Leveraging efficient training and feature fusion in transformers for multimodal classification
2023 IEEE INTERNATIONAL CONFERENCE ON IMAGE PROCESSING, ICIP(2023)
摘要
People navigate a world that involves many different modalities and make decision on what they observe. Many of the classification problems that we face in the modern digital world are also multimodal in nature, where textual information on the web rarely occurs alone, and is often accompanied by images, sounds, or videos. The use of transformers in deep learning tasks has proven to be highly effective. However, the relationship between different modalities remains unclear. This paper investigates ways to simultaneously utilize self-attention over both text and vision modalities. We propose a novel architecture that combines the strengths of both modalities. We show that combining a text model with a fixed image model leads to the best classification performance. Additionally, we incorporate a late fusion technique to enhance the architecture's ability to capture multiple modalities. Our experiments demonstrate that our proposed method outperforms state-of-the-art baselines on Food101, MM-IMDB, and FashionGen datasets.
更多查看译文
关键词
Multimodal,classification,transformers,feature fusion,efficient training
AI 理解论文
溯源树
样例
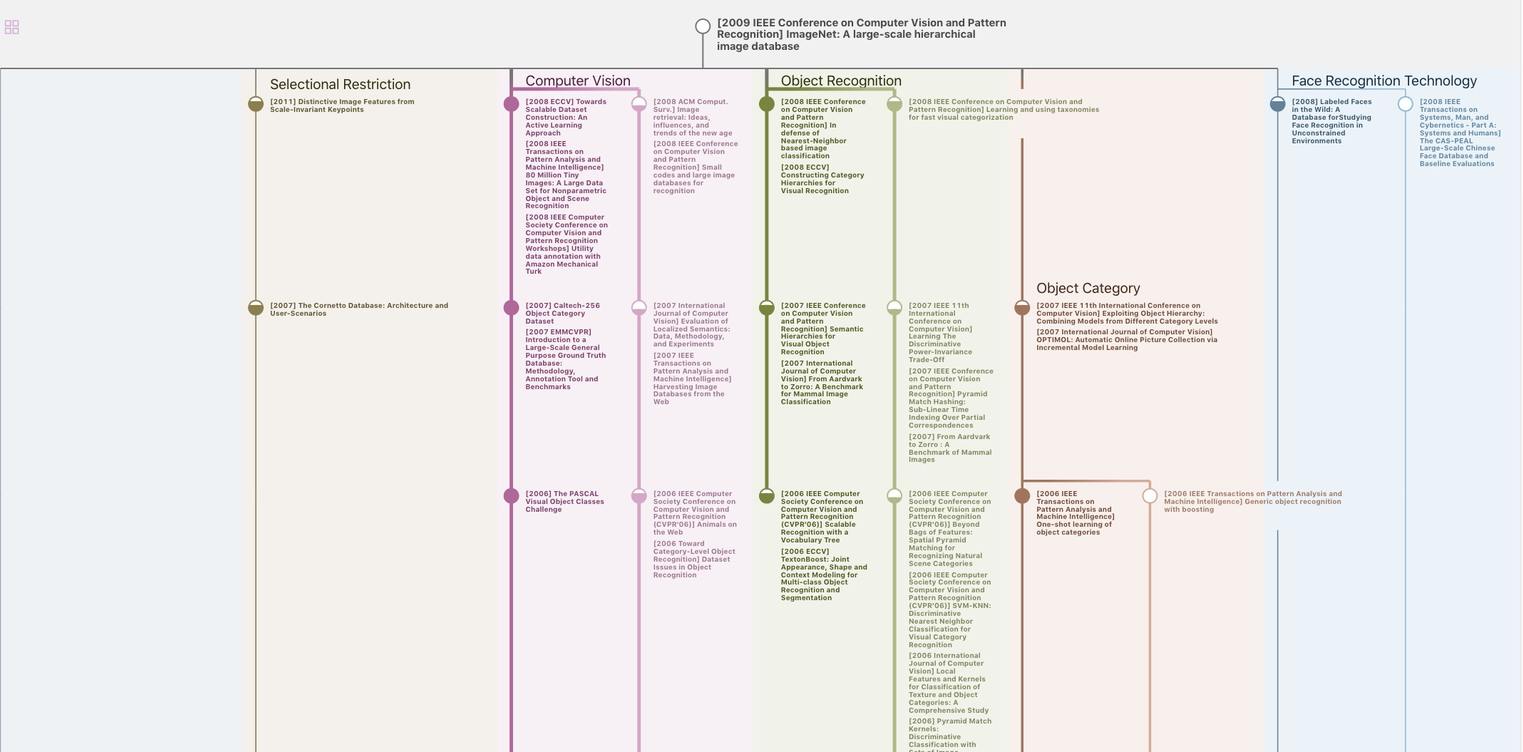
生成溯源树,研究论文发展脉络
Chat Paper
正在生成论文摘要