Multi-Working Conditions Converter Gas Holders Capacities Prediction Based on Improved Multi-LSTM Transfer Ensemble
2023 42nd Chinese Control Conference (CCC)(2023)
摘要
The Linz-Donawitz converter Gas (LDG) produced in the production process of steel enterprises will be stored in the gas holder for supply to other production units in the plant. The prediction of the change trend of the capacity or level of the gas holder can provide a reference for the control and scheduling of the LDG system. Considering that in the actual production process, multiple gas holders' working conditions are constantly switched through pressure valves, resulting in a single data prediction model difficult to obtain relatively stable prediction accuracy under various working conditions. Therefore, this paper proposes a prediction method of converter gas holder capacity for multi-operating conditions. First of all, the sample data is divided into several working condition subsets according to the opening and closing status of the gas holder valve. The LSTM network unit with multi-input-output structure is designed for the multi-working condition modeling problem, and the information of multi-condition data is fused to train the data of multi-condition simultaneously. Among them, a transfer learning method with regularization constraints is proposed for the small sample working condition model. The model trained from the large sample working condition is used as the pre-trained model, and the small sample working condition data is used to fine-tune the pre-trained model. Finally, ensemble learning is used to fuse each working condition model and the global model trained by all data. In order to verify the effectiveness of the proposed method, a real data set from the by-product gas system of a domestic steel plant was selected for the experiment and compared with the state-of-the-art method. The experiment shows that the method proposed in this paper can make stable and accurate predictions under the switching of working conditions, and can provide support for the optimal scheduling of the gas system.
更多查看译文
关键词
Steel Industry,converter gas prediction,LSTM,ensemble learning,transfer learning
AI 理解论文
溯源树
样例
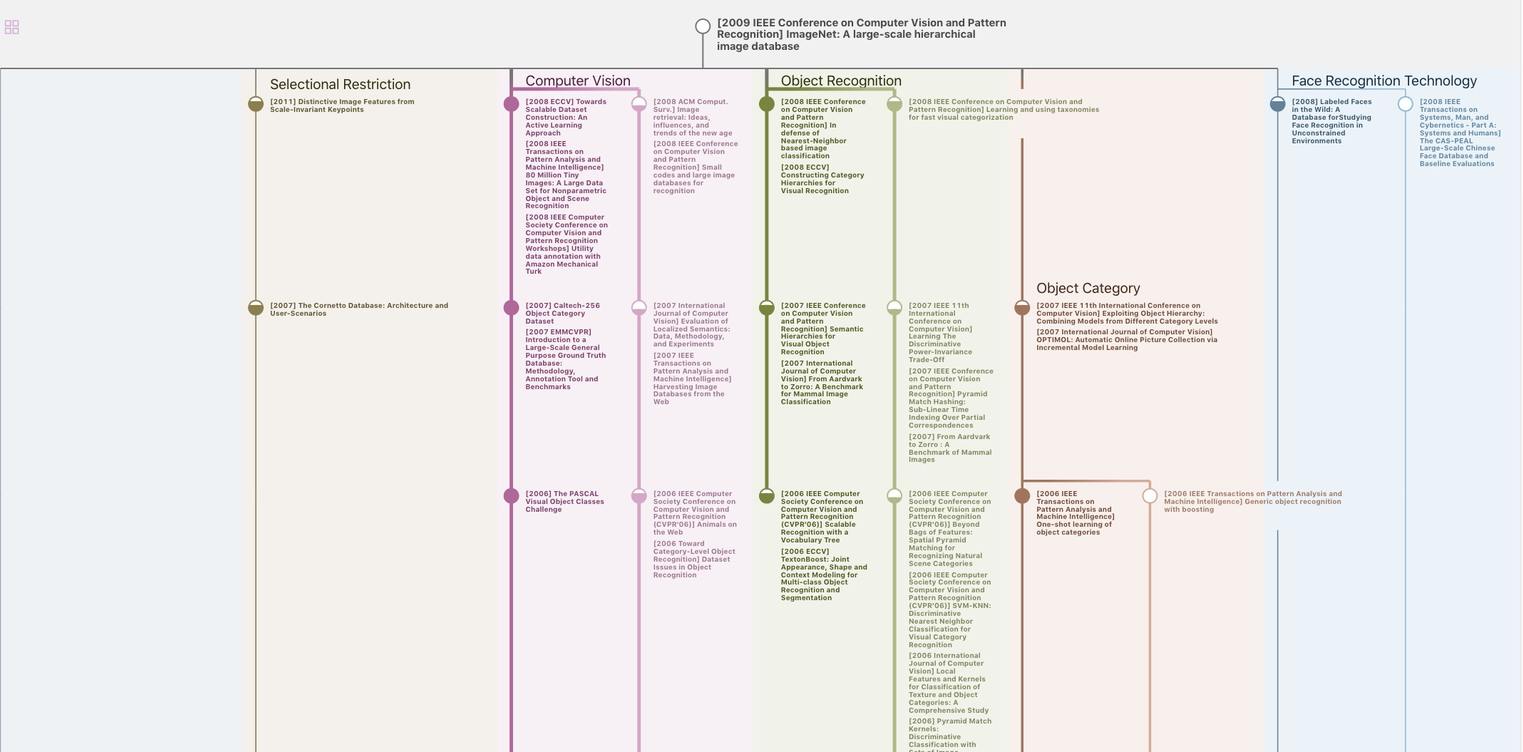
生成溯源树,研究论文发展脉络
Chat Paper
正在生成论文摘要