An Optimized Intrusion Detection System for Cyber-Physical System Attack Using Long Short-Term Memory
2023 IEEE 13th International Conference on CYBER Technology in Automation, Control, and Intelligent Systems (CYBER)(2023)
摘要
With the continuous development of deep learning, Long Short-Term Memory (LSTM) is constantly evolving. This paper presents an optimized method for building an Intrusion Detection System (IDS) for a Cyber-Physical System (CPS) using deep learning techniques. Specifically, the LSTM architecture is applied to a Recurrent Neural Network (RNN), which is trained on the Bot-IoT Dataset. This paper addresses the limitations of traditional RNNs by introducing LSTM, which effectively alleviates the problem of gradient disappearance and allows longer sequences to be used for training. The proposed method acquires high-level abstractions in data through complex architectures or combinations of nonlinear transformations, leading to high detection rates. The theoretical analysis provides insights into the algorithm's performance in terms of its computational complexity, convergence rate, and accuracy, among other important aspects. Through experiments, the optimal parameters of LSTM are determined, and the convergence speed of the neural network is optimized. This work demonstrates the potential of deep learning in IDS and facilitates the development of multi-agent systems by enhancing the agents' ability to process sequential data and temporal information.
更多查看译文
关键词
Bot-IoT Dataset,computational complexity,convergence rate,convergence speed,CPS,cyber-physical system attack,deep learning technique,gradient disappearance,high-level abstraction,IDS,long short-term memory,LSTM architecture,multiagent systems,nonlinear transformation,optimized intrusion detection system,recurrent neural network,RNN,sequential data processing,temporal information
AI 理解论文
溯源树
样例
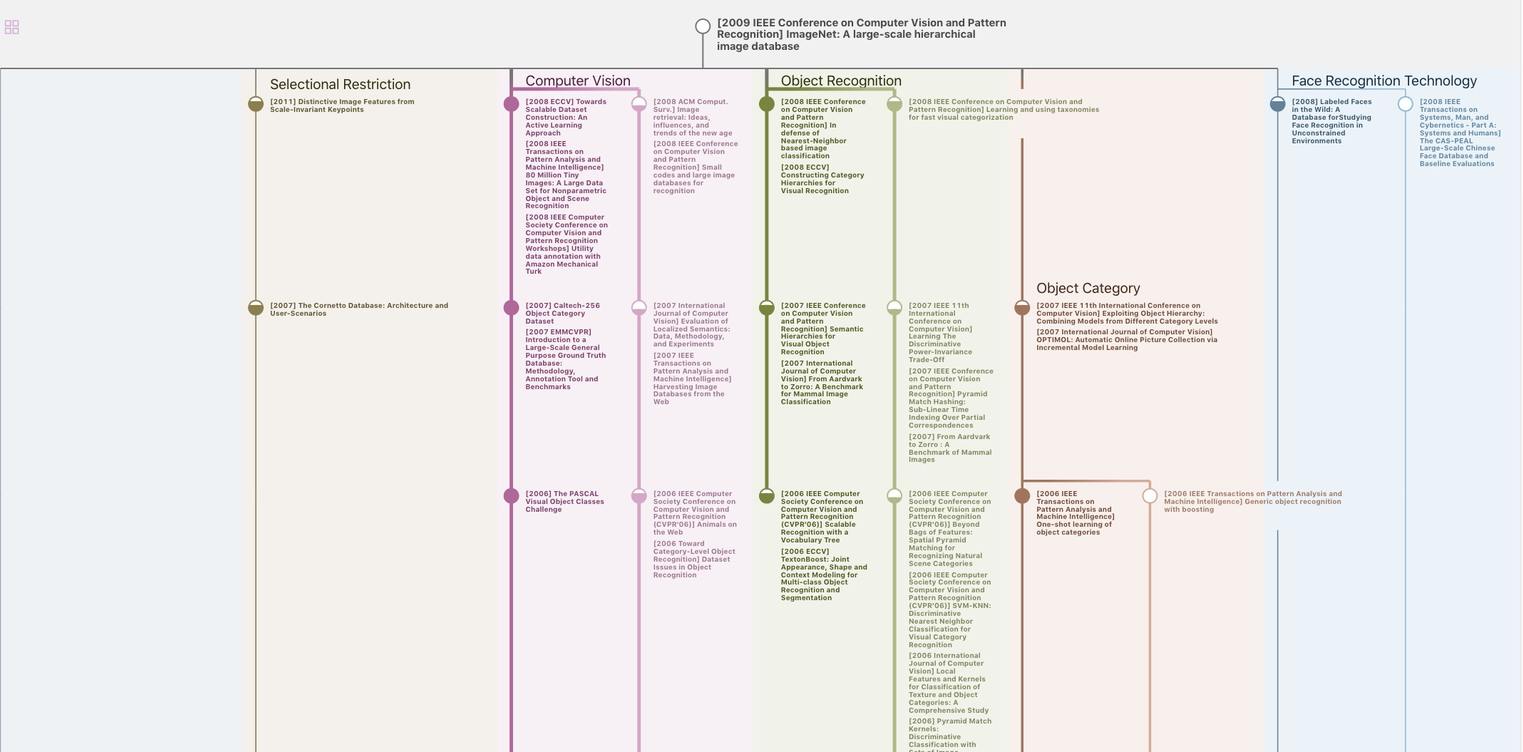
生成溯源树,研究论文发展脉络
Chat Paper
正在生成论文摘要