Late Breaking Results: Reinforcement Learning for Scalable Logic Optimization with Graph Neural Networks
2021 58th ACM/IEEE Design Automation Conference (DAC)(2021)
摘要
Logic optimization is an NP-hard problem commonly approached through hand-engineered heuristics. We propose to combine graph convolutional networks with reinforcement learning and a novel, scalable node embedding method to learn which local transforms should be applied to the logic graph. We show that this method achieves a similar size reduction as ABC on smaller circuits and outperforms it by $1. 5 - 1. 75 \times $ on larger random graphs.
更多查看译文
关键词
local transforms,larger random graphs,reinforcement learning,scalable logic optimization,graph neural networks,NP-hard problem,hand-engineered heuristics,graph convolutional networks,scalable node embedding method
AI 理解论文
溯源树
样例
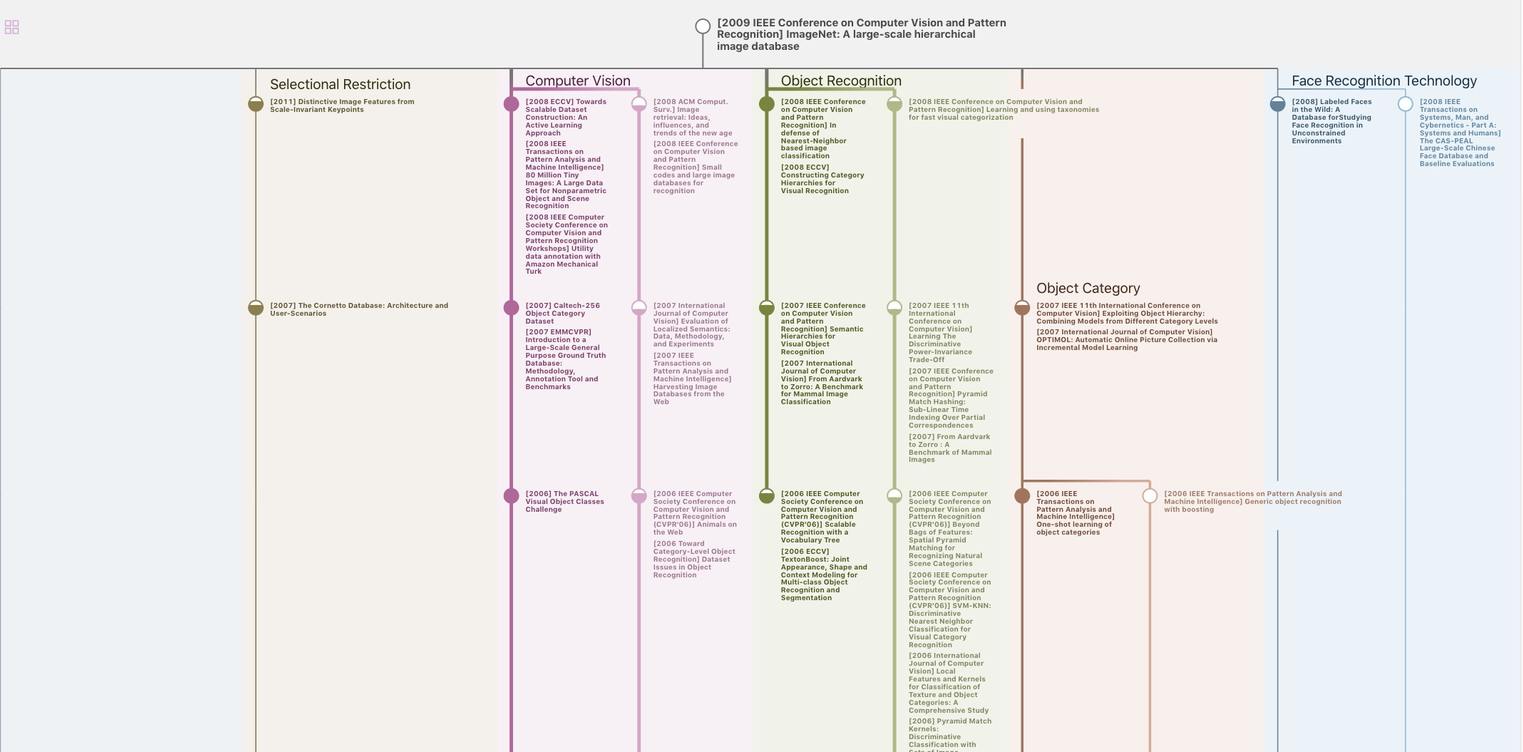
生成溯源树,研究论文发展脉络
Chat Paper
正在生成论文摘要