Global Multi-Object Transformer Tracking with Detecting and Attention Mechanism
2023 IEEE 13th International Conference on CYBER Technology in Automation, Control, and Intelligent Systems (CYBER)(2023)
摘要
Visual multiple-object tracking (MOT) has always been a research hotspot in the fields of computer vision and video image intelligence analysis. Tracking algorithms must not only accurately locate targets but also adapt to various types of interference while meeting robustness requirements. However, the uncertainty of the number of objects in the scene, mutual occlusion between objects, and the low discriminability of object features have led to slow progress in the practical application of visual multiple-object tracking. To address this issue, we designed a modified attention mechanism module and embedded it into a transformer-based global multiple-object tracking architecture, generating global trajectories for all objects. This forms the proposed efficient algorithm for object tracking. For larger convolution kernels, our method effectively extracts each feature in the receptive field while reducing computation costs, thus improving algorithm efficiency. We conducted the experiments on MOT17 and TAO, and achieved the state-of-the-art results. Experimental results show that our proposed method outperforms existing models overall, ensuring stable object trajectories while maintaining high-quality detection.
更多查看译文
关键词
computer vision,global multiobject transformer tracking,modified attention mechanism module,object features,stable object trajectories,tracking algorithms,transformer-based global multiple-object tracking architecture,video image intelligence analysis,visual multiple-object tracking
AI 理解论文
溯源树
样例
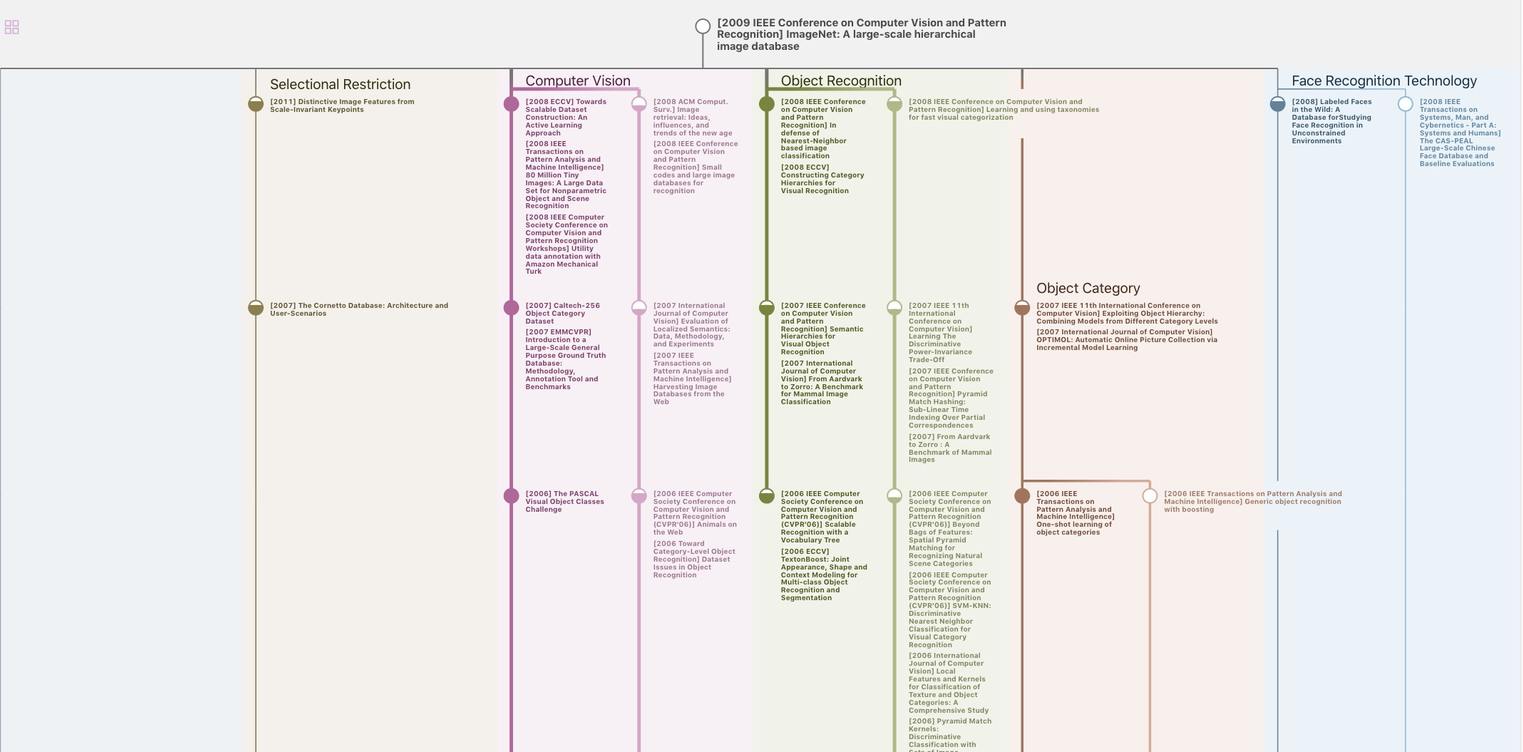
生成溯源树,研究论文发展脉络
Chat Paper
正在生成论文摘要