A Scalable Network Intrusion Detection System using Bi-LSTM and CNN
2023 Third International Conference on Artificial Intelligence and Smart Energy (ICAIS)(2023)
摘要
As cloud technologies are used more frequently, network intrusion detection systems are becoming increasingly well-liked. Due to ever-increasing network traffic and the regular emergence of new types of assaults, Network Intrusion Detection (NIDS) came into existence as a key aspect of network security and must be extremely effective. These kind of IDS systems employ either an anomaly detection system based on machine learning or a system for matching patterns. The False Positive Rate for pattern matching approaches is high, but AI/ML-based systems determine the possibility of an attack by identifying a metric or characteristic or a connection between a number of metrics or characteristics. The most popular models include KNN, SVM, and others, they only work on a small range of traits, are not very accurate, and have a high False Positive Rate. This study created a deep learning system to learn the temporal and spatial data properties using the advantages of CNN and Bidirectional LSTM. The system present in this paper is trained and analyzed using the openly available dataset NSL-KDD. The proposed model has a high rate of detection and a low incidence of false positives. A lot of cutting-edge Network Intrusion Detection systems that use Machine Learning/Deep Learning models perform better than the suggested model.
更多查看译文
关键词
Network Intrusion,Cyber Attacks,Classification,Decision Trees,Intrusion Detection System (IDS),Deep Learning,Recurrent Neural Networks (RNN),Convolutional Neural Networks (CNN),Bi-Directional LSTM (Bi-LSTM),Ensemble Models
AI 理解论文
溯源树
样例
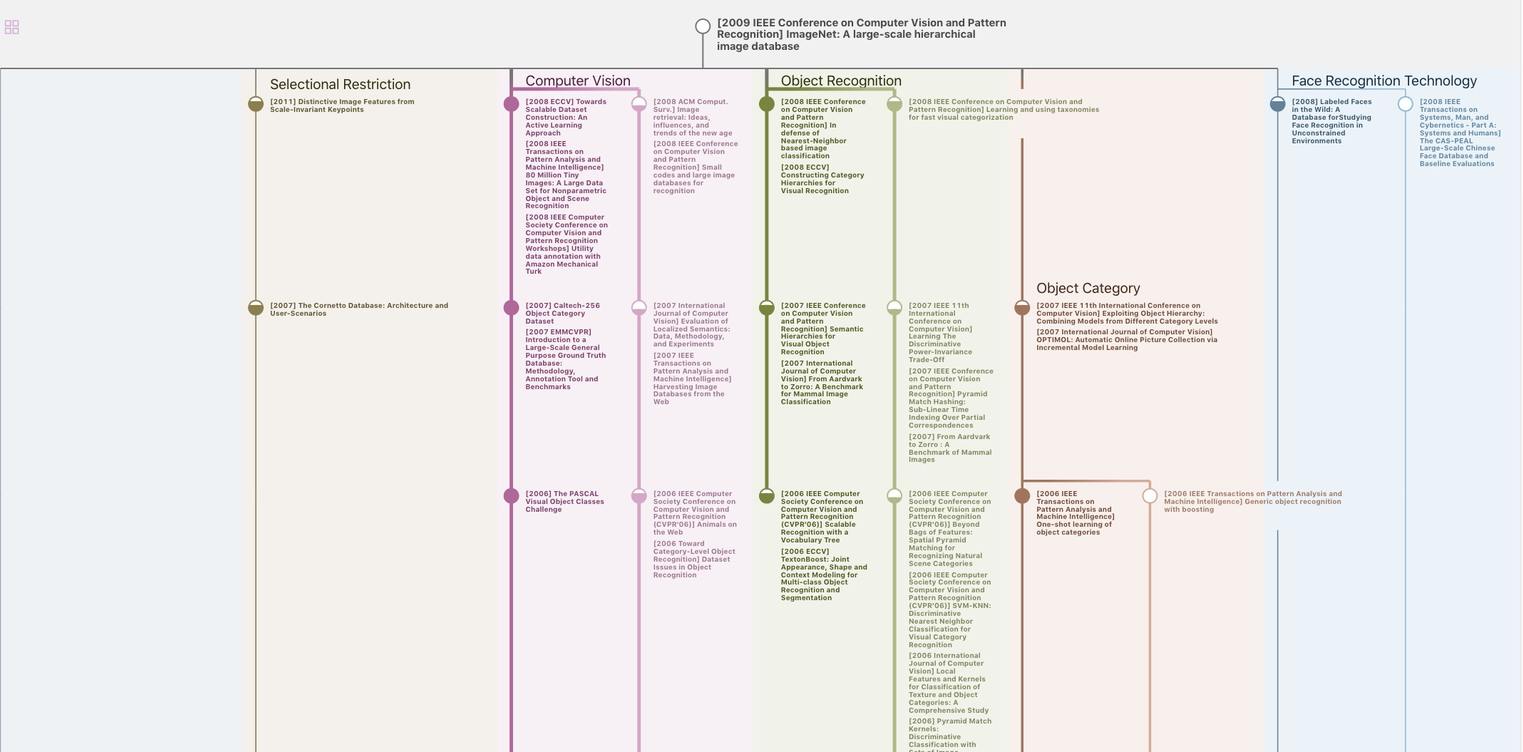
生成溯源树,研究论文发展脉络
Chat Paper
正在生成论文摘要