Data-Efficient Pin Defect Detection with Transformer in Transmission Lines
2023 5th International Conference on Electronic Engineering and Informatics (EEI)(2023)
摘要
Pin defect is an important component of high-voltage line safety inspection. Due to the harsh environment of the power lines, large-size dataset of pin defects is hard to obtain. DEtection TRansformer (DETR) have achieved great success on large-size datasets, but perform poorly on small-size datasets. In this paper, we found that the reasons why DETR-like methods perform poorly on small-size datasets is that there is too much redundancy in the queries in the encoder, making it difficult for the model to attend to sparse objects. Secondly, the object queries in the decoder are randomly initialized and difficult to learn object features. To address these issues, we propose a data-efficient Deformable DETR (DE-Deformable DETR), which includes a sparse encoder and a feature sampling decoder. The sparse encoder reduces computational complexity and accelerates convergence, while the feature sampling decoder uses cascaded sampling for efficient feature extraction. Extensive experiments show that DE-Deformable DETR can improve the performance of DETR-like methods on two small datasets and achieve optimal results.
更多查看译文
关键词
component,Pin defects,DETR,large-size dataset,small-size dataset
AI 理解论文
溯源树
样例
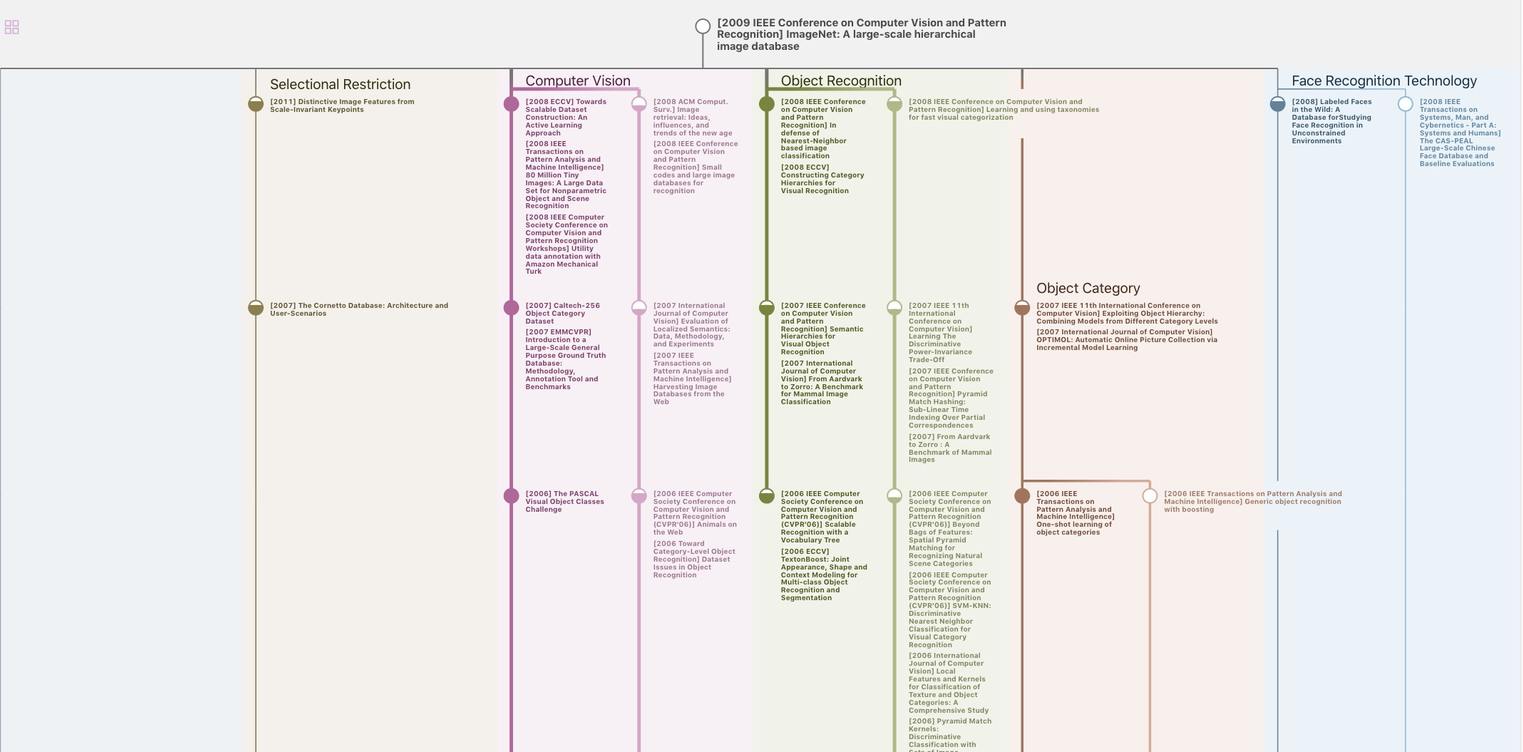
生成溯源树,研究论文发展脉络
Chat Paper
正在生成论文摘要