Prompt prototype learning based on ranking instruction for few-shot visual tasks
2023 IEEE INTERNATIONAL CONFERENCE ON IMAGE PROCESSING, ICIP(2023)
摘要
Querying large language models (LLMs), such as GPT-3, for high-quality prompts and utilizing pre-trained vision-language models, such as CLIP, to construct a zero-shot visual classification model, offer promising performance across various downstream visual tasks. However, when applied to specific domains, their efficacy is restricted due to the gap between the general prompts they generate and the required domain-specific knowledge. In this paper, we propose a novel, lightweight method for prompt prototype learning through ranking instruction, specifically designed to bridge this gap in the context of few-shot visual classification. We generate domain-specific prompts leveraging the knowledge contained in LLMs and then fine-tune the prompt prototype with effective ranking instructions from several domain images. Our few-shot experiments on facial expression benchmarks demonstrate the efficacy of the prompt prototype. Notably, our method delivers results that are on par with state-of-the-art few-shot image classification techniques and can be integrated with them to further improve performance in the facial expression domain. Our approach provides a promising solution to few-shot visual classification, making use of the knowledge contained in LLMs to generate domain-specific prompts.
更多查看译文
关键词
Prompt prototype,Few-hot,Ranking instruction,Large-scale pre-training models
AI 理解论文
溯源树
样例
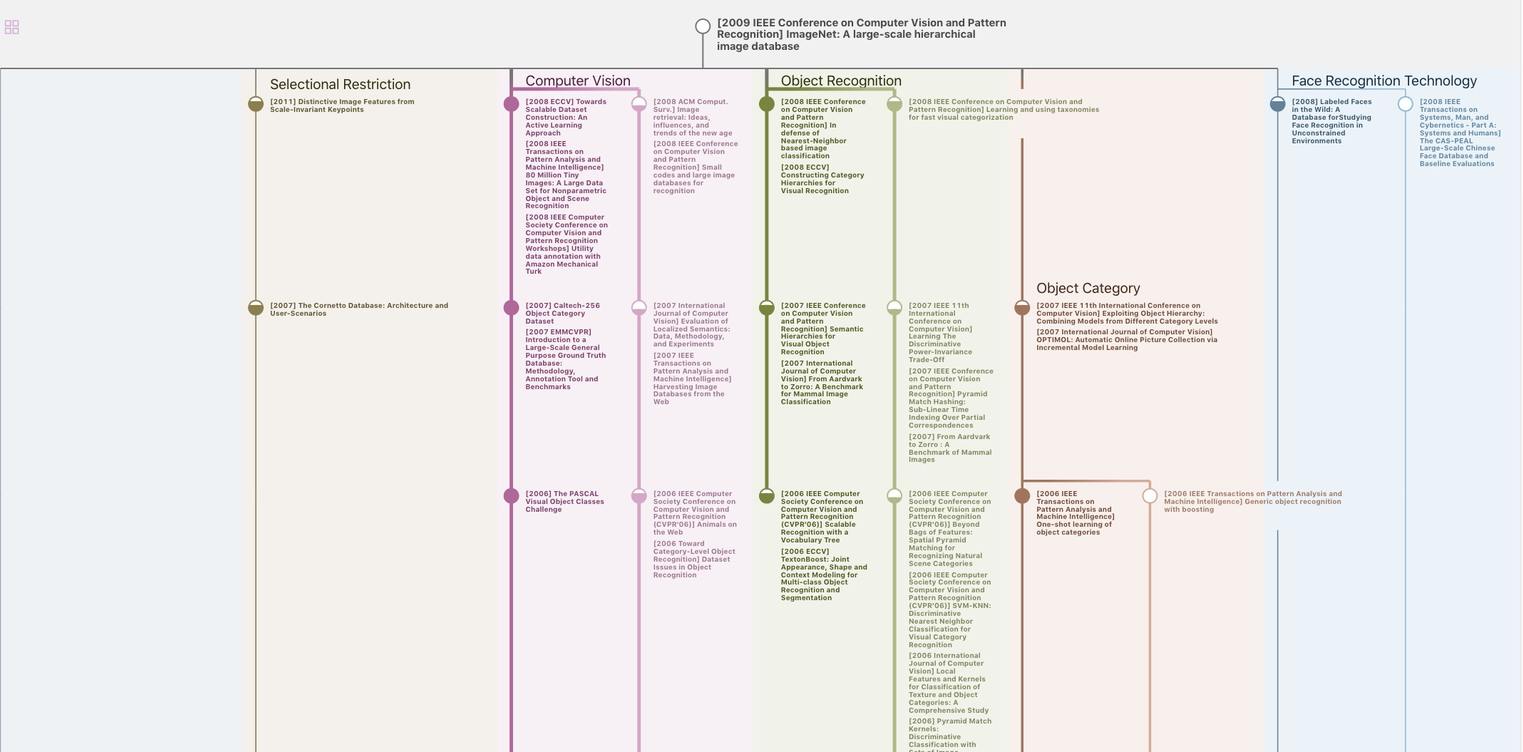
生成溯源树,研究论文发展脉络
Chat Paper
正在生成论文摘要