Machine Learning Application to Extreme Weather Power Outage Forecasting in Distribution Networks using a Majority Under-Sampling and Minority Over-Sampling Strategy
2023 IEEE Power & Energy Society General Meeting (PESGM)(2023)
摘要
In the race against climate change as the frequency and severity of extreme weather events increases power system contingency analysis becomes increasingly critical. Power system operators can leverage Machine Learning (ML) in emergency response planning and operation to minimize the risks associated with power outages caused by extreme weather events. This paper presents a Machine Learning (ML) based classifier based on logistic regression theory to predict power distribution grid outages caused by an ice storm. The proposed Majority Under-Sampling and Minority Over-sampling (MUMO) addresses the frequency bias commonly present in power system outage data and improves the outage prediction model performance through Minimum Redundancy Maximum Relevance (MRMR) feature engineering and cross-validation.
更多查看译文
关键词
Machine Learning,Ice Storm,Power Distribution Grid Resilience,Majority Under-Sampling and Minority Over-Sampling,Minimum Redundancy Maximum Relevance
AI 理解论文
溯源树
样例
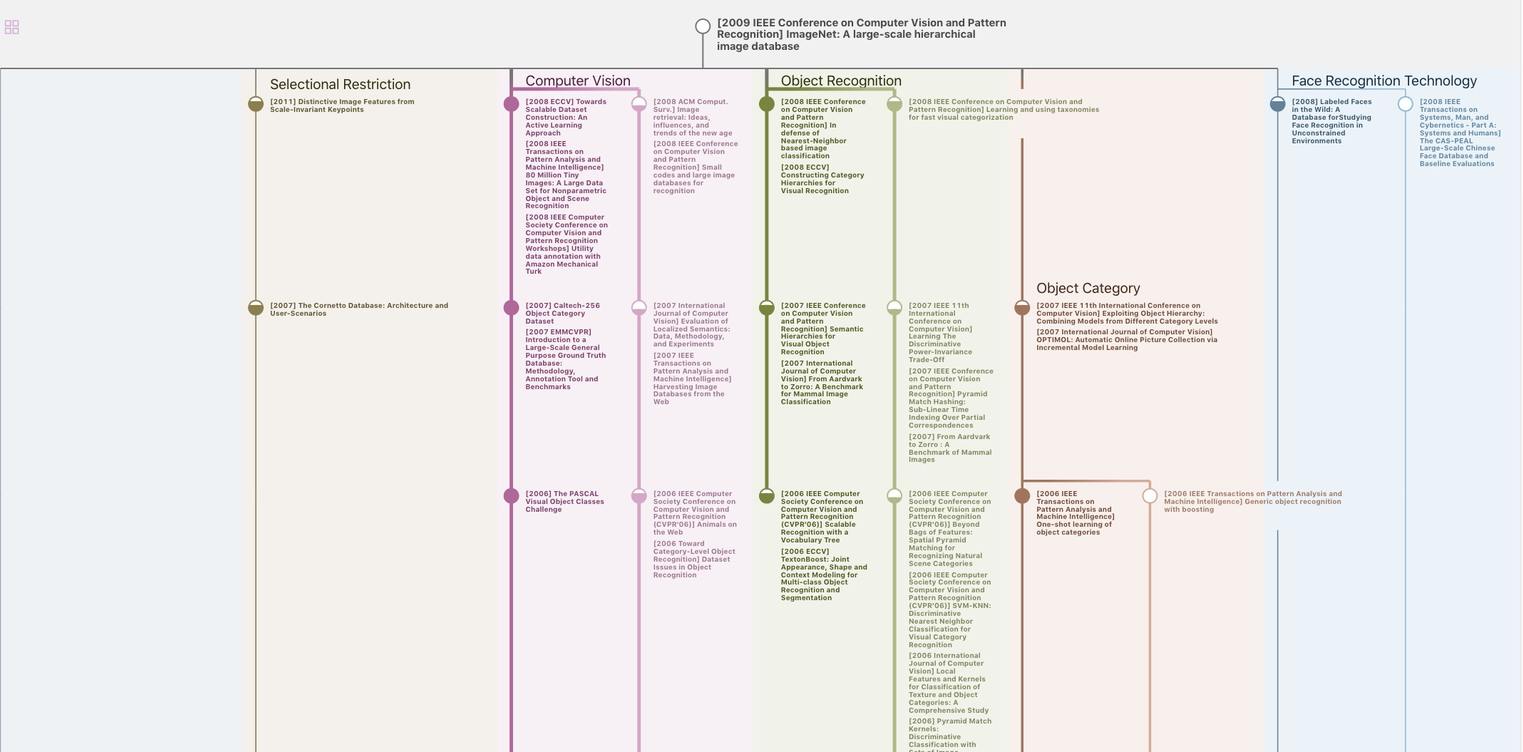
生成溯源树,研究论文发展脉络
Chat Paper
正在生成论文摘要