Application of machine learning models to identify serological predictors of COVID-19 severity and outcomes.
Research square(2023)
摘要
Critically ill people with COVID-19 have greater antibody titers than those with mild to moderate illness, but their association with recovery or death from COVID-19 has not been characterized. In 178 COVID-19 patients, 73 non-hospitalized and 105 hospitalized patients, mucosal swabs and plasma samples were collected at hospital enrollment and up to 3 months post-enrollment (MPE) to measure virus RNA, cytokines/chemokines, binding antibodies, ACE2 binding inhibition, and Fc effector antibody responses against SARS-CoV-2. The association of demographic variables and >20 serological antibody measures with intubation or death due to COVID-19 was determined using machine learning algorithms. Predictive models revealed that IgG binding and ACE2 binding inhibition responses at 1 MPE were positively and C1q complement activity at enrollment was negatively associated with an increased probability of intubation or death from COVID-19 within 3 MPE. Serological antibody measures were more predictive than demographic variables of intubation or death among COVID-19 patients.
更多查看译文
关键词
serological predictors,machine learning models,machine learning,severity
AI 理解论文
溯源树
样例
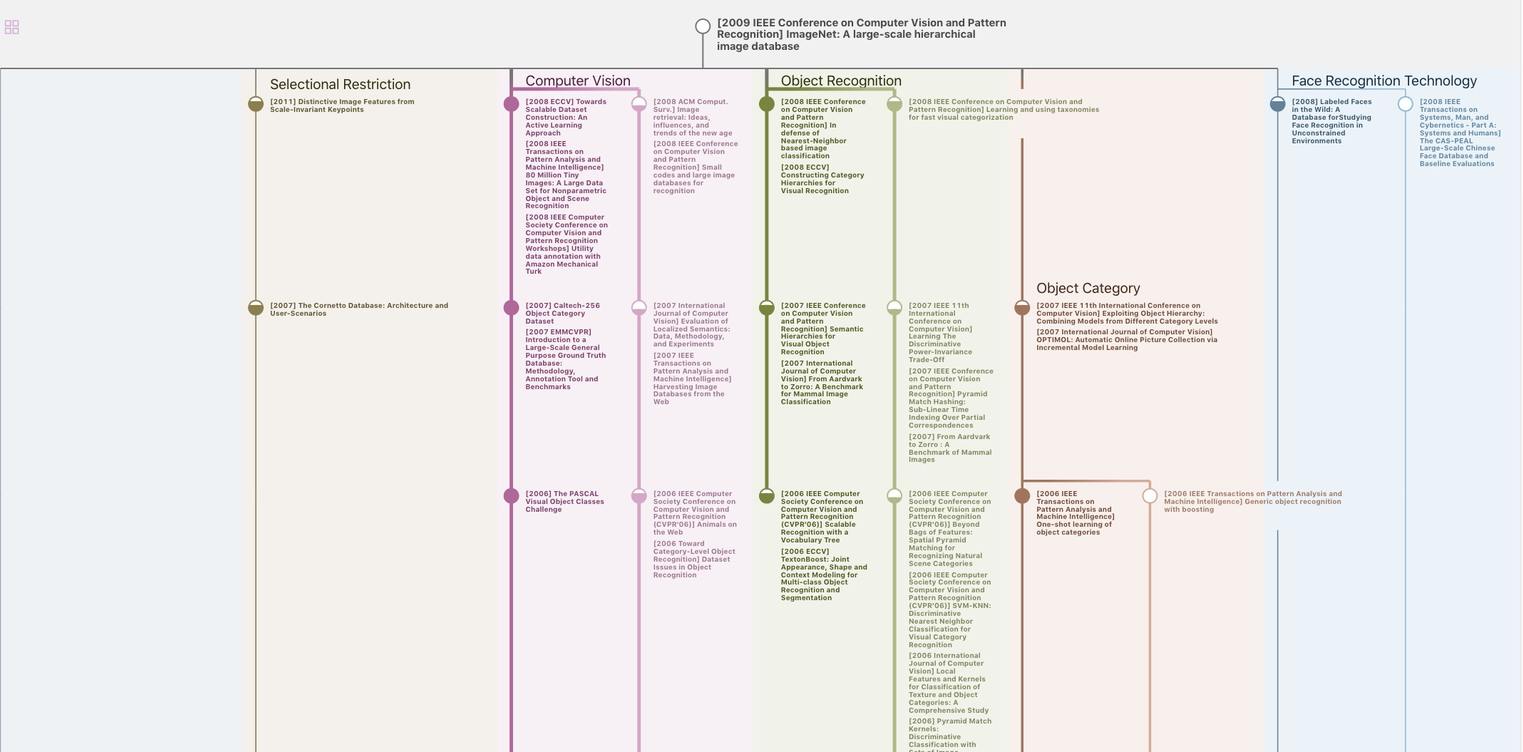
生成溯源树,研究论文发展脉络
Chat Paper
正在生成论文摘要