Fast Oscillation Detection and Labeling via Coarse Grained Time Series Data for ML Applications
2022 IEEE Power & Energy Society Innovative Smart Grid Technologies Conference (ISGT)(2022)
摘要
Oscillation detectors use an RMS signal’s energy in pre-specified frequency bands to detect the presence of different kinds of oscillations. While originally intended for situational awareness applications, they can be used for labeling the periods were they appear in large historical data sets. Labeled data sets are a requirement in machine learning applications. One such application is that of deriving associations between system operating conditions and the appearance of certain oscillations. However, there are two main challenges when realizing such application in practice. Firstly, a reliable detector requires properly set thresholds for the energy values. Secondly, scaling the algorithm to label multi-year historical data archives containing hundreds of terabytes of signals requires fast data access. The PingThings PredictiveGrid time series data platform deployed at Dominion Energy stores statistical averages of synchrophasor data at increasingly coarse resolutions as well as the original measurements. This work explores the use of coarse as opposed to full resolution PMU data for fast oscillation detection. Furthermore, K-means clustering is used to automatically determine energy thresholds based on the energy distribution of historical data in relevant frequency bands. Results using synchrophasor data from a STATCOM in the Dominion system expose a local mode.
更多查看译文
关键词
Machine learning,oscillation detection,syn-chrophasors,spectral analysis
AI 理解论文
溯源树
样例
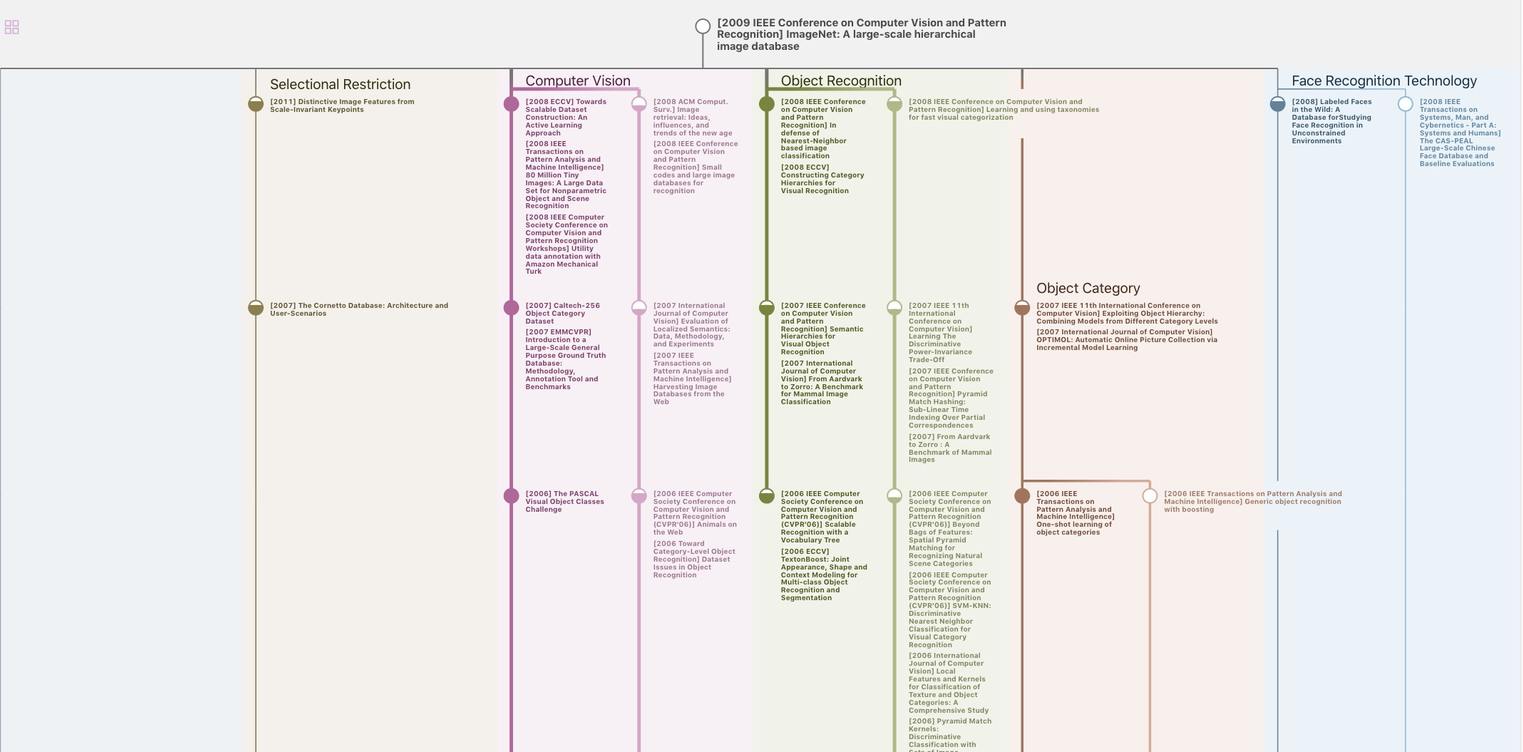
生成溯源树,研究论文发展脉络
Chat Paper
正在生成论文摘要