Hierarchical multi-task learning via task affinity groupings
2023 IEEE INTERNATIONAL CONFERENCE ON IMAGE PROCESSING, ICIP(2023)
摘要
Multi-task learning (MTL) permits joint task learning based on a shared deep learning architecture and multiple loss functions. Despite the recent advances in MTL, one loss often dominates the learning optimization in multiple unrelated tasks. This often results in poor performance compared to the corresponding single task learning. To overcome the aforementioned "negative transfer", we propose a novel hierarchical framework that leverages task relations via inter-task affinity to supervise multi-task learning. Specifically, the inter-task affinity generated task sets, with low-level task set and complex task set at the bottom and top layers respectively, enables iterative multi-task information sharing. In addition, it also alleviates simultaneous image annotations for multiple tasks. The proposed framework achieves state-of-the-art results on classification, detection, semantic segmentation and depth estimation across three standard benchmarks. Furthermore, with state of the results on two benchmarks for image retrieval task, we also demonstrate that the embeddings learned using such a framework provide good generalization and robust representation learning.
更多查看译文
关键词
Multi-task Learning,Detection,Semantic Segmentation,Depth Prediction,Inter-Task Affinity
AI 理解论文
溯源树
样例
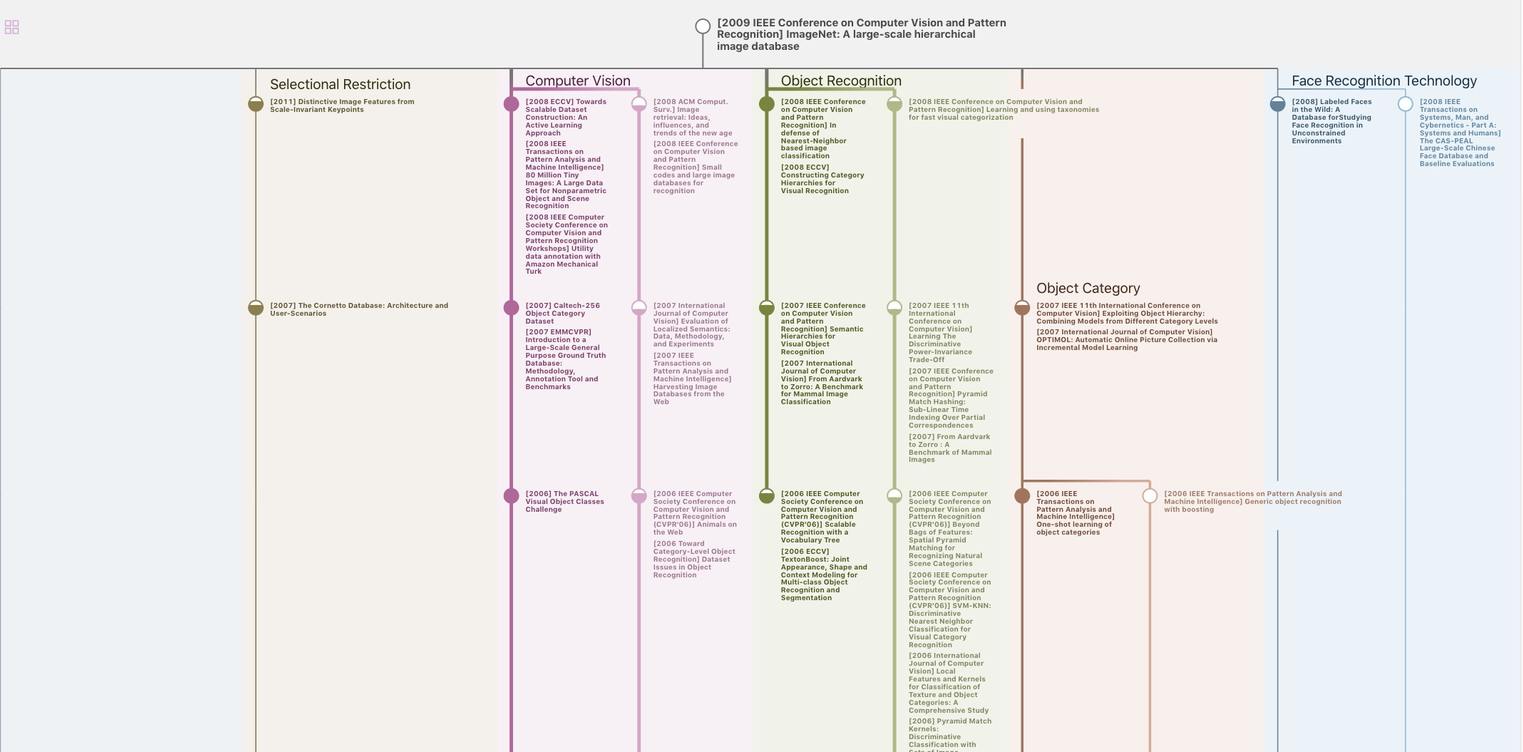
生成溯源树,研究论文发展脉络
Chat Paper
正在生成论文摘要