Improved Unbalance Data Classification Performance Based on Fused SMOTE-RBU
2023 42nd Chinese Control Conference (CCC)(2023)
摘要
To address the extreme imbalance between counterfeit and normal parcels, the courier industry must consider the impact of this factor in the prediction of counterfeit cigarette crime. Otherwise, the performance of the prediction model for unknown parcels will be severely compromised. To improve the accuracy and solve the misclassification problem for this dataset, this paper proposes an algorithm that incorporates SMOTE-RBU. The algorithm first synthesises minority class samples using the SMOTE algorithm, then removes redundant samples from the majority class samples using the RBU algorithm, and finally merges the processed minority class samples with the majority class samples to obtain a balanced dataset that is fed into the classifier for classification prediction. In this study, we tested six different sampling methods (RUS,ROS,Adasyn, SMOTE,SmoteTomek and SMOTE-RBU) and compared five machine learning algorithms (K-Nearest Neighbour, Logistic Regression, Decision Tree, Random Forest, AdaBoost) and four dataset sizes. The experimental results show that the SMOTE-RBU algorithm combined with the RF classifier performs best, with false positives of 0.0165, 0.0179, 0.0126 and 0.0102 on different datasets. Therefore, due to space limitations, only the results of different sampling algorithms combined with the SMOTE-RBU sampling method proposed in this paper for the RF classifier are presented.
更多查看译文
关键词
SMOTE-RBU,Imbalanced data,Machine Learning,Deep Learning
AI 理解论文
溯源树
样例
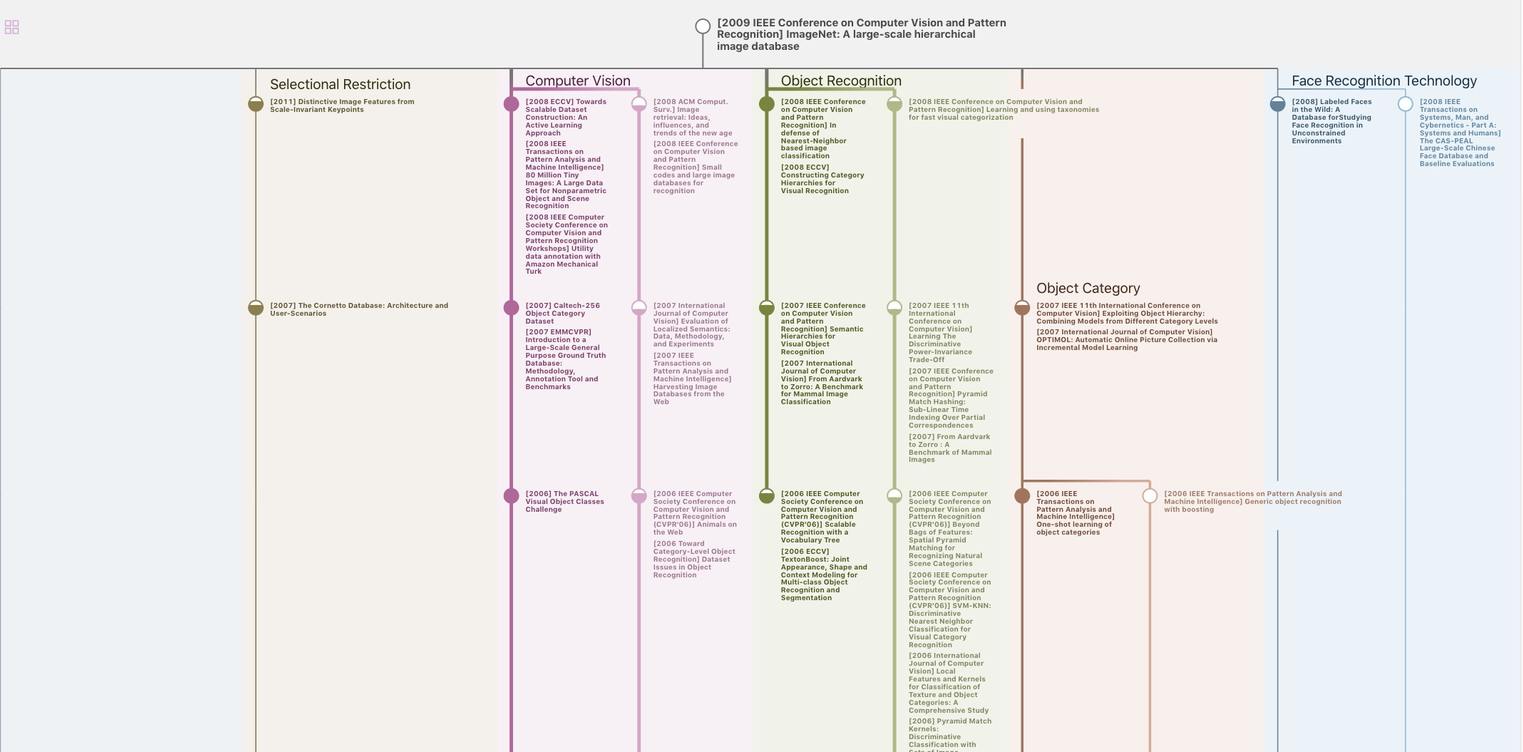
生成溯源树,研究论文发展脉络
Chat Paper
正在生成论文摘要