Loop Closure Detection Based on Geometry of Semantic Point Cloud
2023 IEEE 18th Conference on Industrial Electronics and Applications (ICIEA)(2023)
摘要
The spatiotemporal constraints provided by loop closure detection play a crucial role in the simultaneous localization and mapping of robots. Traditional methods based on key points information are vulnerable to point-level feature instability. In this paper, a loop closure detection method based on robust geometry of the objects extracted through semantics of point cloud is proposed. Our method is based on the fact that point cloud can robustly describe geometric properties, and the extracted local features are extremely robust and invariant to rotation, thus achieving remarkable results in loop closure detection. We conduct experiments on the KITTI odometry dataset and confirm the effectiveness of our method.
更多查看译文
关键词
extracted local features,key points information,KITTI odometry dataset,loop closure detection method,point-level feature instability,robust geometry,semantic point cloud,simultaneous localization and mapping,spatiotemporal constraints
AI 理解论文
溯源树
样例
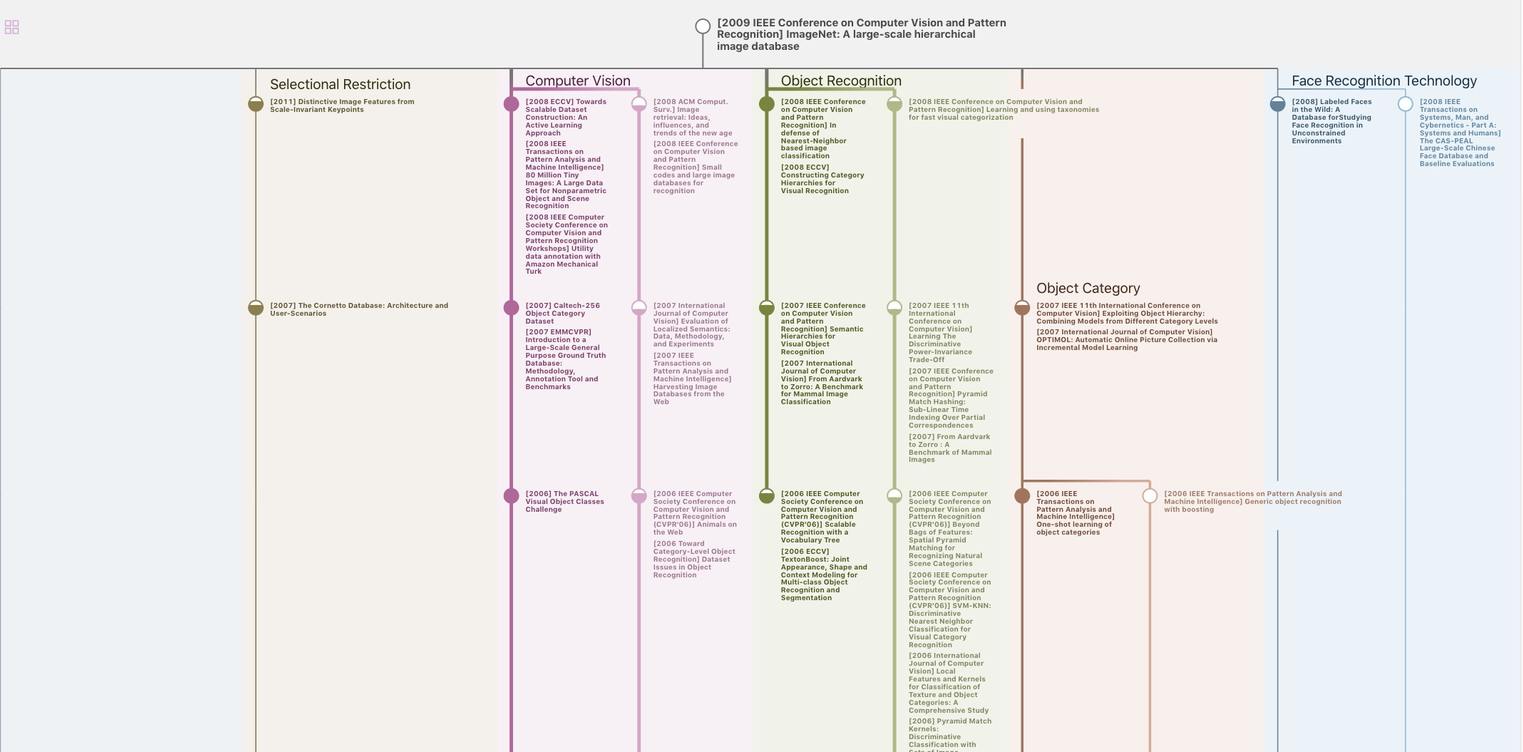
生成溯源树,研究论文发展脉络
Chat Paper
正在生成论文摘要