Splinets -- Orthogonal Splines and FDA for the Classification Problem
arxiv(2023)
摘要
This study introduces an efficient workflow for functional data analysis in classification problems, utilizing advanced orthogonal spline bases. The methodology is based on the flexible Splinets package, featuring a novel spline representation designed for enhanced data efficiency. Several innovative features contribute to this efficiency: 1)Utilization of Orthonormal Spline Bases 2)Consideration of Spline Support Sets 3)Data-Driven Knot Selection. Illustrating this approach, we applied the workflow to the Fashion MINST dataset. We demonstrate the classification process and highlight significant efficiency gains. Particularly noteworthy are the improvements that can be achieved through the 2D generalization of our methodology, especially in scenarios where data sparsity and dimension reduction are critical factors. A key advantage of our workflow is the projection operation into the space of splines with arbitrarily chosen knots, allowing for versatile functional data analysis associated with classification problems. Moreover, the study explores Splinets package features suited for functional data analysis. The algebra and calculus of splines use Taylor expansions at the knots within the support sets. Various orthonormalization techniques for B-splines are implemented, including the highly recommended dyadic method, which leads to the creation of splinets. Importantly, the locality of B-splines concerning support sets is preserved in the corresponding splinet. Using this locality, along with implemented algorithms, provides a powerful computational tool for functional data analysis.
更多查看译文
AI 理解论文
溯源树
样例
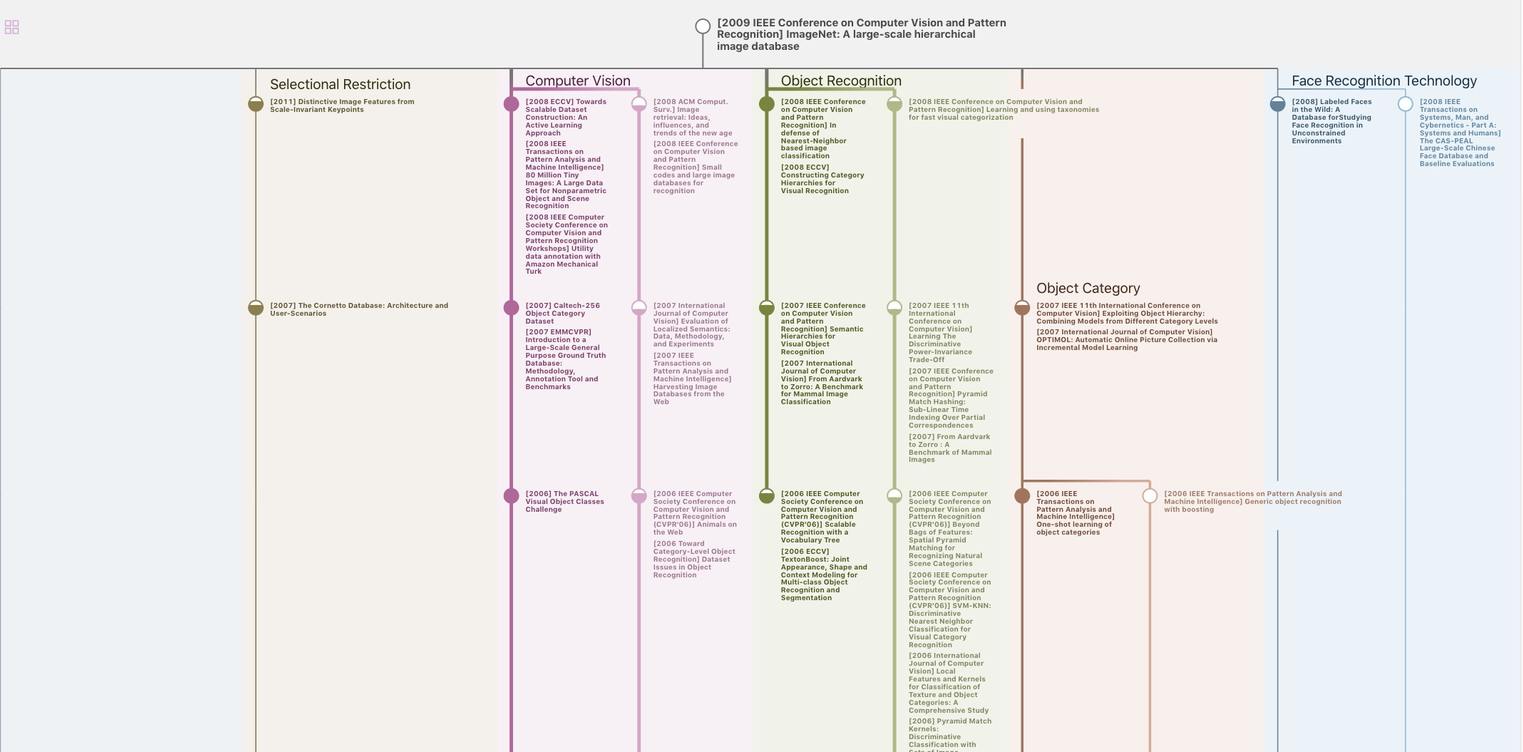
生成溯源树,研究论文发展脉络
Chat Paper
正在生成论文摘要