How Much Speech Data Is Needed for Tracking Language Change in Alzheimer's Disease? A Comparison of Random Length, 5-Min, and 1-Min Spontaneous Speech Samples.
Digital Biomarkers(2023)
摘要
Introduction:Changes in speech can act as biomarkers of cognitive decline in Alzheimer's disease (AD). While shorter speech samples would promote data collection and analysis, the minimum length of informative speech samples remains debated. This study aims to provide insight into the effect of sample length in analyzing longitudinal recordings of spontaneous speech in AD by comparing the original random length, 5- and 1-minute-long samples. We hope to understand whether capping the audio improves the accuracy of the analysis, and whether an extra 4 min conveys necessary information.
Methods:110 spontaneous speech samples were collected from decades of Youtube videos of 17 public figures, 9 of whom eventually developed AD. 456 language features were extracted and their text-length-sensitivity, comparability, and ability to capture change over time were analyzed across three different sample lengths.
Results:Capped audio files had advantages over the random length ones. While most extracted features were statistically comparable or highly correlated across the datasets, potential effects of sample length should be acknowledged for some features. The 5-min dataset presented the highest reliability in tracking the evolution of the disease, suggesting that the 4 extra minutes do convey informative data.
Conclusion:Sample length seems to play an important role in extracting the language feature values from speech and tracking disease progress over time. We highlight the importance of further research into optimal sample length and standardization of methods when studying speech in AD.
更多查看译文
关键词
digital biomarkers,natural language processing,speech,language,alzheimer’s disease,dementia,digital health
AI 理解论文
溯源树
样例
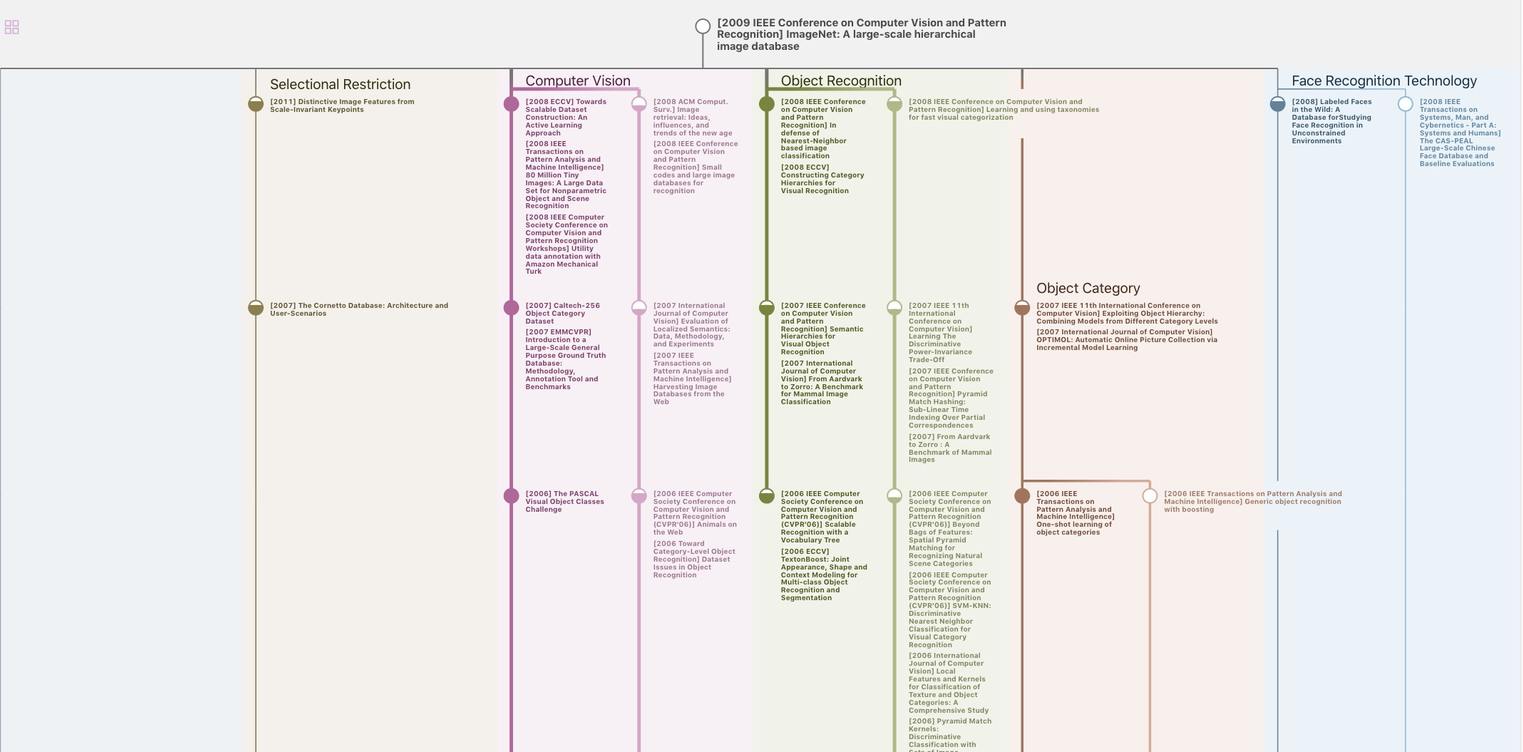
生成溯源树,研究论文发展脉络
Chat Paper
正在生成论文摘要