SE-yolov5 inland waterway vessel target recognition based on CGAN image enhancement
2023 7th International Conference on Transportation Information and Safety (ICTIS)(2023)
摘要
With the continuous development of deep learning technology, ship target recognition based on video images has emerged as a viable approach for water supervision. However, traditional self-built datasets suffer from insufficient feature sampling and large target recognition errors in ship target detection. In order to enhance the accuracy of ship target detection in complex environments, this paper proposes an improved yolov5 ship target recognition method based on CGAN image enhancement. The method leverages CGAN network to augment the original dataset, thereby completing data enhancement. Furthermore, the SE (Squeeze-and-Excitation) attention mechanism module is integrated into the yolov5 network to train and evaluate the ship image dataset. Experimental results demonstrate that the CGAN network augmentation image outperforms the GAN basic network augmentation image in the dataset, generating type condition constraints. The accuracy of the SE-YOLOV5 network for bulk carriers, container ships, official ships, and beacons is 70%, 97%, 90%, and 60% respectively, showcasing improvements compared to the basic yolov5 network and Faster-RCNN network. The findings indicate that the improved yolov5 ship target recognition method, incorporating CGAN image enhancement, effectively addresses the challenge of low target detection accuracy in multi-target and inland river ships with insufficient lighting conditions under small data sets. It significantly enhances the overall performance of ship detection and recognition.
更多查看译文
关键词
Ship target detection,CGAN,SE-YOLOV5,Feature extraction,Deep learning
AI 理解论文
溯源树
样例
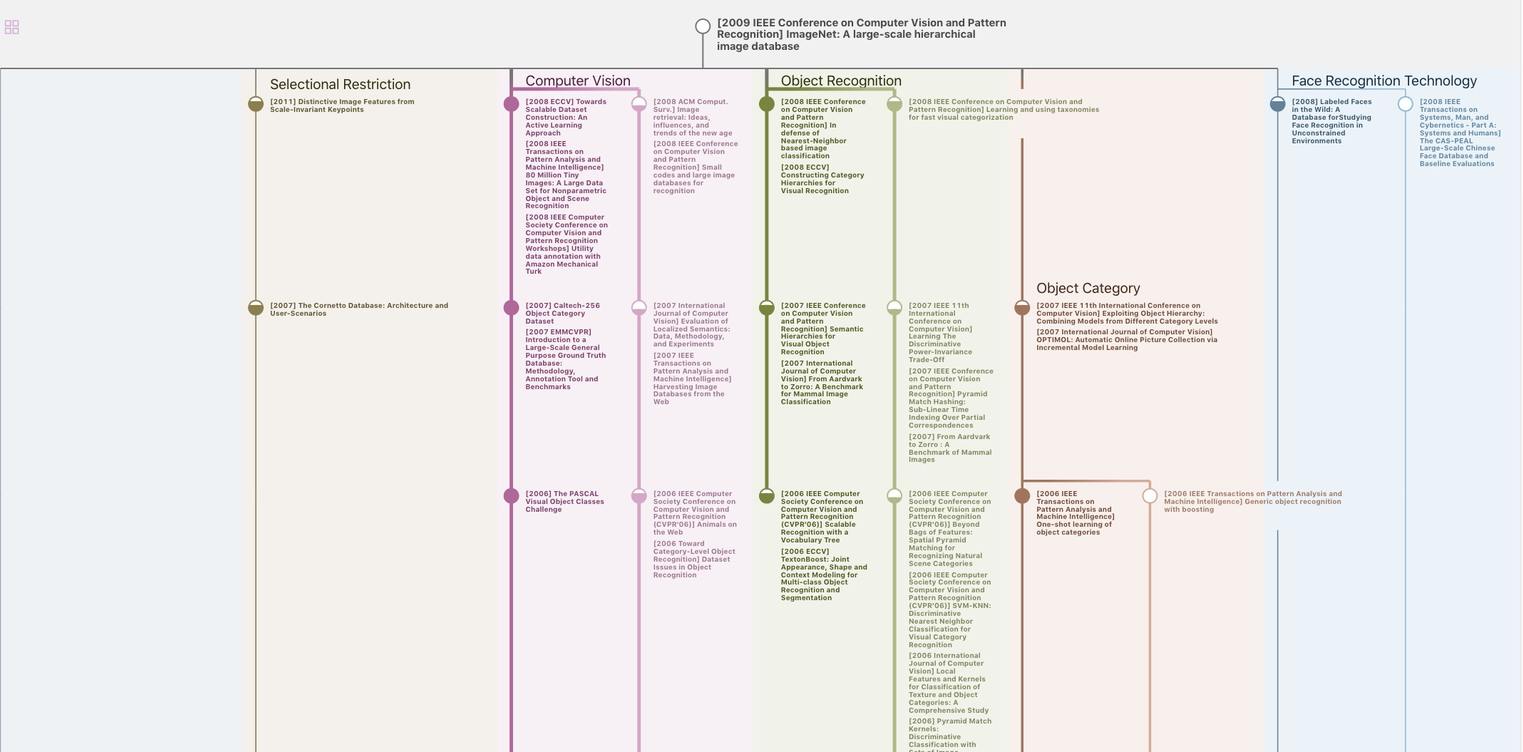
生成溯源树,研究论文发展脉络
Chat Paper
正在生成论文摘要