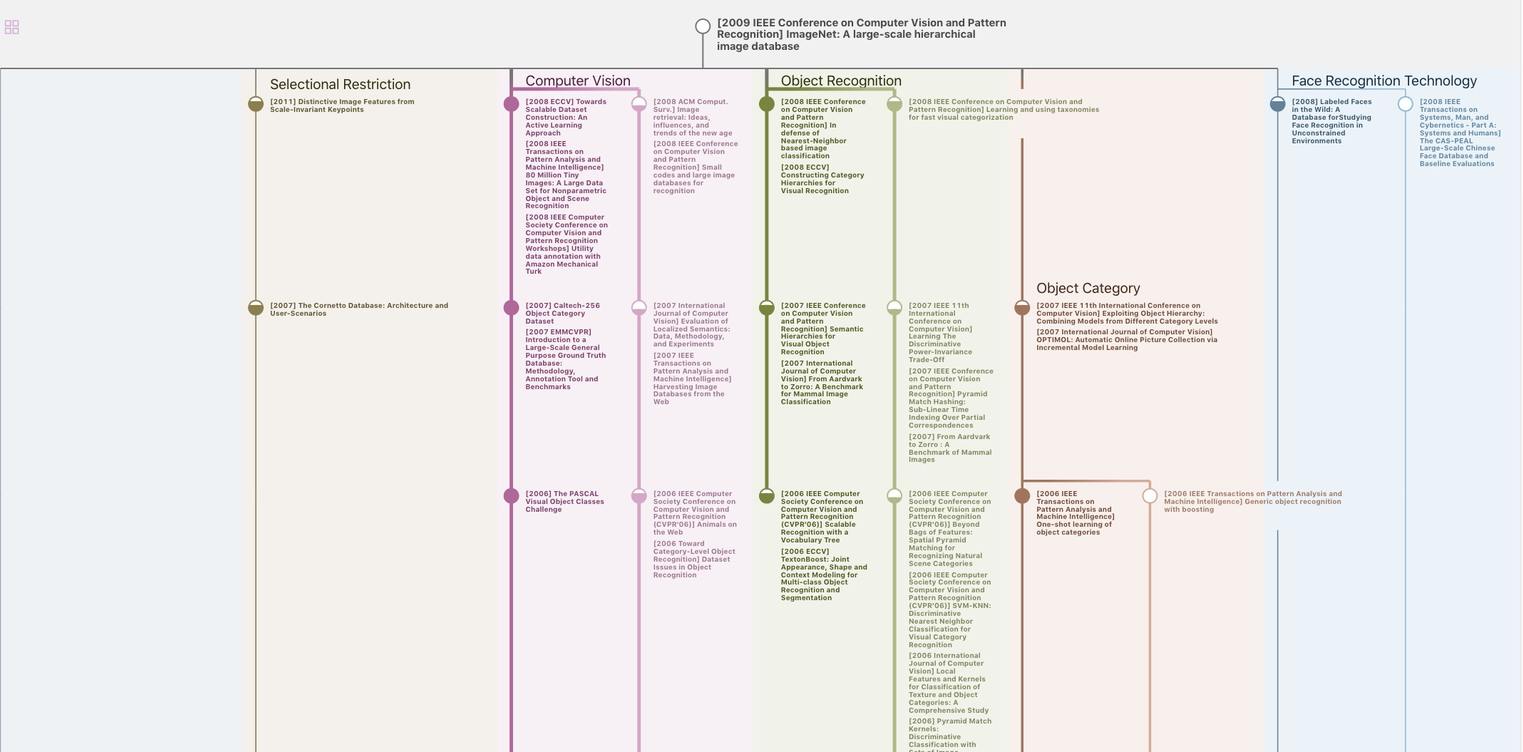
生成溯源树,研究论文发展脉络
Chat Paper
正在生成论文摘要