Battery Health Prognosis: Discharging Capacity Prediction at All Operating Voltage Levels
2023 IEEE Power & Energy Society General Meeting (PESGM)(2023)
摘要
The battery energy storage system is an essential component in the modern energy system with the development of renewable energy, transportation electrification, and carbon-neutral goals. Battery degradation has been the most challenging issue of energy storage. This work presents a data-driven battery degradation model powered by long short-term memory (LSTM) recurrent neural network (RNN). Utilizing the battery dataset with more than 100 batteries exposed to different operations, the proposed model gives a precise prediction of full-discharge capacity and internal resistance (IR) with the root-mean-square error (RMSE) of 0.008 Ah and 0.00017 Ohm in 100 cycles, respectively. Instead of a single capacity or state of health (SOH) value projection, our model predicts the full-discharge capacity-voltage trajectory of the following cycles, addresses the capacity and energy content in different voltage ranges, and improves the accuracy and applicability of the SOH prognosis in industrial applications.
更多查看译文
关键词
state of health, data -driven prognosis, battery degradation modeling, battery health indicator
AI 理解论文
溯源树
样例
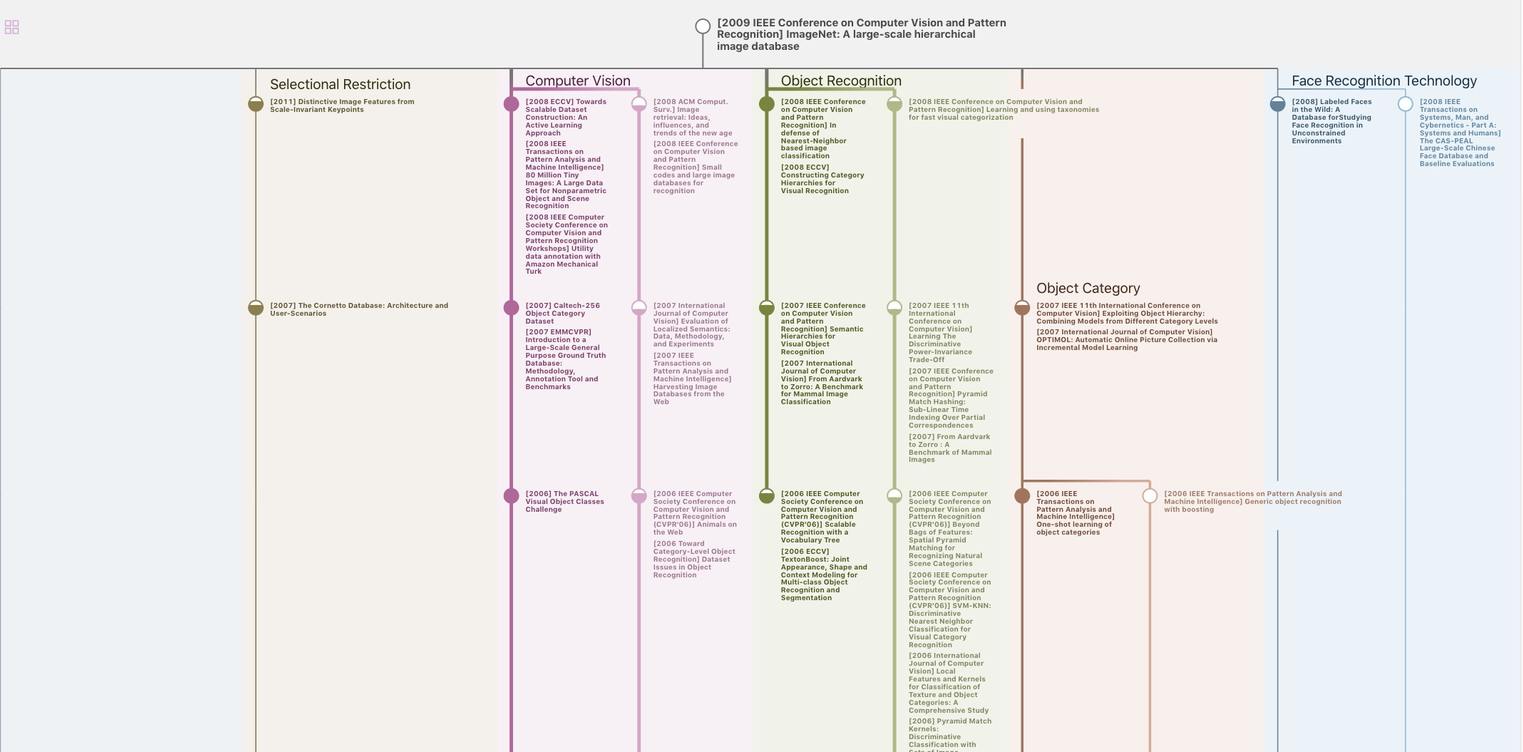
生成溯源树,研究论文发展脉络
Chat Paper
正在生成论文摘要