The Composite Technique for Predicting Traffic Flow Based on Missing Traffic Information: A Review
2022 4th International Conference on Advances in Computing, Communication Control and Networking (ICAC3N)(2022)
摘要
Due to the large number of traffic sensors that may be used to gather traffic data, lots of work has done in the development of traffic prediction algorithms for improving route navigation, urban planning and traffic management, among other things. One of the most challenging characteristics of traffic forecasting is determining and how much to be dependent on models built from previous data in actual traffic situations, which may vary from past data and change over time. This study presents a new accessible structure that can acquire from real-time traffic situation (or contexts) and forecast future traffic by comparing the current situation to the best effective prediction model developed from prior data. The traffic environment space is adaptive adjustable partitioned as real-time traffic enters the system, allowing each base predictor's performance to be evaluated effectively under diverse scenarios. As a result of our online technique, both long-term and short-term performance guarantees are generated and demonstrated in this study. When authentic labels are not available or delayed, the proposed approach remains effective. It is also feasible to identify which component of the traffic prediction scenario is the most significant, which can help reduce implementation complexity while simultaneously providing relevant information to traffic policymakers. The suggested strategy outperforms existing solutions on real-world data in real-world circumstances, according to our research. Using correlated and collinear traffic data, the proposed model can accurately and effectively predict short-term traffic conditions. Mainly three type of case studies created for demonstrate the model’s use in short-term traffic forecasting applications
更多查看译文
关键词
Long and short term performance,Genetic algorithm,Support vector regression
AI 理解论文
溯源树
样例
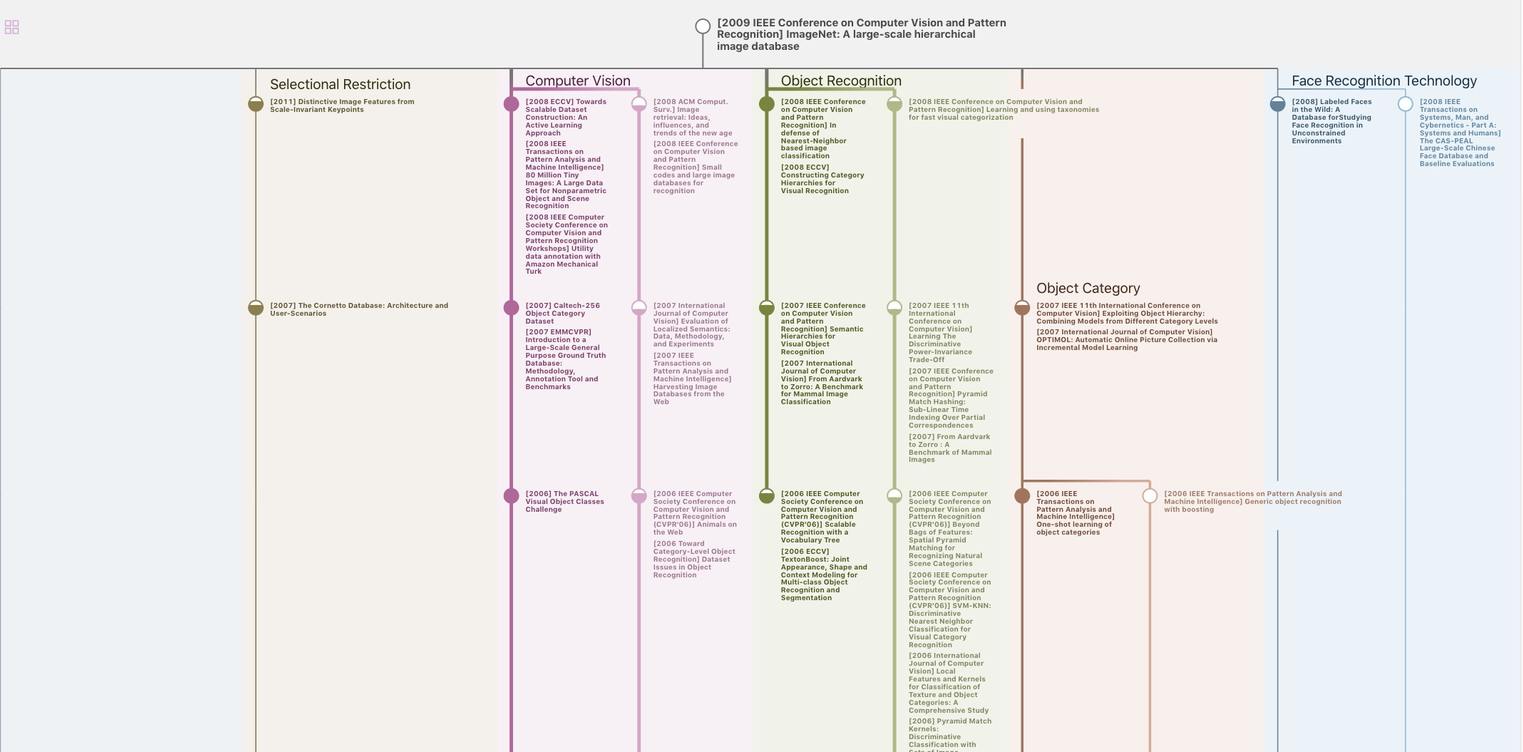
生成溯源树,研究论文发展脉络
Chat Paper
正在生成论文摘要