Self-Noise Cancellation in Underwater Acoustics using Deep Neural Network Frameworks
OCEANS 2023 - Limerick(2023)
摘要
The direction-of-arrival (DOA) estimation is a challenging task for towed array sonars in the presence of self-noise. Hence, self-noise cancellation (SNC) is necessary for correct detection and DOA estimation of the targets. Deep learning techniques, with their high-feature extraction capability and self-learning ability have been used for de-noising in image and audio processing, but have not been attempted in the underwater acoustics for SNC. We have therefore proposed SNC using autoencoders and VGG-16 based encoder-decoder (VGG-ED), trained in both supervised and semi-supervised manner. With the knowledge of the clean signal (without self-noise) and actual target DOAs, the autoencoder learns in a supervised manner to estimate the clean signal from the noisy signal. Since the clean signal is unavailable in reality, we also propose to use a semi-supervised learning approach. Herein, the autoencoder is trained with the estimated clean signal produced by the null space projection technique using self-noise and corresponding signal-to-interference-noise-ratio (SINR). The proposed autoencoder can reduce self-noise by 51 dB when SINR is −32 dB with a fewer sensors and snapshots.
更多查看译文
关键词
Self-noise cancellation, deep learning, convolutional neural networks, sensor array
AI 理解论文
溯源树
样例
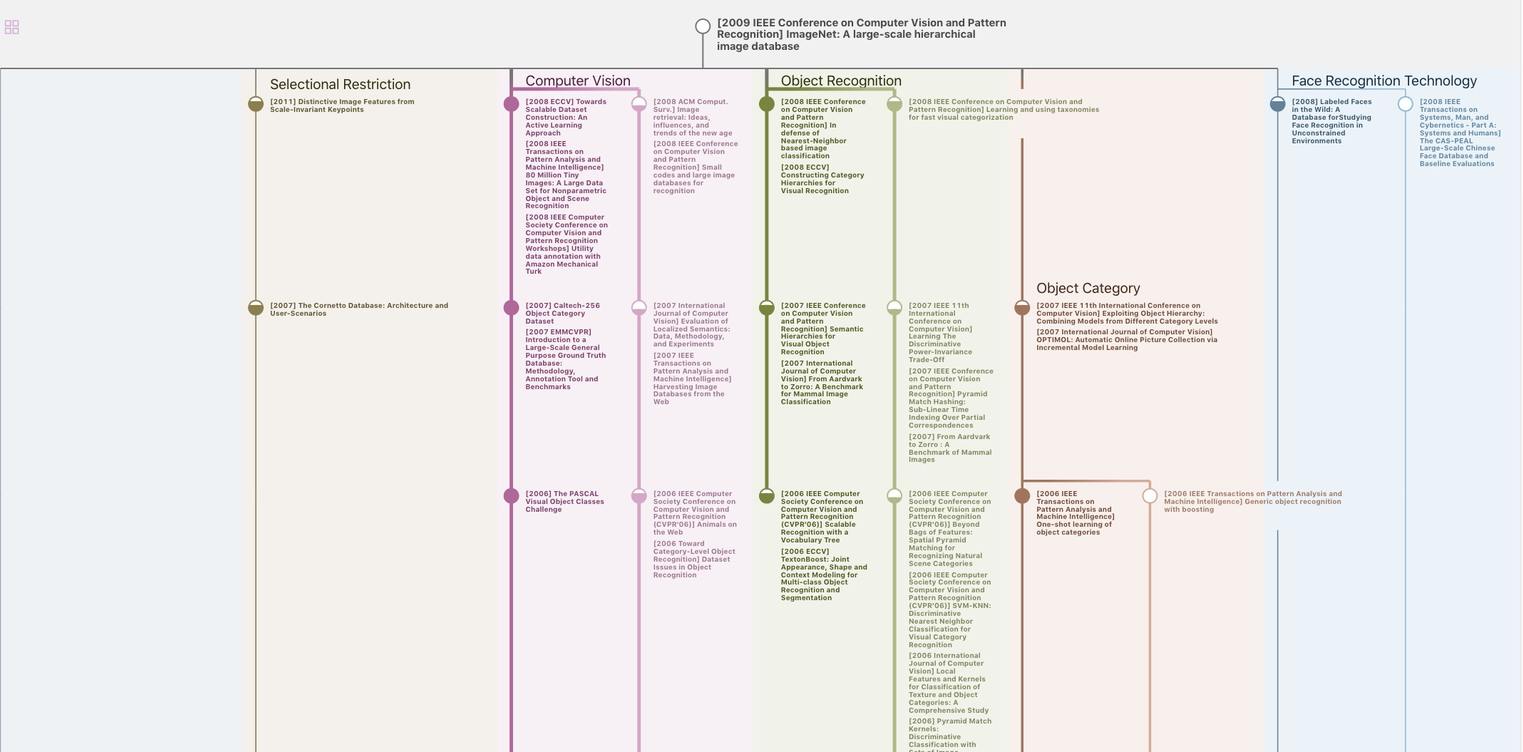
生成溯源树,研究论文发展脉络
Chat Paper
正在生成论文摘要