Neural Implicit Representation for Three-dimensional Ultrasound Carotid Surface Reconstruction using Unsigned Distance Function
2023 IEEE International Ultrasonics Symposium (IUS)(2023)
摘要
Accurate 3D geometric shapes of carotid arteries are imperative for the three-dimensional (3D) ultrasound (US) imaging to clinically assess the carotid atherosclerosis (CA). However, the traditional surface reconstruction method, such as iso-surface (ISO-SURF), suffers from the image noise, voxel resolution and additional processing. In this paper, we introduce the neural implicit representation based on the deep learning network for the 3D surface reconstruction of media-adventitia boundary (MAB), plaque, and lumen-intima boundary (LIB) together. The unsigned distance functions are learned by the network to generate the mesh. For the validation, six volumes were simulated in carotid shape with MAB and LIB, and random noise was added around the boundaries. The results showed that experiments on six simulated volumes illustrated better performance than ISO-SURF, with 47%, 36%, and 55% decrease in Chamfer distance, average absolute distance, and Hausdorff distance, respectively. The visualization results from the CA clinical data revealed a smoother and more continuous geometric surface than ISO-SURF. The comparison result has shown the potential of the proposed method to examine vascular pathologies in the future.
更多查看译文
关键词
Carotid atherosclerosis,3D ultrasound surface reconstruction,Unsigned distance functions,Neural network
AI 理解论文
溯源树
样例
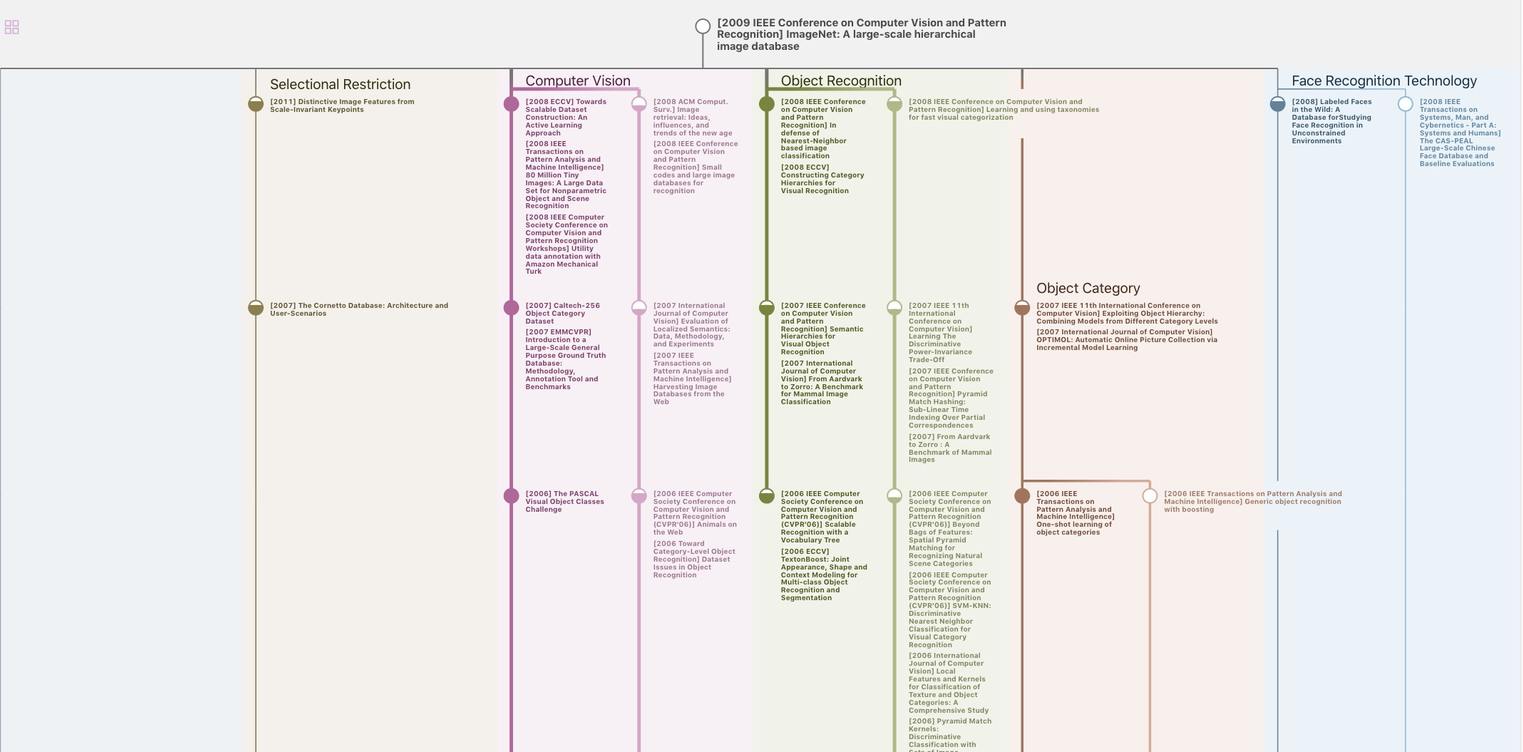
生成溯源树,研究论文发展脉络
Chat Paper
正在生成论文摘要