Machine Learning-Based CEMRI Radiomics Integrating LI-RADS Features Achieves Optimal Evaluation of Hepatocellular Carcinoma Differentiation
JOURNAL OF HEPATOCELLULAR CARCINOMA(2023)
摘要
Purpose: To develop and compare various machine learning (ML) classifiers that employ radiomics extracted from contrast-enhanced magnetic resonance imaging (CEMRI) for diagnosing pathological differentiation of hepatocellular carcinoma (HCC), and validate the performance of the best model. Methods: A total of 251 patients with HCCs (n = 262) were assigned to a training (n = 200) cohort and a validation (n = 62) cohort. A collection of 5502 radiomics signatures were extracted from the CEMRI images for each HCC nodule. To reduce redundancy and dimensionality, Spearman rank correlation, minimum redundancy maximum relevance (mRMR), and the least absolute shrinkage and selection operator (LASSO) approach were employed. Eight ML classifiers were trained to obtain the best radiomics model. The performance of each model was evaluated based on the area under the receiver operating characteristic curve (AUC). The radiomics model was integrated with liver imaging reporting and data system (LI-RADS) features to design a combined model. Results: The eXtreme Gradient Boosting (XGBoost)-based radiomics model outperformed other ML classifiers in evaluating pHCC, achieving an AUC of 1.00 and accuracy of 1.00 in the training cohort. The LI-RADS model demonstrated an AUC value of 0.77 and 0.82 in the training and validation cohorts. The combined model exhibited best performance in both the training and validation cohorts, with AUCs of 1.00 and 0.86 for evaluating HCC differentiation, respectively. Conclusion: CEMRI radiomics integrating LI-RADS features demonstrated excellent performance in evaluating HCC differentiation, suggesting an optimal clinical decision tool for individualized diagnosis of HCC differentiation.
更多查看译文
关键词
machine learning,radiomics,LI-RADS,differentiation,hepatocellular carcinoma,HCC
AI 理解论文
溯源树
样例
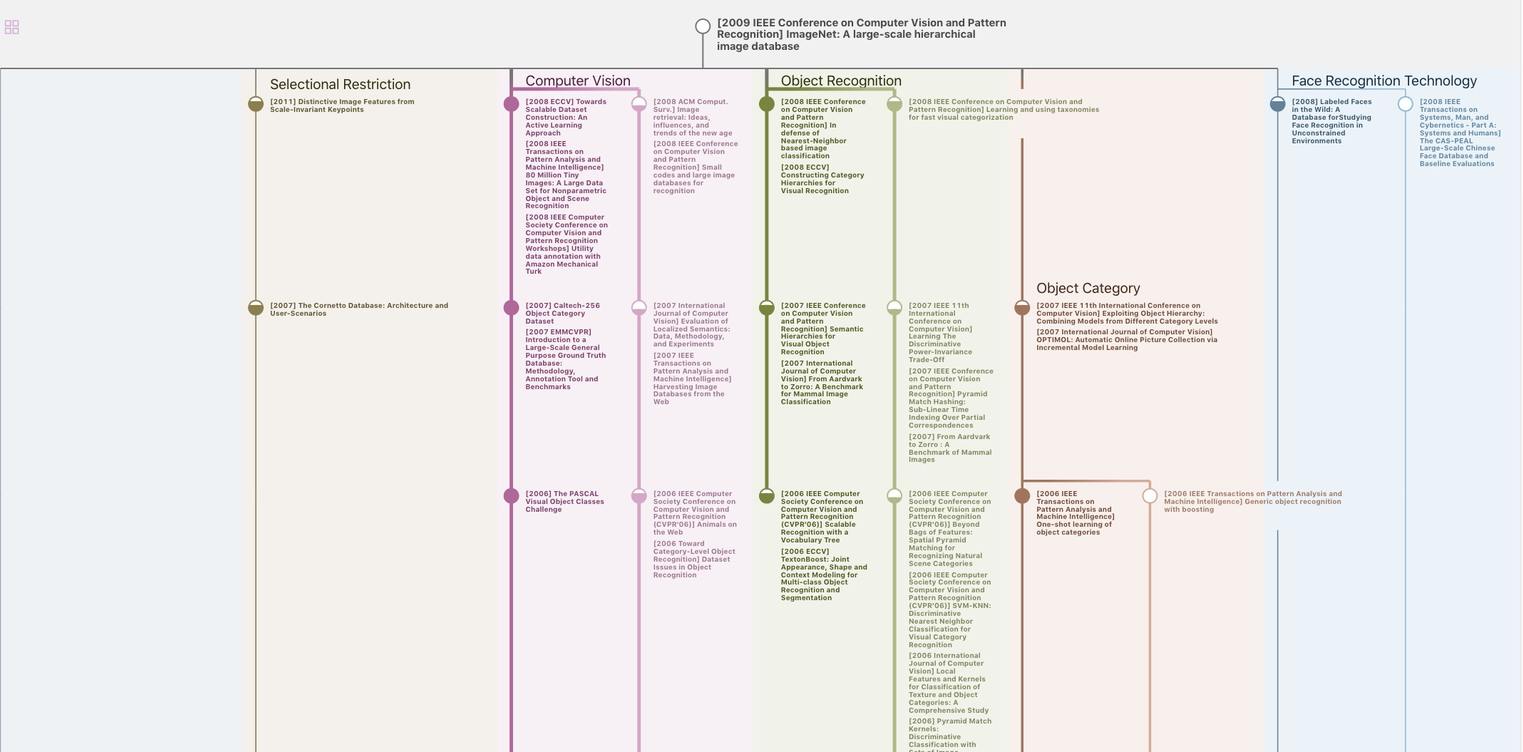
生成溯源树,研究论文发展脉络
Chat Paper
正在生成论文摘要