Sequential Abruptly Changing Hidden States Estimation using Adaptive Particle Impoverishment Mitigation Scheme
2023 Joint International Conference on Digital Arts, Media and Technology with ECTI Northern Section Conference on Electrical, Electronics, Computer and Telecommunications Engineering (ECTI DAMT & NCON)(2023)
摘要
Particle filtering (PF) is a sequential Monte Carlo (SMC) method that infers the states of the hidden parameters of interest from the posterior probability distribution functions (PDFs) given the noisy measurement data obtained from any non-linear systems. Each sample of parameter states (called particle) is randomly drawn, so their likelihoods can be different from each other and particle degeneracy may happen. Resampling eliminates the particles that have low likelihoods and replicates those having high likelihoods. Particle impoverishment is a side-effect where particle diversity is destroyed and all of these particles are likely to have low likelihoods, especially if the true state abnormally evolves. This paper proposes an adaptive scheme that relocates the particles when they are located far away from the maximum likelihood value to mitigate the particle impoverishment. The proposed scheme provides satisfactory effectiveness in discovering the abruptly changing hidden states and satisfactory estimation accuracy.
更多查看译文
关键词
Likelihood,particle filtering (PF),particle impoverishment,probability distribution function (PDF),state estimation
AI 理解论文
溯源树
样例
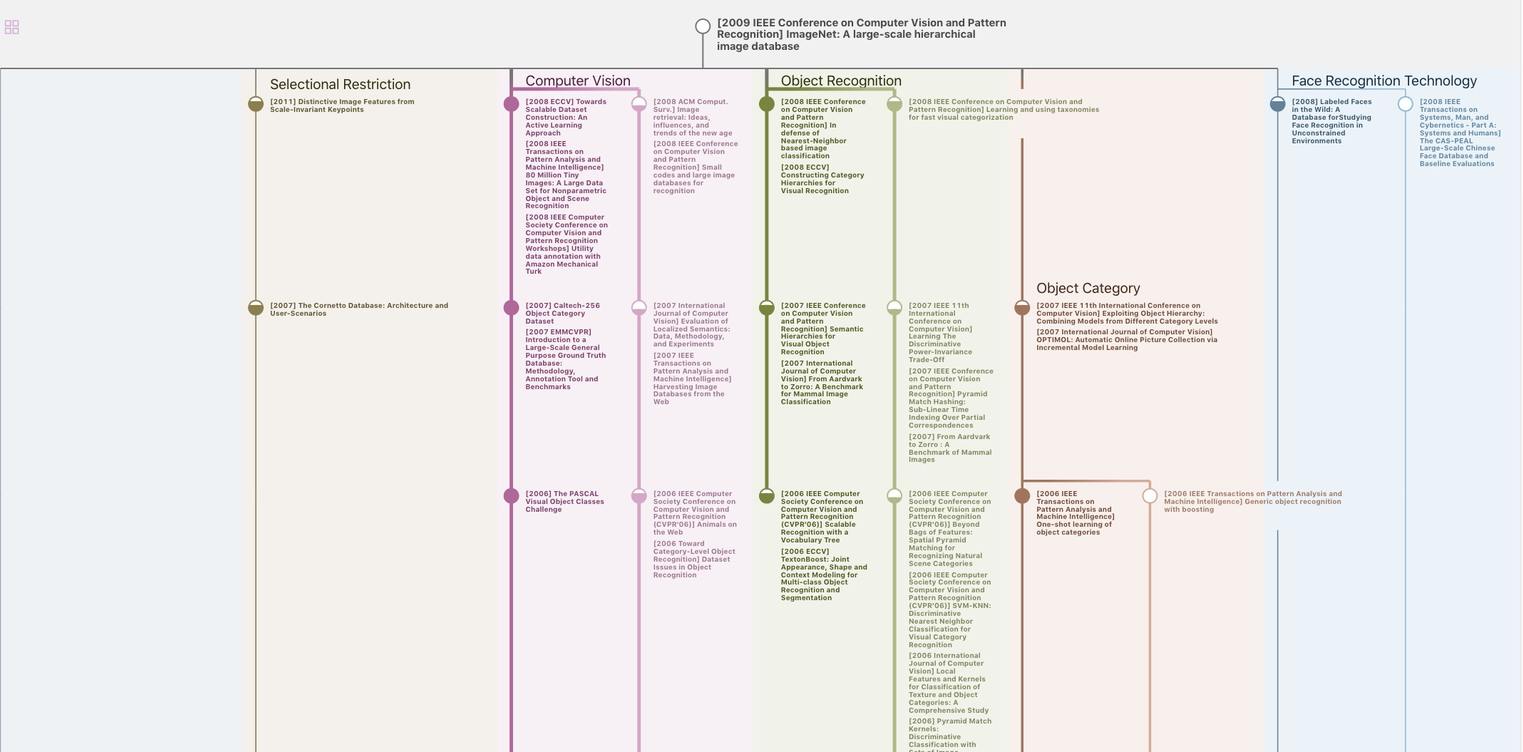
生成溯源树,研究论文发展脉络
Chat Paper
正在生成论文摘要