Data-Driven Hyperparameter Tuning for Point-Based 3D Semantic Segmentation
2023 IEEE International Conference on Image Processing Challenges and Workshops (ICIPCW)(2023)
摘要
Successfully applying state-of-the-art 3D semantic segmentation networks like PointNeXt to new datasets requires setting dataset-specific hyperparameters to suitable values. Specifically, the voxel grid size (for point cloud sampling) and query ball radius (for grouping) play a crucial role in many point-based architectures as they jointly determine the receptive field. Tuning these parameters via sweeping or trial-and-error is both time-consuming and computationally expensive. We therefore propose a training-free, data-driven method for automatically tuning the voxel grid size and query ball radius through a volumetric analysis of the training data. We demonstrate the effectiveness of the approach by evaluating the performance of PointNeXt with default parameters versus parameters set by our auto-tuning method across a diverse set of datasets: Beams&Hooks, ScanNetV2 and SemanticKITTI. Our method improves the mIoU score by 37.4, 0.5 and 26.3 percentage points, respectively, with negligible computational costs. Our code is publicly available
1
1
https://github.com/SimonBuusJensen/AutoTune3DSemanticSeg.
更多查看译文
关键词
3D point clouds,3D semantic segmentation,hyperparameter tuning
AI 理解论文
溯源树
样例
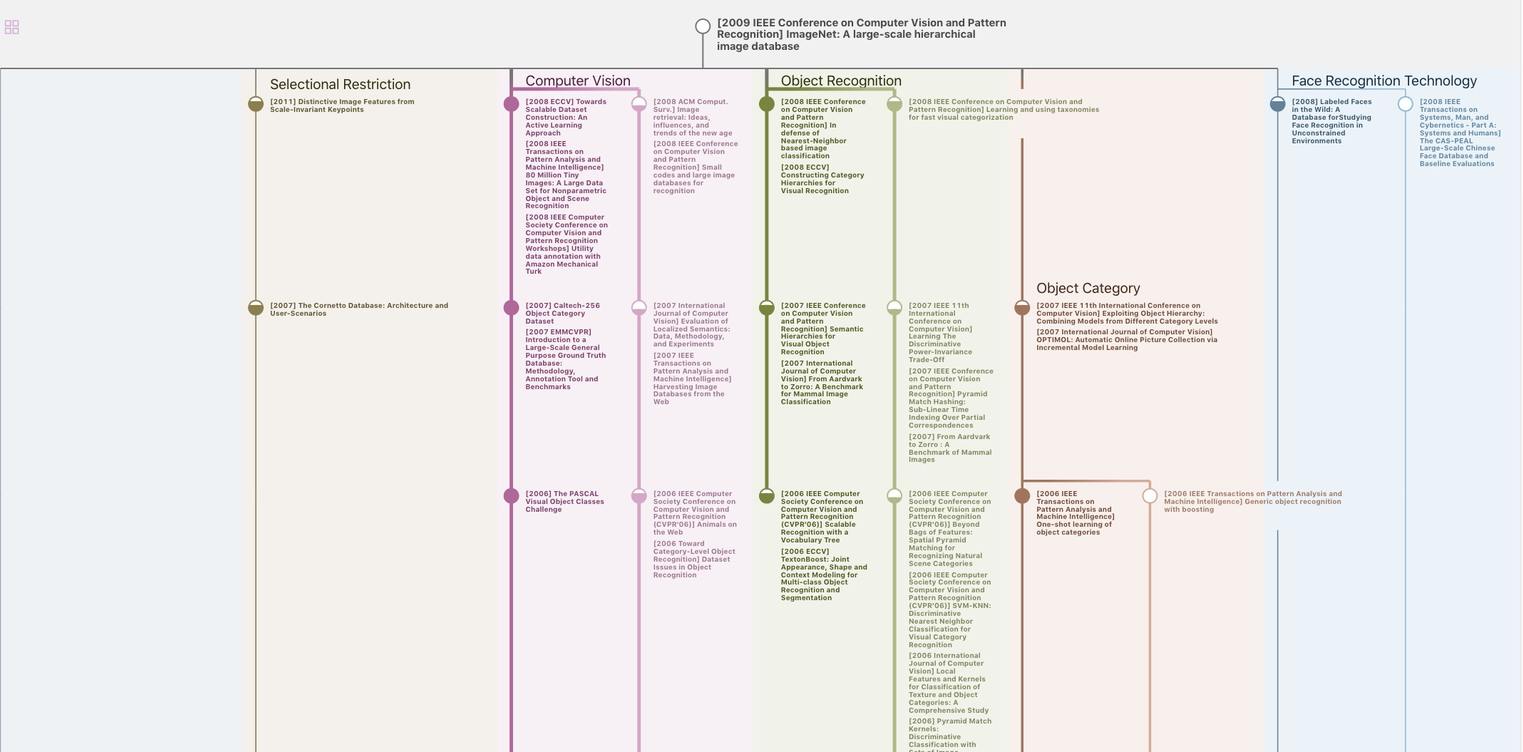
生成溯源树,研究论文发展脉络
Chat Paper
正在生成论文摘要