ESSNet: Lightweight Semantic Segmentation Network
2023 4th International Conference on Computer Engineering and Application (ICCEA)(2023)
摘要
Semantic segmentation is widely used in fields such as self-driving, robot navigation and augmented virtual reality, which has extremely high requirements for the real-time reasoning and accurate segmentation of the network. Recently, semantic segmentation has made great progress in segmentation accuracy, but little attention has been paid to real-time reasoning. Therefore, it makes sense to propose a network to balance accuracy and real-time reasoning. In this paper, we propose a lightweight semantic segmentation network with Multiple Correction Confusion Mechanism (MCCM) and Lightweight Attention Mechanisms (LAM), named Lightweight Semantic Segmentation Network (ESSNet). MCCM selectively incorporates low-level feature to make the network segmentation contour clearer. LAM effectively captures the context information, making the feature representation more abundant. In general, different scale features confusion, and efficient attention mechanisms are adopted by LCANet to significantly improve its performance. To demonstrate the effectiveness of the network, we conducted experiments on the Cityscapes and CamVid datasets, and obtained MIOU of 68.7
%
and 74.8%, respectively, which is comparable to the state-of-the-art methods under the same experimental settings. In addition, on a single Titan RTX card, the network reached a forward reasoning speed of 136.4 FPS (480x480x3 image resolution).
更多查看译文
关键词
Attention Mechanism,Semantic Segmentation,Different Scale Features Confusion,Real-time Reasoning
AI 理解论文
溯源树
样例
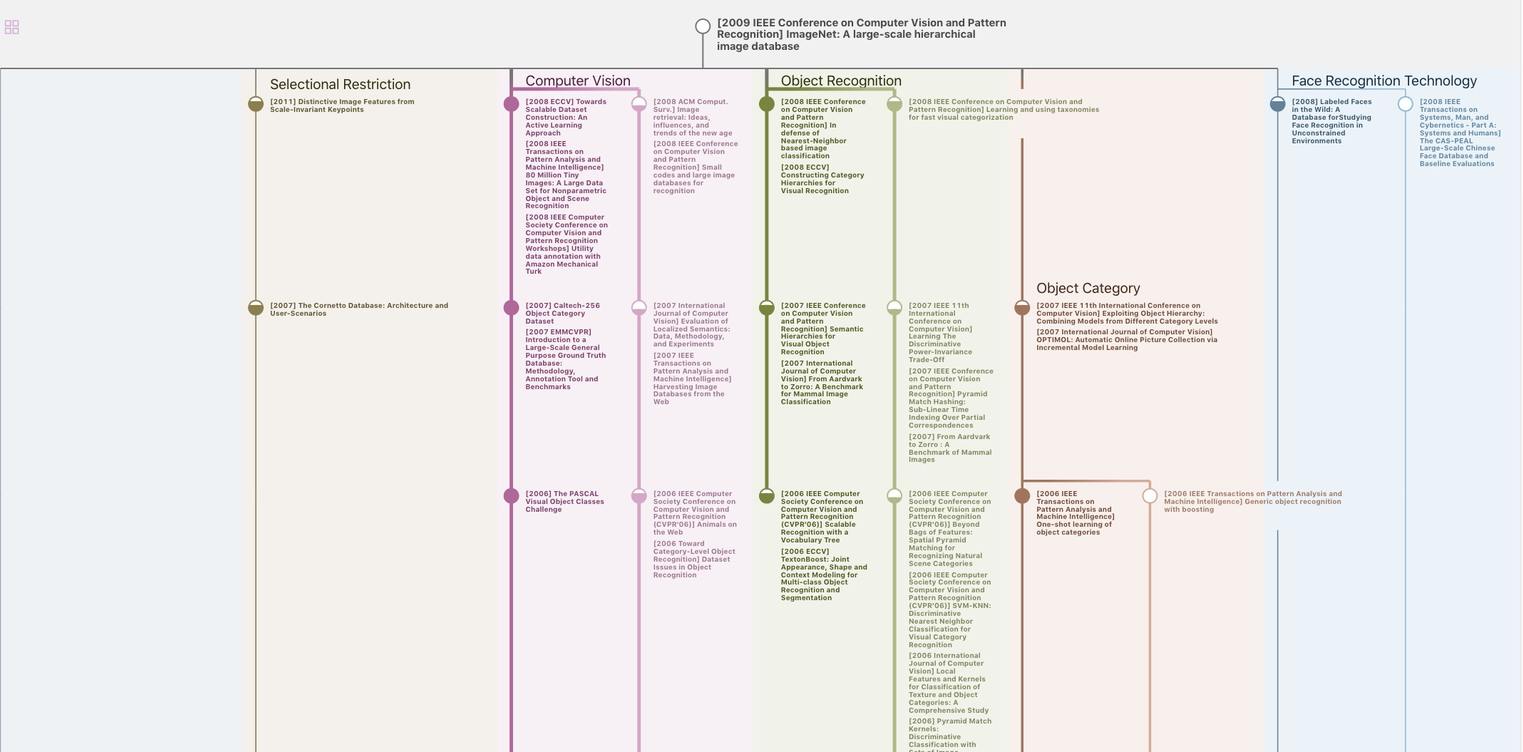
生成溯源树,研究论文发展脉络
Chat Paper
正在生成论文摘要