An Urban Traffic Flow Prediction Approach Integrating External Factors Based on Deep Learning and Knowledge Graph
2023 4th International Conference on Information Science, Parallel and Distributed Systems (ISPDS)(2023)
摘要
In addition to depending on historical traffic data, urban traffic flow forecasting may be interfered by external factors as well. However, the existing traffic forecast models seldom take external factors into account. Therefore, an urban traffic flow forecast approach that considers weather and POI information is presented founded on the premise that adding external factors can heighten the forecast precision in this article. The proposed method firstly constructs an urban traffic knowledge graph to represent the multiple external factors and traffic information, and obtains the knowledge embeddings with a knowledge representation learning model called KR-EAR. After that, the traffic flow features and knowledge embeddings are integrated by a knowledge fusion unit we designed and fed into a spatial-temporal Chebyshev graph convolutional recurrent network. Finally, the attention mechanism is introduced to aggregate global temporal information by adjusting the significance of different time points. The experimental results on real datasets show that the traffic flow prediction task considering external factors is effective compared to traditional traffic forecast methods. Moreover, the forecast performance of the presented approach outperforms baseline models at each horizon setting.
更多查看译文
关键词
traffic flow prediction,knowledge graph,spatial-temporal network,attention mechanism
AI 理解论文
溯源树
样例
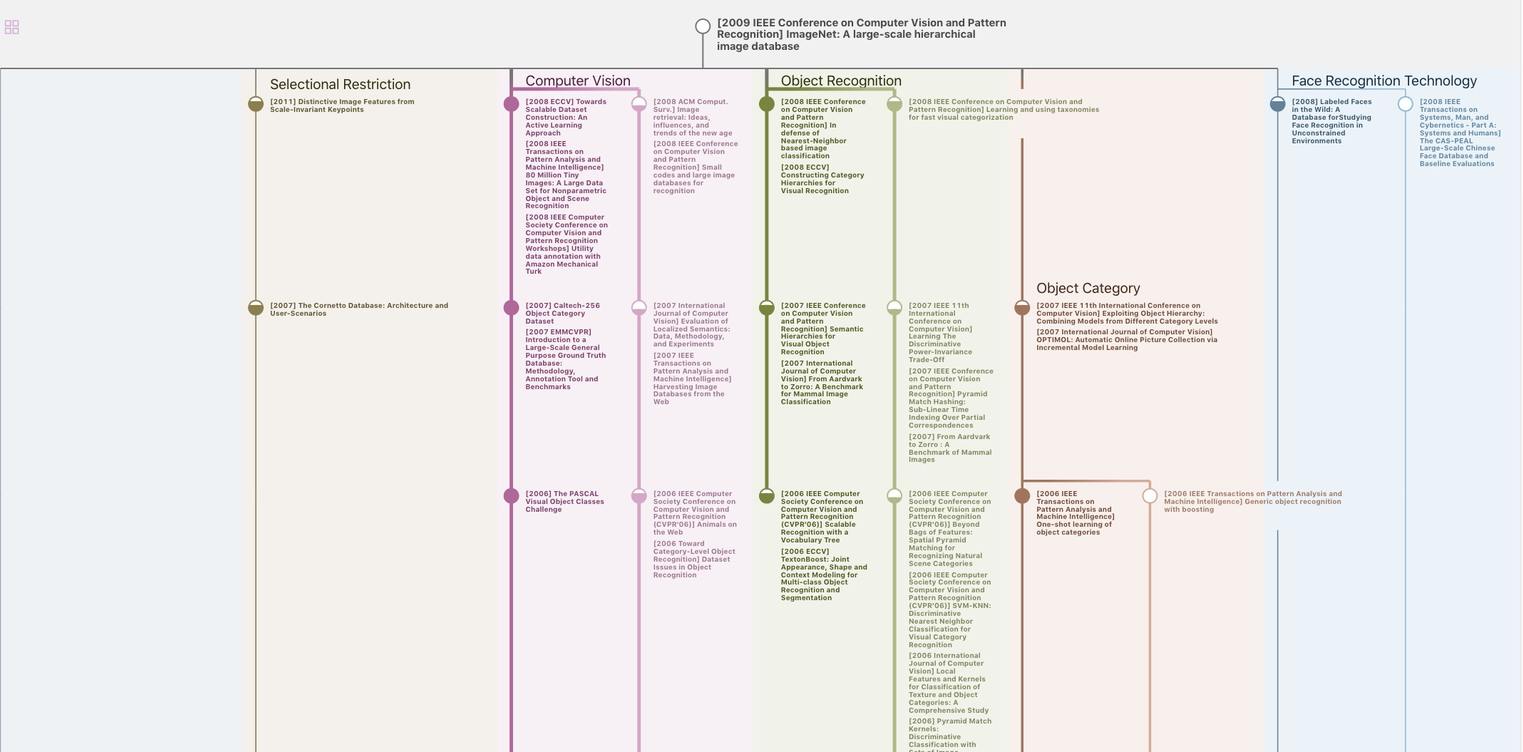
生成溯源树,研究论文发展脉络
Chat Paper
正在生成论文摘要