Efficient Transfer by Robust Label Selection and Learning with Pseudo-Labels
2023 IEEE INTERNATIONAL CONFERENCE ON IMAGE PROCESSING, ICIP(2023)
摘要
Semi-supervised techniques have been successful in reducing the amount of labels needed to train a neural network. Often these techniques focus on making the most out of the given labels and exploiting the unlabeled data. Instead of considering the labels as a given, we start with focusing on how to select good labels for efficient semi-supervised learning with pseudolabels. We propose CLaP: Clustering, Label selection and Pseudolabels. It clusters an unlabeled dataset on an embedding, that was pretrained on large scale dataset (ImageNet1k). We use the cluster centers for querying initial labels that are both representative and diverse. We use samples consistent over multiple clustering runs as pseudo-labels. We propose a mixed loss on both the initial labels and the pseudo-labeled data to train a neural network. With CLaP the samples to be annotated are automatically selected, reducing the load by a human annotator to search for these samples. We demonstrate that CLaP outperforms of state-of-theart methods in few-shot transfer tasks on full datasets by 20% on 1-shot to 3% on 5-shot. It also outperforms the accuracy of the state-of-the-art in the BSCD-FSL benchmark up to 23%, depending on the dataset and amount of labels.
更多查看译文
关键词
few shot learning,clustering,label selection,pseudo-labels
AI 理解论文
溯源树
样例
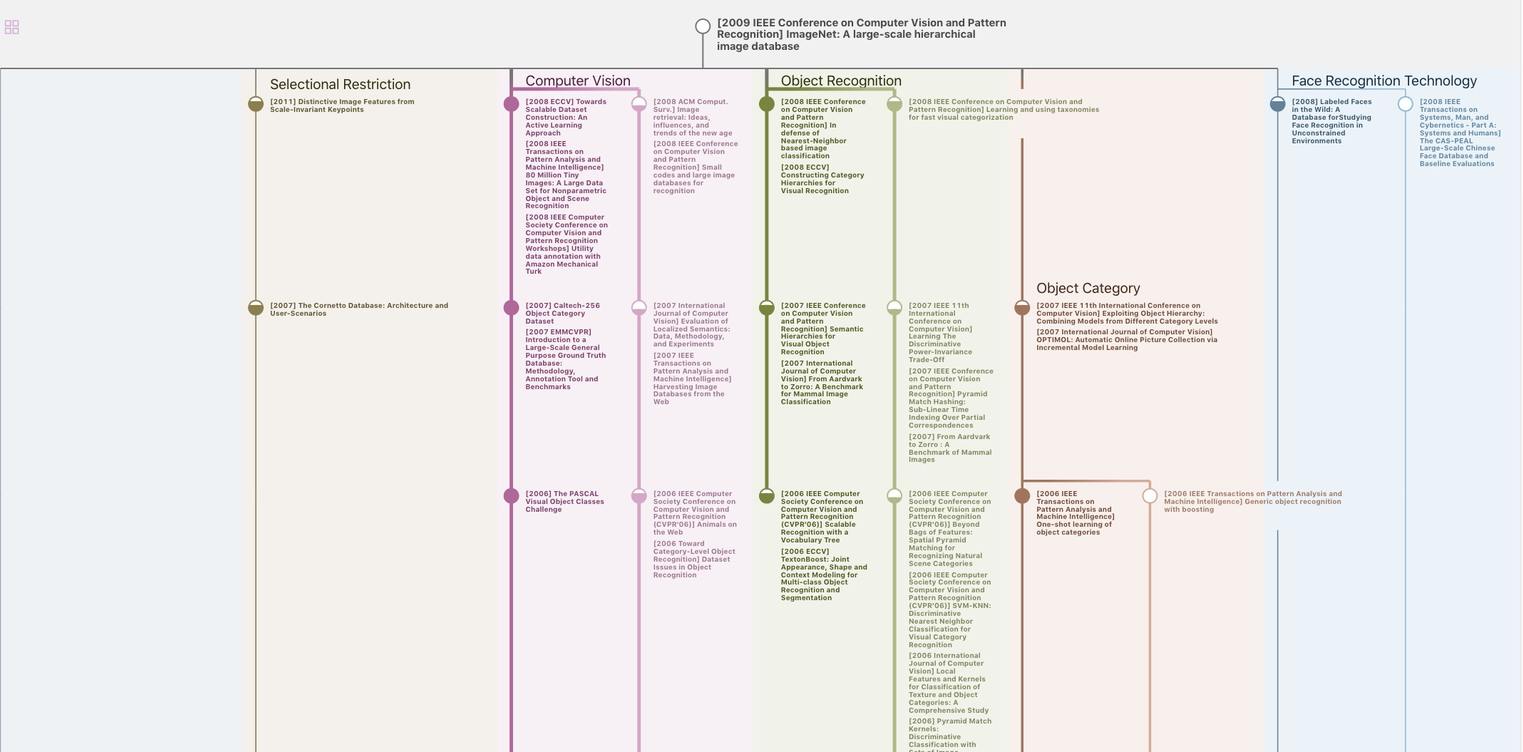
生成溯源树,研究论文发展脉络
Chat Paper
正在生成论文摘要