Monocular Object Detection & Localization on a 2D Plane Adapted to 360° Images without Retraining
2023 8th International Conference on Control and Robotics Engineering (ICCRE)(2023)
摘要
Equirectangular 360° images have the property of encompassing the omnidirectional field-of-vision in a single one-shot image, which have benefits and interesting use-cases as a form of perception for robots and autonomous vehicles. It is thus reasonable to implement object detection and localization on such images to enrich the perception of the surroundings of a given robot. Even though object detection models that were trained by deep learning have seen massive developments over the years, they do not adequately address the spherical semantics of an equirectangular image without special modification; a single image represents an observation (a color sphere with an assumed constant radius) in the form of a 2D image that does not semantically connect the side edges that are in fact the same in the real physical world. As a result, objects that lie on those vertical edges are not correctly detected. In this paper, we address this main problem by describing a methodology that adapts to any pre-trained object detection model without any retraining necessary. This is achieved by first applying the calibration parameters of the utilized camera to obtain a spherically corrected equirectangular image, then inferencing bounding box locations based on a batch of one image and its horizontally shifted version. Afterwards, we select the correct bounding boxes based on positional criteria. Additionally, we utilize calibration to correctly map between image pixel positions and real-world spherical coordinates. This allows us to utilize the spherical coordinates to create an image-to-world homography (assumption a flat-surface topology), thus achieving object localization.
更多查看译文
关键词
computer vision,equirectangular imagery,object detection,robot perception
AI 理解论文
溯源树
样例
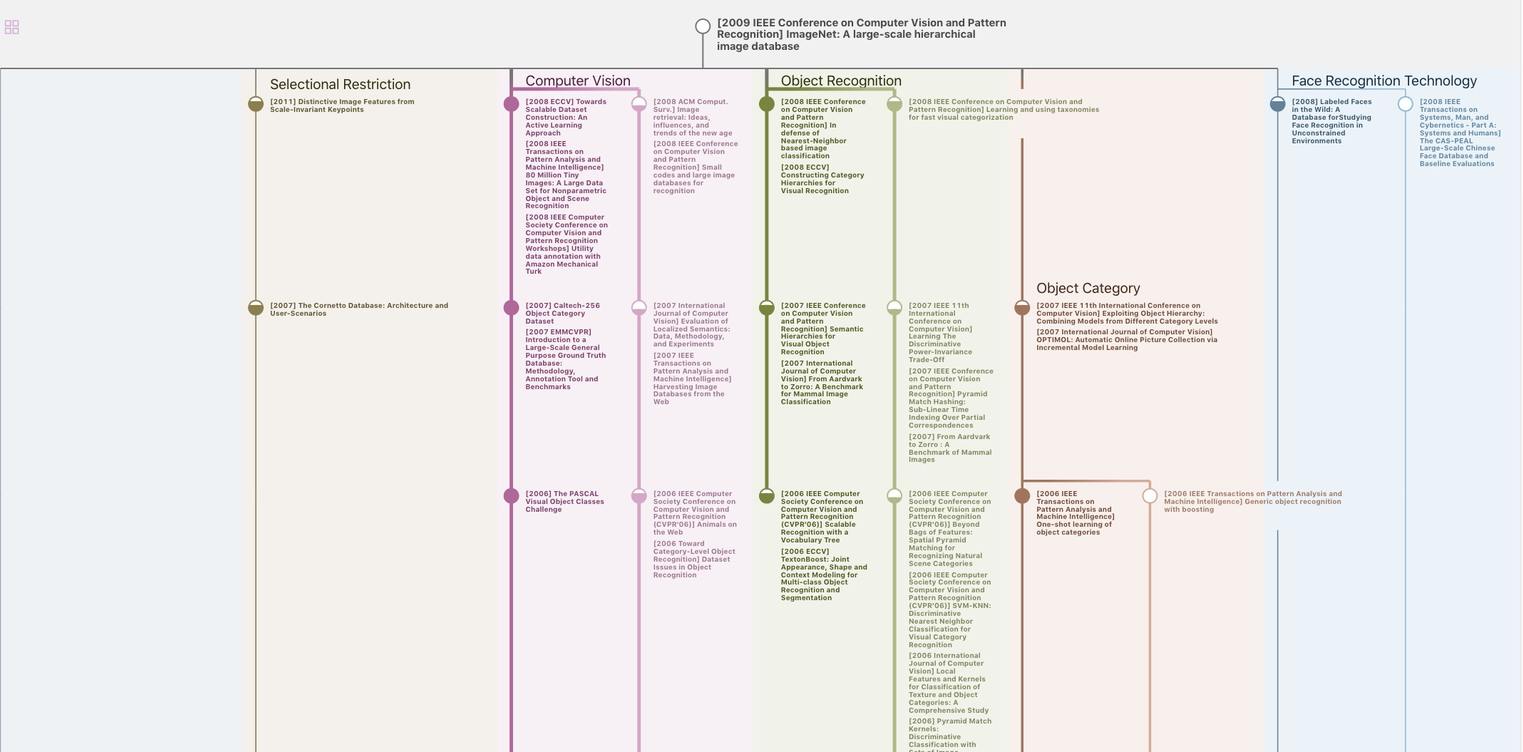
生成溯源树,研究论文发展脉络
Chat Paper
正在生成论文摘要