Skeleton action recognition based on spatio-temporal features
2023 IEEE INTERNATIONAL CONFERENCE ON IMAGE PROCESSING, ICIP(2023)
摘要
Graph convolutional networks have performed well in action recognition tasks using skeleton data, but topology extraction remains a challenge. Most methods fail to consider the correlations between channels in skeleton topology graphs. To address this, we propose a multi-channel optimal graph convolutional network (MOGCN) that combines multi-channel optimal graph convolution and multi-scale temporal convolution techniques. MOGCN exhibits improved capability in processing spatio-temporal information and node relationships, generating optimal skeleton topology graphs that model correlations between all nodes in the sequence. We also use multi-scale temporal convolution to extract temporal features, improving the modeling of long-term correlations and global correlations of spatio-temporal joints. We further improve accuracy using a multi-stream fusion network model. Experiments on multiple datasets show that the proposed MOGCN module is effective and improves the classification accuracy of skeleton-based action recognition.
更多查看译文
关键词
Action Recognition,Graph Convolutional Network,Skeleton Data
AI 理解论文
溯源树
样例
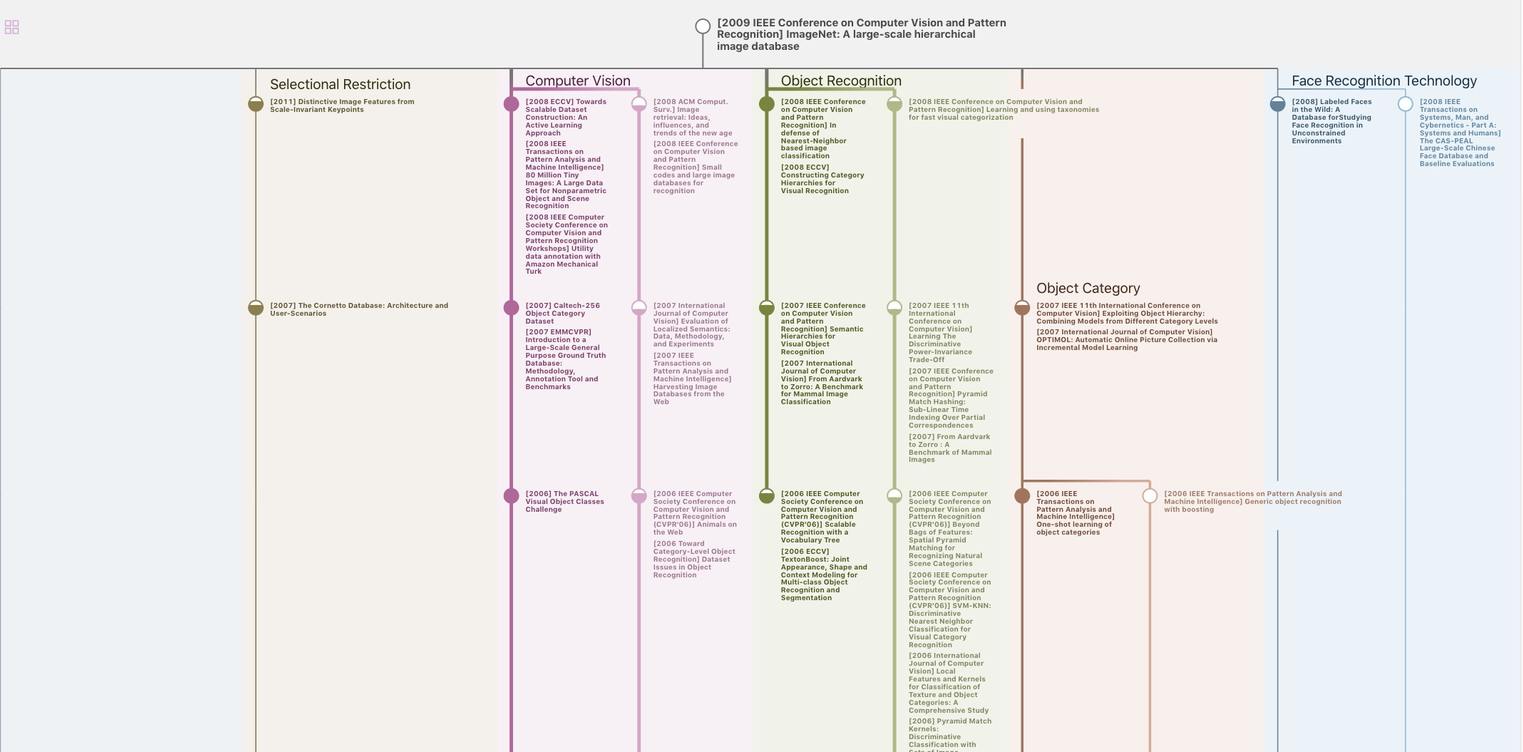
生成溯源树,研究论文发展脉络
Chat Paper
正在生成论文摘要