A BPSO and Deep Learning Based Hybrid Approach for Android Feature Selection and Malware Detection
2022 IEEE 11th International Conference on Communication Systems and Network Technologies (CSNT)(2022)
摘要
With the rapid expansion of smartphone technology, smartphone has become the most influential tool to access the Internet and avail myriad services at a click. On the contrary, app vulnerabilities and malware have become the main threats to the security of Android devices. In general, large datasets are used for malware detection, which may contain several inappropriate and redundant attributes, which can increase the misclassification rate and computational time. As a result, we require a system that can eliminate unwanted features and improve detection accuracy. The authors, here, present a Binary Particle Swarm Optimization (BPSO) and a Deep learning-based highly efficient hybrid approach that helps to address this complex issue of critical relevance. The BPSO method extracts the best features from the search space of API calls, intentions, and command signatures, while Deep Neural Networks (DNNs) assess subsets using data insights. The proposed approach has been experimentally verified with the standard android ‘DREBIN’ dataset. This hybrid approach achieves a significant detection precision of 97.92%, a Recall rate of 96.35 %, an accuracy of 94.92 %, and an F1- score of 92.39% with 72 optimal features.
更多查看译文
关键词
Android Malware Detection,Binary Particle Swarm Optimization (BPSO),Feature Selection,Meta-heuristic algorithm,Deep Learning(DLL),Deep Neural Networks
AI 理解论文
溯源树
样例
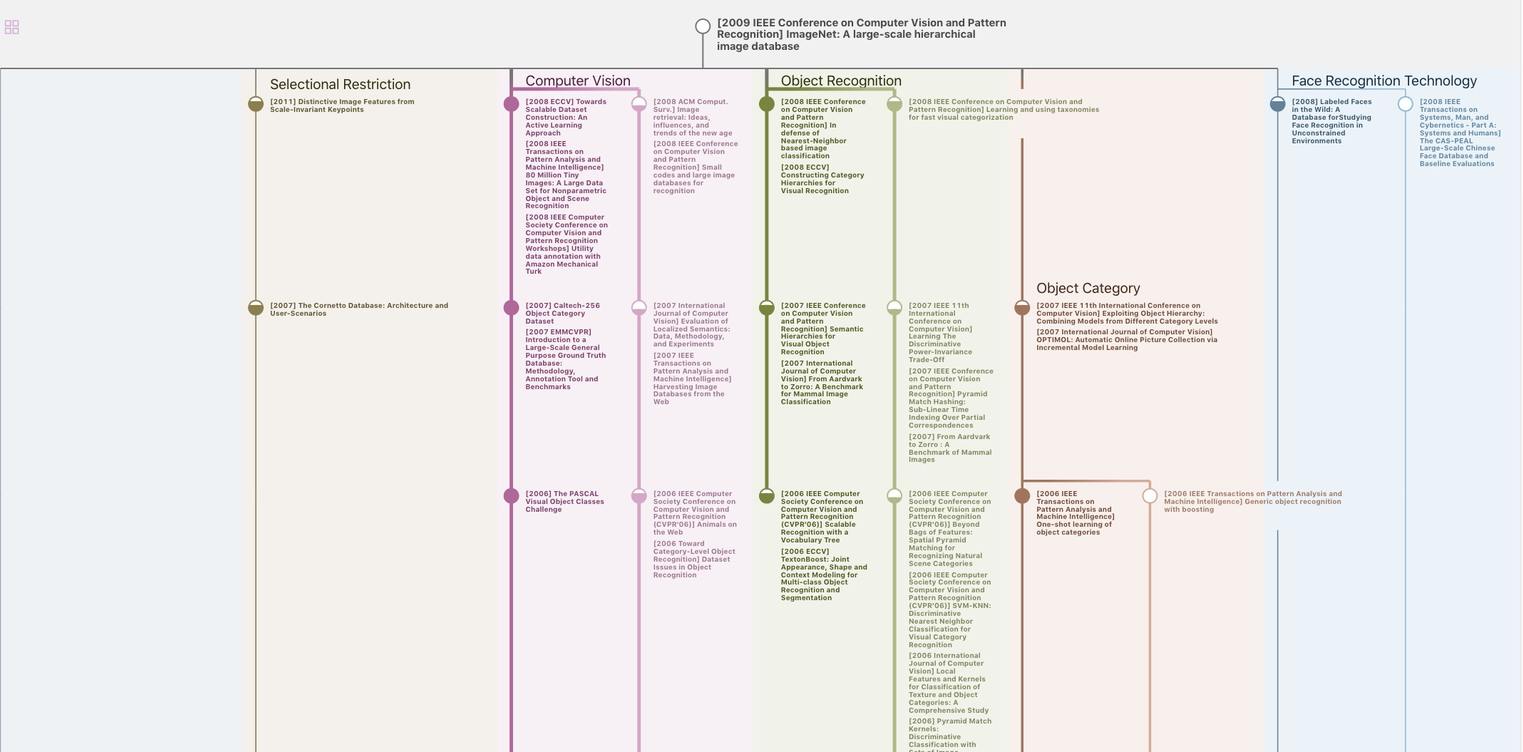
生成溯源树,研究论文发展脉络
Chat Paper
正在生成论文摘要