Robustness of ultrasound deep beamformers using low-energy adversarial perturbations
2023 IEEE International Ultrasonics Symposium (IUS)(2023)
摘要
As the use of ultrasound beamformers based on deep neural networks (DNNs) continues to gain popularity, it becomes increasingly important to assess their robustness. Traditional model-based beamformers are typically evaluated by studying factors such as sound speed variations, off-axis clutter, and other variables that challenge the underlying model. However, these conventional evaluation methods may not be well-suited for assessing model-free DNN-based beamformers. To address this challenge, we propose employing adversarial perturbations as a means of evaluating their robustness. Thus, we computed low-energy adversarial perturbations for two deep beamformers using the basic iterative method. We then assessed their performance when exposed to perturbed inputs using a contrast metric. Our results indicate that both of these deep beamformers are susceptible to these perturbations, whereas the traditional Delay-and-Sum (DAS) beamformer was little affected. Furthermore, one of the deep beamformers exhibited greater vulnerability to these perturbations, resulting in reduced performance compared to the other. Experimental results corroborated these findings, showing similar trends in phantom acquisitions. In summary, our findings emphasize the utility of adversarial perturbations as a valuable tool in assessing the robustness of deep neural network-based beamformers.
更多查看译文
关键词
Ultrasound beamforming,deep learning,adversarial perturbations,worst-case performance,robustness
AI 理解论文
溯源树
样例
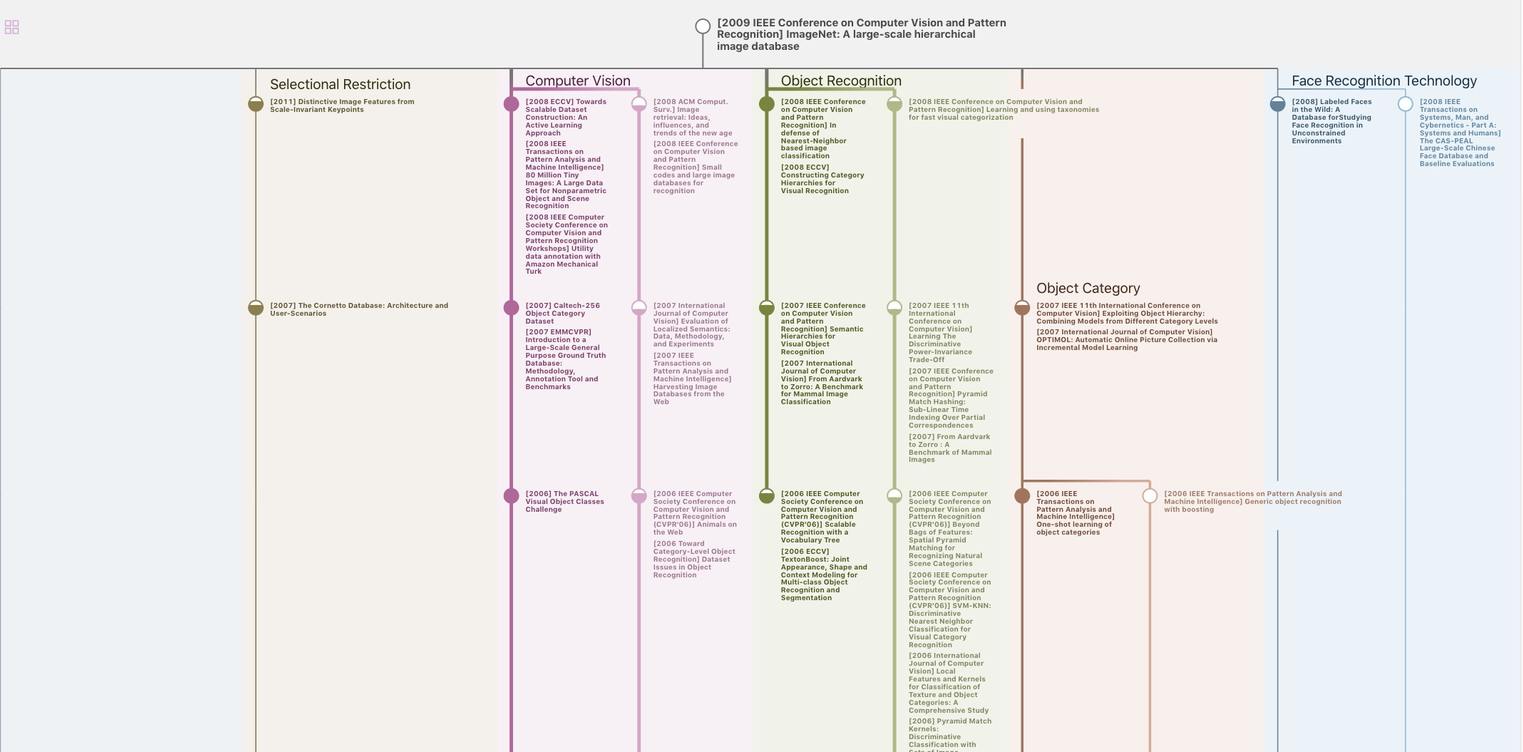
生成溯源树,研究论文发展脉络
Chat Paper
正在生成论文摘要