A Novel Data-Driven Fault Diagnosis Method Based on Semi-Generative Adversarial Network for Buck Converter ICs
2023 IEEE 18th Conference on Industrial Electronics and Applications (ICIEA)(2023)
摘要
DC-DC converter ICs have been widely used in portable electronic products due to their high efficiency and high integration. However, in the manufacturing and use, it will encounter problems such as voltage instability, poor soldering, and component damage, which will affect the normal operation of the entire system. Most of the existing fault diagnosis methods rely on human experience, and the dependence on labeled data is relatively large, which makes the production process time-consuming, laborious and costly. In order to solve these problems, this paper takes buck converter IC as an example and proposes a data-driven fault diagnosis method based on semi-supervised generative adversarial network. Firstly, the fault types reflected in the steady-state ripple test of buck converter ICs are analyzed, and the acquired waveform signal data and fault characteristics are introduced in this paper. Secondly, the network structure of generator and discriminator in semi-supervised generative adversarial network and the training process of model are introduced. The proposed fault diagnosis method does not rely on human experience, which greatly reduces the dependence on labeled data, reduces the cost, and improves the accuracy of fault diagnosis. Finally, a comparative experiment is carried out with the artificial neural network model, which verifies the effectiveness and practicality of the proposed fault diagnosis method.
更多查看译文
关键词
buck converter IC,fault detection,feature extraction,semi-supervised generative adversarial network
AI 理解论文
溯源树
样例
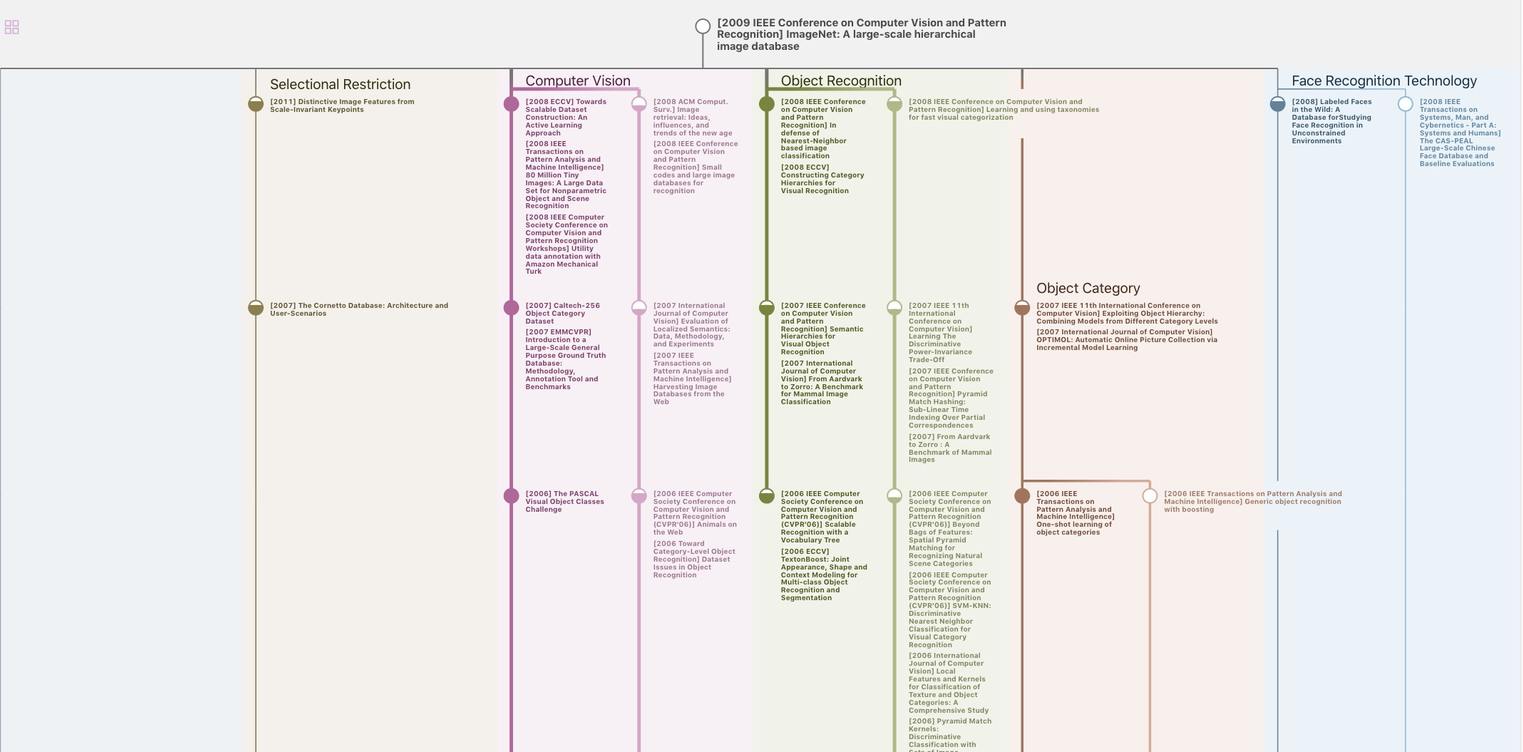
生成溯源树,研究论文发展脉络
Chat Paper
正在生成论文摘要