Comparison of seabed classification using unsupervised and supervised learning on ship noise spectrograms
OCEANS 2023 - Limerick(2023)
摘要
Applications of supervised machine learning to ocean acoustics is often limited by the lack of labeled measured data. To overcome this, synthetic data can be used for training. This paper explores the potential for unsupervised learning to provide labels for measured data. Specifically, a comparison is made between seabed classification from supervised learning and labels inferred from unsupervised learning. Both networks are trained on synthetic ship noise spectrograms. Six CNN-based supervised learning methods were trained using synthetic data labeled by seabed class. The trained networks were applied to 69 measured spectrograms from the Seabed Characterization Experiment 2017. The results show a distinct preference for seabeds with softer top layer (water-sediment sound speed ratios less than one). The unsupervised ML method, k-means clustering, is applied to same synthetic dataset, and the resulting clusters are evaluated based on the characteristics of the synthetic data samples placed into each cluster. The measured ship noise spectrograms are then passed through the trained clustering model, and the characteristics of the assigned clusters are evaluated. Of the 69 measured data samples, 70% are placed in clusters showing a distinct preference for seabed classes similar to those obtained from the CNN-based classifiers. Other measured data samples are placed in clusters that contain synthetic data samples from short ranges. This work illustrates the potential for using clustering to assign preliminary labels to unlabeled data.
更多查看译文
关键词
machine learning, unsupervised learning, ocean acoustics, ship noise, spectrograms, k-means clustering, seabed classification
AI 理解论文
溯源树
样例
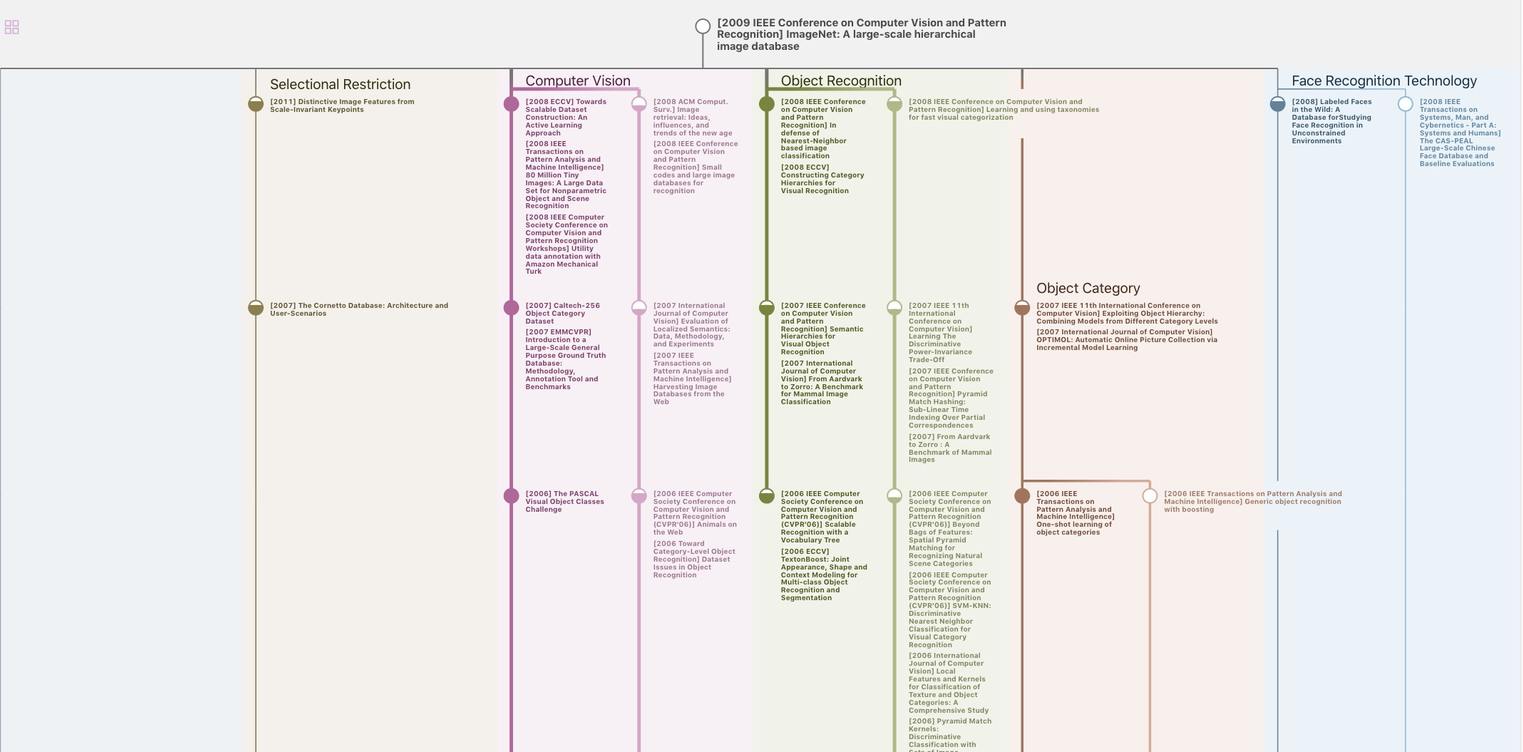
生成溯源树,研究论文发展脉络
Chat Paper
正在生成论文摘要