ICCVAE: Item Concept Causal Variational Auto-Encoder for top-n recommendation
2023 8th International Conference on Intelligent Computing and Signal Processing (ICSP)(2023)
摘要
Recently, recommendation systems, especially interpretable ones, have become increasingly popular. The recommendation system provides personalized recommendations to users based on their previous behaviour data. Existing approaches often transfer methods in disentangle learning, especially Variational Auto-Encoder (VAE) framework, into the recommendation system. However, VAE is proved sub-optimal due to the independence factor assumption. Unfortunately, few research focuses on the VAE framework without an independent assumption for recommendation system. To escape from sub-optimality from independence assumption and provide more interpretability, in this paper, we propose a new method for top-n recommendation tasks called Item Concept Causal Variational Auto-Encoder (ICCVAE), enabling to build up causal structure for factors, i.e., item concepts in recommendation system. We conduct experiments to prove superiority for ICCVAE. Our framework can reach up to 5.3%, 3.4%, and 6.1% definite improvement over baselines in terms of Recall@20, Recall@50 and NDCG@100.
更多查看译文
关键词
recommendation system,Variational Auto-Encoder,interpretability
AI 理解论文
溯源树
样例
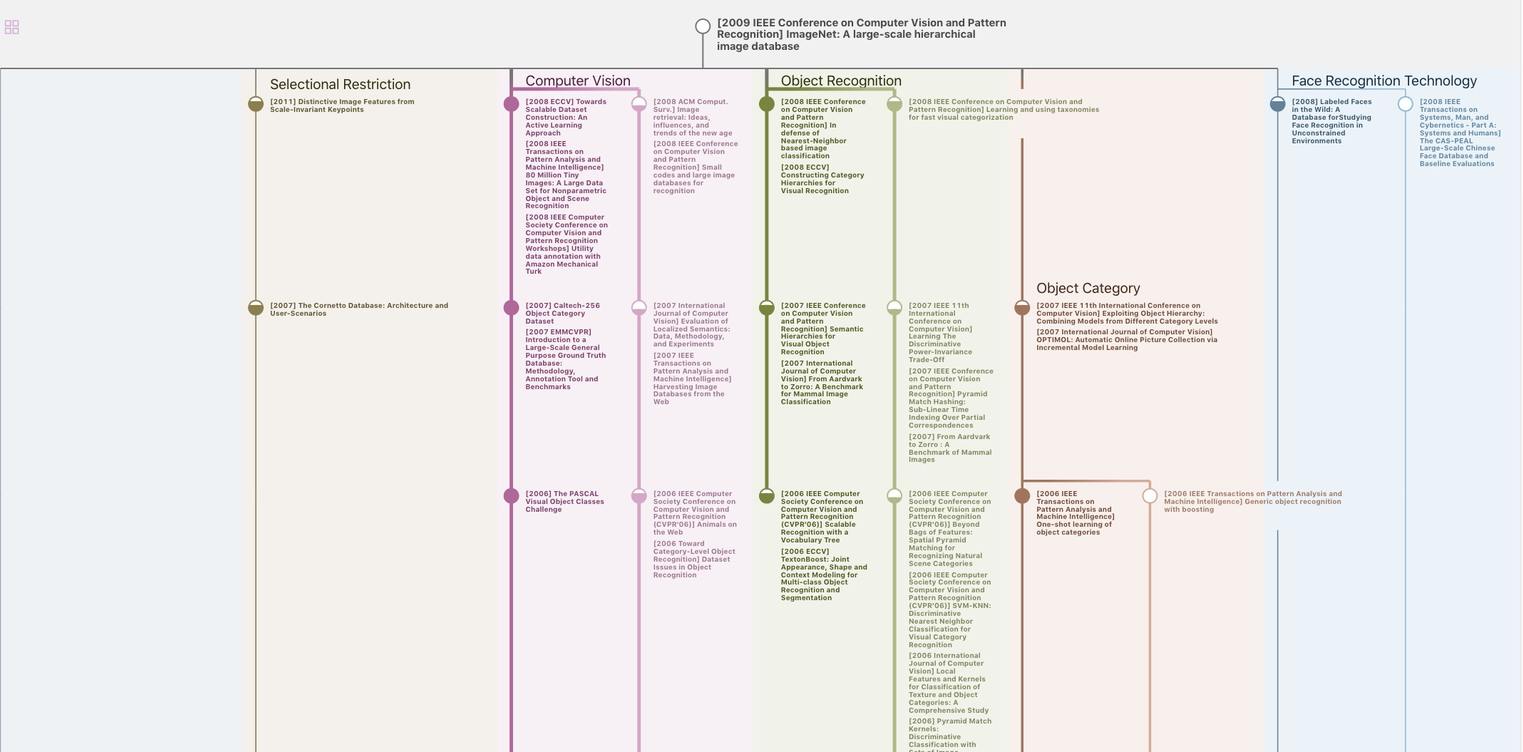
生成溯源树,研究论文发展脉络
Chat Paper
正在生成论文摘要