An Approach to Identifying Aspects of Positive Pilot Behavior within the Aviation Safety Reporting System
2023 IEEE/AIAA 42ND DIGITAL AVIONICS SYSTEMS CONFERENCE, DASC(2023)
摘要
The National Airspace System (NAS) is constantly evolving as air traffic continues to ramp up to pre-pandemic numbers and projected to grow to unprecedented levels in the coming years. As well as increasing demand to the current system, Emerging operations such as Unmanned Autonomous Systems are also expected to add to complexity to the airspace. To address these issues, the industry and government agencies supporting the NAS will need to rely upon additional automation and new technologies to address future operational requirements, while continuing to be a world-leading safe transportation system. As these new technologies are implemented, the system continues to rely on human pilots and controllers in the loop to monitor the system and intervene in situations the automation cannot handle. The goal of proactively addressing safety is of foremost concern to ensure passenger well-being. The industry has implemented various Safety Management Systems to identify safety risks and proactively address them before they result in a serious incident or accident. One such program is NASA's Aviation Safety Reporting System (ASRS). ASRS is a long-established system where pilots and controllers voluntarily and anonymously report safety incidents they experienced and observed during line operations by providing rich text narratives describing the events, the environment, and conditions leading to the safety event of concern. These narratives provide insight and context around events of interest and can be used to identify emerging problems. These reports can also trigger investigations within Flight Operational Quality Assurance or Flight Data Monitoring programs. However, this process typically focuses on the adverse events and the unsafe aspects of the operations surrounding the reported or detected events. This perspective of investigating factors that went wrong around an adverse event is commonly referred to as Safety I. Alternatively, characterizing successful actions that operators perform every day under varying conditions that keep the system within safe operating bounds is a concept referred to as Safety II. The benefit of the Safety II view is that its scope is much larger than that of Safety I since a vast majority of the operations result in successful flights. Many of the successful techniques used to manage operational threats are not documented in standard operating procedures or taught during training. They are typically acquired over time by working with experienced pilots during line operations or in many cases after experiencing a problem for the first time and reacting to it in situ, drawing from years of experience to manage the threat. In an attempt to quantify these positive actions, we are proposing an approach to extract key behaviors within ASRS reports that can support the Safety II concept. Our analysis assumes that ASRS reports contain some descriptions of corrective actions that operators performed to prevent a situation from becoming an accident. Leveraging recent advances in Natural Language Process (NLP) modeling, we have developed an approach to extract positive sentiment from reports, embed these positive statements in a vector space where they can be numerically analyzed, and clustering these statements into similar contextual categories. From these contextualized categories we can attempt to summarize and distill aspects of the positive behavior. The goal is to identify categories of behavior that describe consistent operator techniques that supports the Safety II concept. With this information, airlines may enable learning from these positive actions, or address procedures that need to be changed. These insights can provide a lens into what is "going right" in the operations that may otherwise not be known widely within the community. It is envisioned that this approach can be extended to other narrative programs such as Line Operation Safety Audit or Learning Improvement Team reports where similar observed behavior can be analyzed to extract positive actions and inform the overall operations.
更多查看译文
关键词
NLP,ASRS,Aviation Safety,Human Factors,Text Mining
AI 理解论文
溯源树
样例
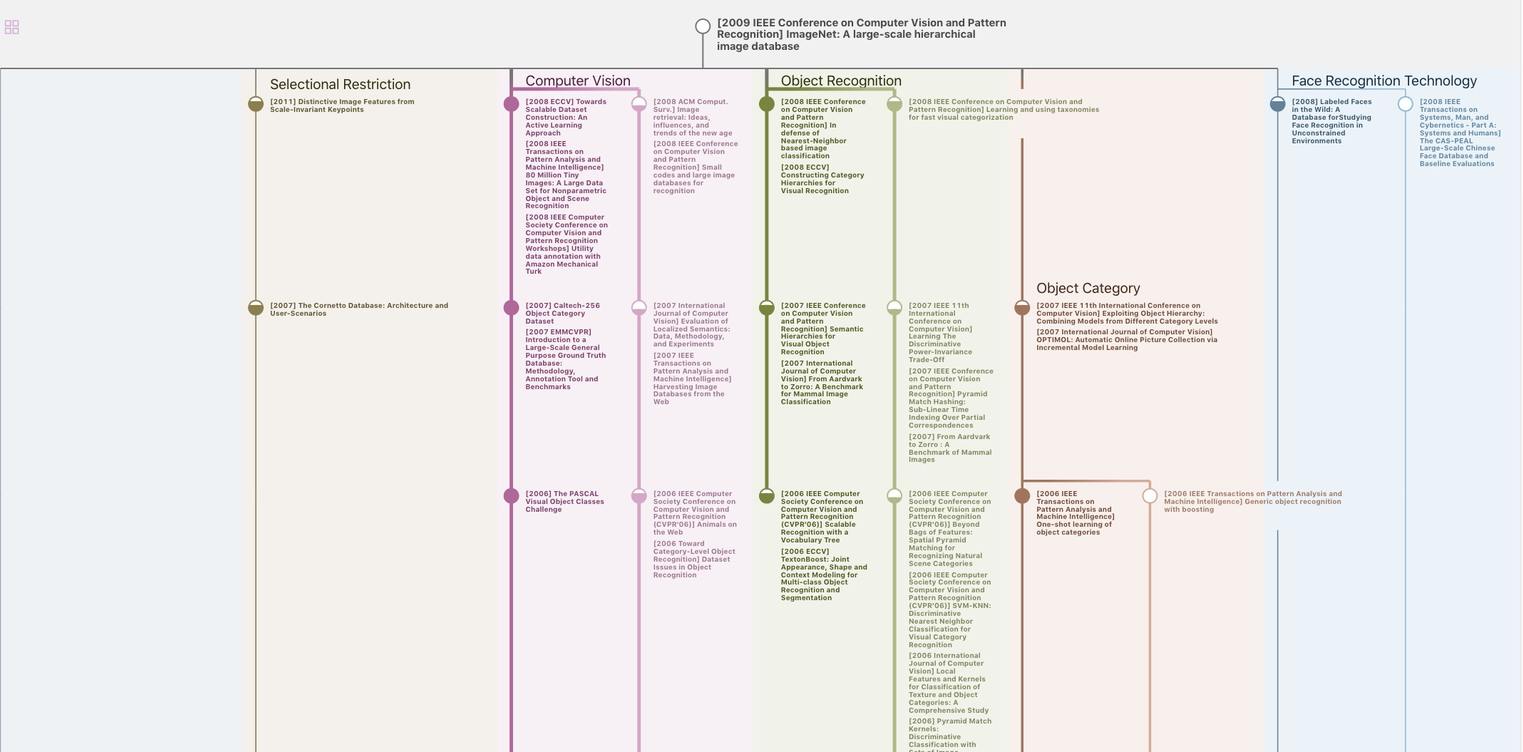
生成溯源树,研究论文发展脉络
Chat Paper
正在生成论文摘要