Optimizing transformer for large-hole image inpainting
2023 IEEE INTERNATIONAL CONFERENCE ON IMAGE PROCESSING, ICIP(2023)
摘要
In recent years, leveraging Convolutional Neural Network (CNN) to optimize Transformer (called hybrid model) has achieved great progress in image inpainting. However, the slow growth of the effective receptive field of CNN in processing large-hole regions significantly limits the overall performance. To alleviate this problem, this paper proposes a new Transformer-CNN-based hybrid framework (termed PUT+) by introducing the fast Fourier convolution (FFC) into the CNN-based refinement network. The proposed framework introduces an improved Patch-based Vector Quantized Variational Auto-Encoder (P-VQVAE+). The encoder transforms the masked region into non-overlapping patch-based unquantized feature vectors as the input of Un-Quantized Transformer (UQ-Transformer). The decoder restores the masked region from the predicted quantized features output by the UQ-Transformer while maintaining the unmasked region unchanged. Many experimental results show that the proposed method outperforms the state-of-the-art by a large margin, especially for image inpainting with large masked areas. The code is available at https://github.com/GZHU-DVL/PUTplus.
更多查看译文
关键词
Image inpainting,Transformer,fast Fourier convolution,receptive field,information loss
AI 理解论文
溯源树
样例
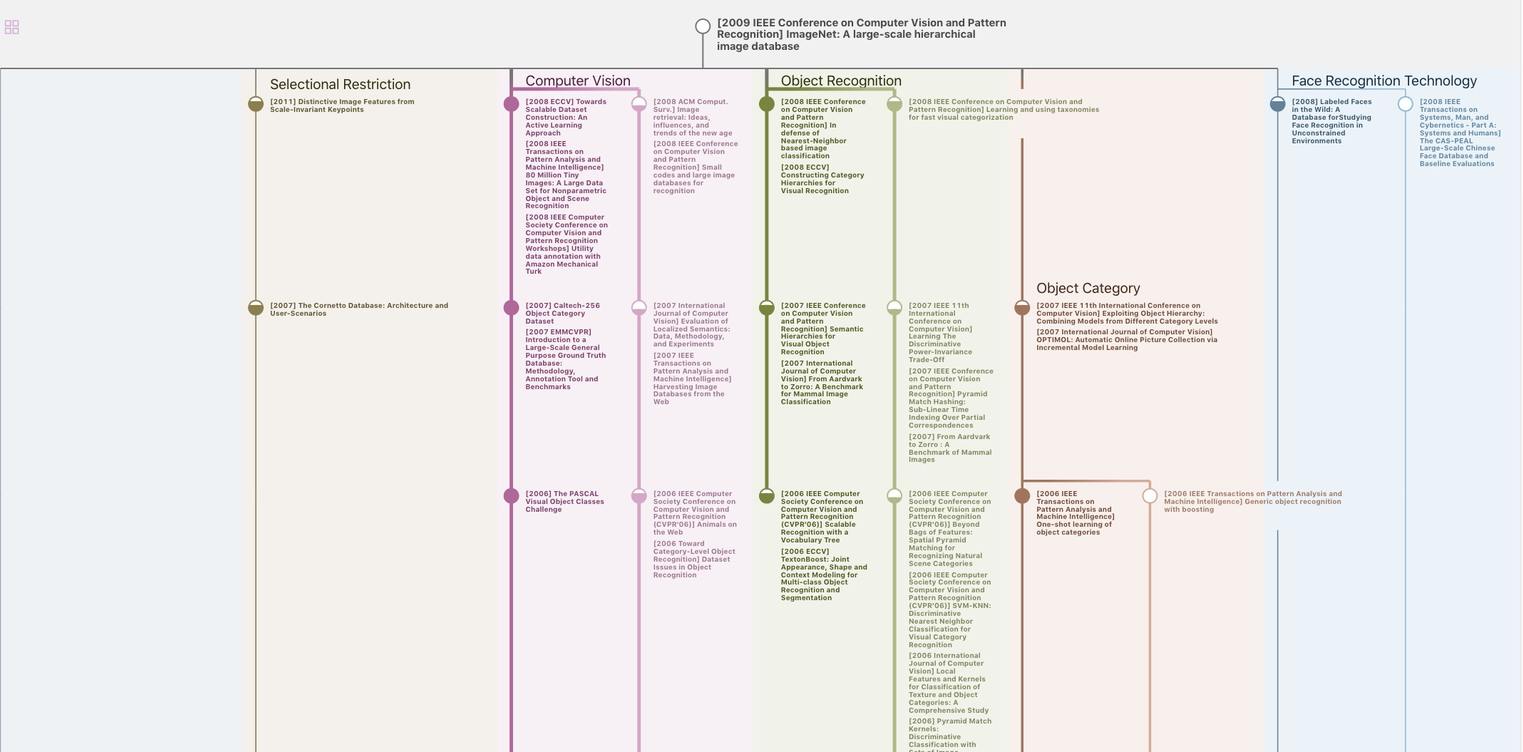
生成溯源树,研究论文发展脉络
Chat Paper
正在生成论文摘要