Improved RetinaNet-Based Defect Detection for Engine Parts
2023 42nd Chinese Control Conference (CCC)(2023)
摘要
The safety of aviation, vehicles, and ships relies heavily on the quality assurance of engine parts. However, traditional target detection algorithms currently used to detect defects in engine parts do not have a high recognition rate. This paper proposes an improved RetinaNet to address this problem. Firstly, the backbone network ResNet was improved by replacing
$7\times 7$
convolutional kernels with stacked
$3\times 3$
convolutional kernels, and the C2 layer of ResNet was densely connected to C3, C4, and C5 layers to improve the feature extraction capability. Then we improved the feature pyramid (FPN) structure by adding a convolutional attention module (CBAM) after layers P3 to P5 of the feature pyramid. Finally, the improved RetinaNet was trained on a self-made engine part missing detection dataset, and the model performance was tested separately. The experimental results show that RetinaNet has better performance in detecting engine part defects, but there is a small part of missed detection. The improved model performs well in detecting small targets, and the mean average precision (mAP) reaches 88.4%, which is 1.8% higher than the mAP of the original RetinaNet, furthermore, the average detection time is 0.22s. In the practical scene application, it can quickly and accurately detect defects in engine parts.
更多查看译文
关键词
Machine vision,engine part detection,deep learning,target detection,image recognition,RetinaNet
AI 理解论文
溯源树
样例
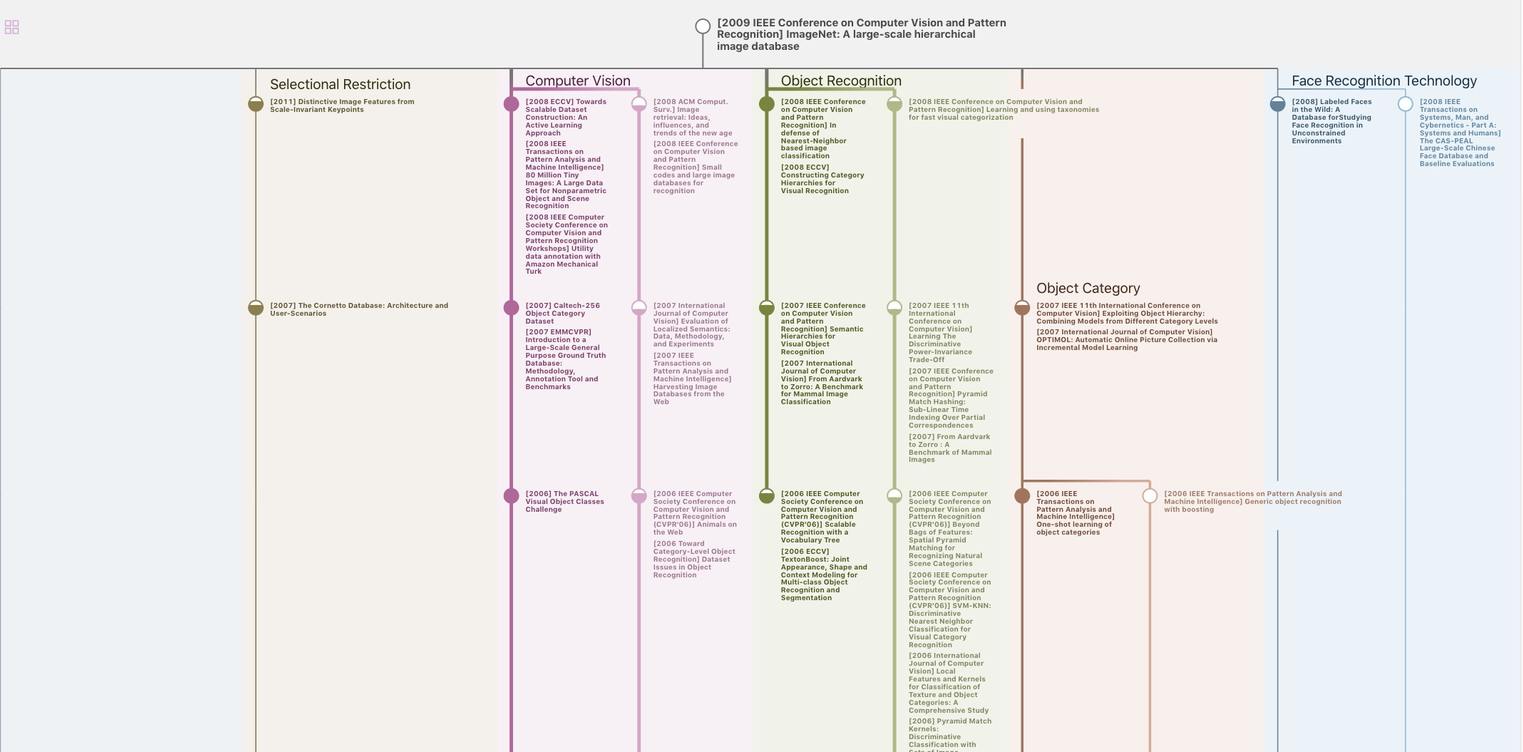
生成溯源树,研究论文发展脉络
Chat Paper
正在生成论文摘要