Investigating effective transfer of deep learning models from adults to children for lung ultrasound data analysis
2023 IEEE International Ultrasonics Symposium (IUS)(2023)
摘要
Automated assessment of LUS data from adult population is an ongoing research over the last few years. To this extent, various deep learning DL-based methods have been proposed to assess lung alterations caused by pneumonia. However, no work has been done in this regard to evaluate the LUS data from children for the presence/absence of consolidations due to pneumonia. Pediatric healthcare data acquisition in a resource constraint environment is a challenging task. As a result of which limited data is acquired. This prevents the effective training of DL models for the development of reliable computer aided assessment systems. To address this challenge, we employed pre-trained DL models, previously trained on LUS data from adults, and evaluated them over LUS data from children in a transfer learning (TL) setting. Two TL strategies i.e. linear probing (LP) and fine-tuning (FT) are utilized. The models are trained and tested in a leave-one-out cross validation manner with results showing that all the pre-trained models achieved higher classification performance for FT in comparison to LP. Among the models it is found that with the addition of spatial attention and custom loss to the base CNN, the model outperformed with the highest mean F1-Score of 81.9%. While evaluating the model’s performance w.r.t age, it is found that the more accurate the model, the less impact of age has on the underlying classification performance. These findings lay down the foundation for accurate consolidation classification in LUS data from children in a resource constraint environment
更多查看译文
关键词
Lung Ultrasound,Pediatric Ultrasound,Pneumonia,Resource-Limited Setting,Artificial Intelligence,Transfer Learning,Domain Adaptation
AI 理解论文
溯源树
样例
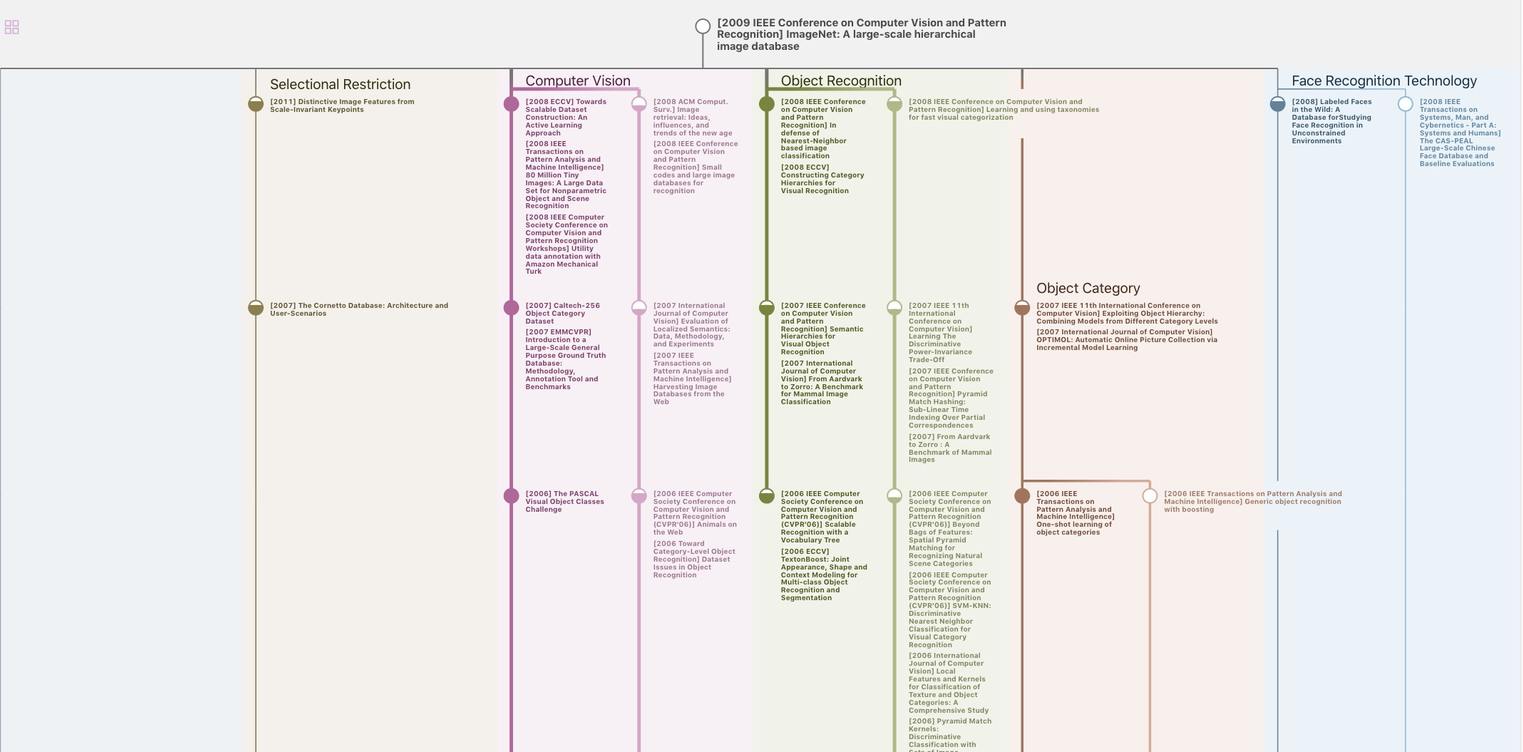
生成溯源树,研究论文发展脉络
Chat Paper
正在生成论文摘要