Application of explainable artificial intelligence to analyze basic features of a tender
2023 3rd International Conference on Electrical, Computer, Communications and Mechatronics Engineering (ICECCME)(2023)
摘要
This work consists of predicting tenders based on machine learning techniques using classification algorithms such as Decision Tree, Logistic Regression, Random Forest, and explainability techniques LIME and SHAP. The objective is to improve transparency and efficiency in the supplier selection process for public contracts. A comparison is made between the classification algorithms to determine which performs best, resulting in the Random Forest model. To improve the model’s explainability, LIME and SHAP techniques are used, which allow visualizing and understanding the impact of each variable on the model’s decision. The proposed analysis can be a valuable tool for SMEs (Small and medium-sized enterprises) looking to participate in public procurement more efficiently. In conclusion, this project demonstrated that the LIME technique allows for data interpretation in a simpler and more understandable way with the Random Forest classification model.
更多查看译文
关键词
explainable artificial intelligence,machine learning,tender,Random Forest,LIME,SHAP
AI 理解论文
溯源树
样例
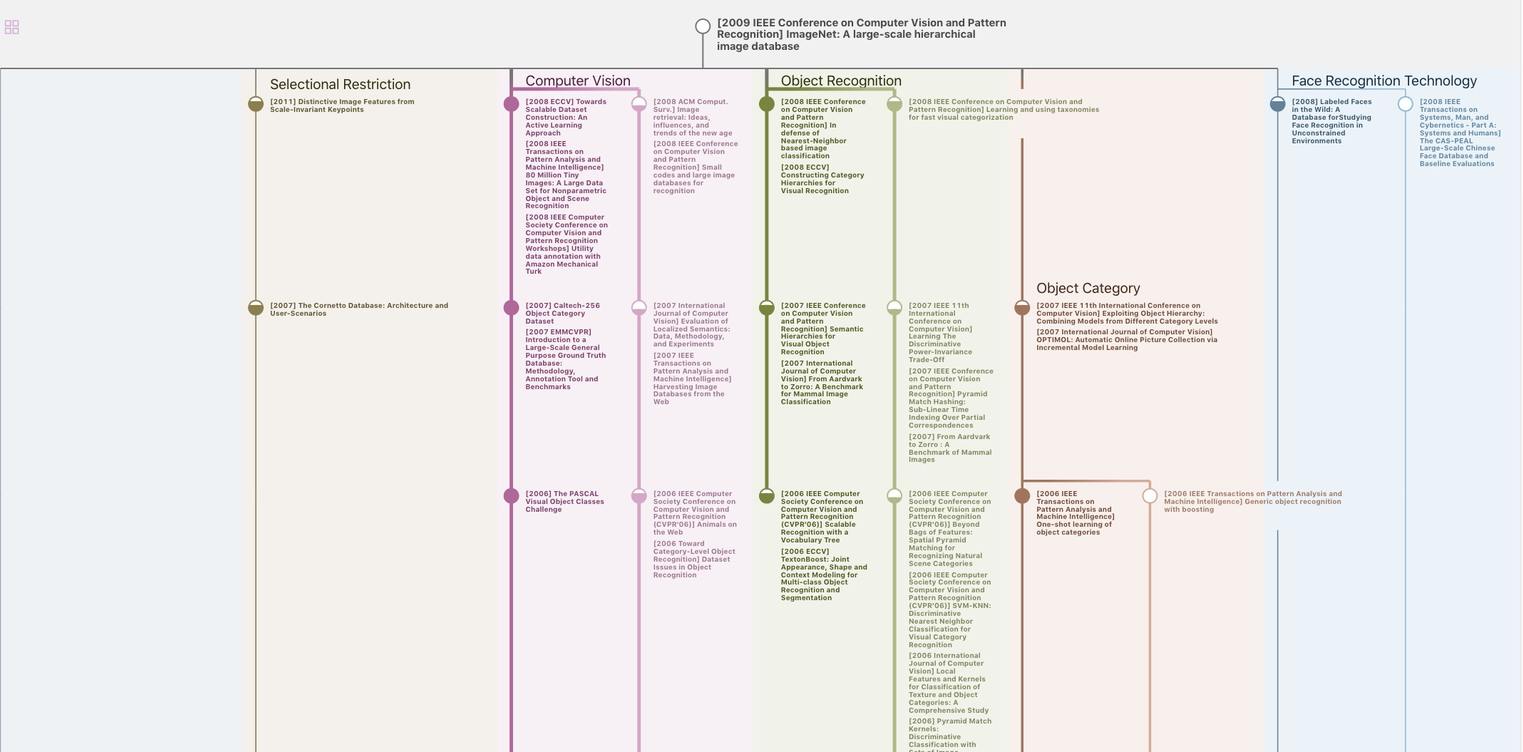
生成溯源树,研究论文发展脉络
Chat Paper
正在生成论文摘要