Irecut+MM: Data Generalization and Metric Improvement for Few-shot Learning
2023 IEEE International Conference on Multimedia and Expo (ICME)(2023)
摘要
Few-shot learning is intended to address situations where there are few training samples per class. The main challenge is how to adequately extend the data or identify tighter associations from the sparse data. In this study, the final classification and data from few-shot learning are taken into account. First, we provide an efficient regularization to internal network generalization to accomplish data augmentation. Second, to enhance the Nearest Class Mean classifier (NCM) and soft K-means methods for classification, we raise a new regularized estimator based on the concept of Mahalanobis distance. We conduct extensive experiments based on CIFAR-FS and FC100. The ablation experiments show that the proposed data augmentation method is 2% higher than the baseline on CIFAR-FS(1-shot), and the classification algorithm is equally effective. The comparison experiments reveal results that much exceed the vast majority of state-of-the-art performance measures.
更多查看译文
关键词
Few-shot Learning,Data Enhancement,Classification
AI 理解论文
溯源树
样例
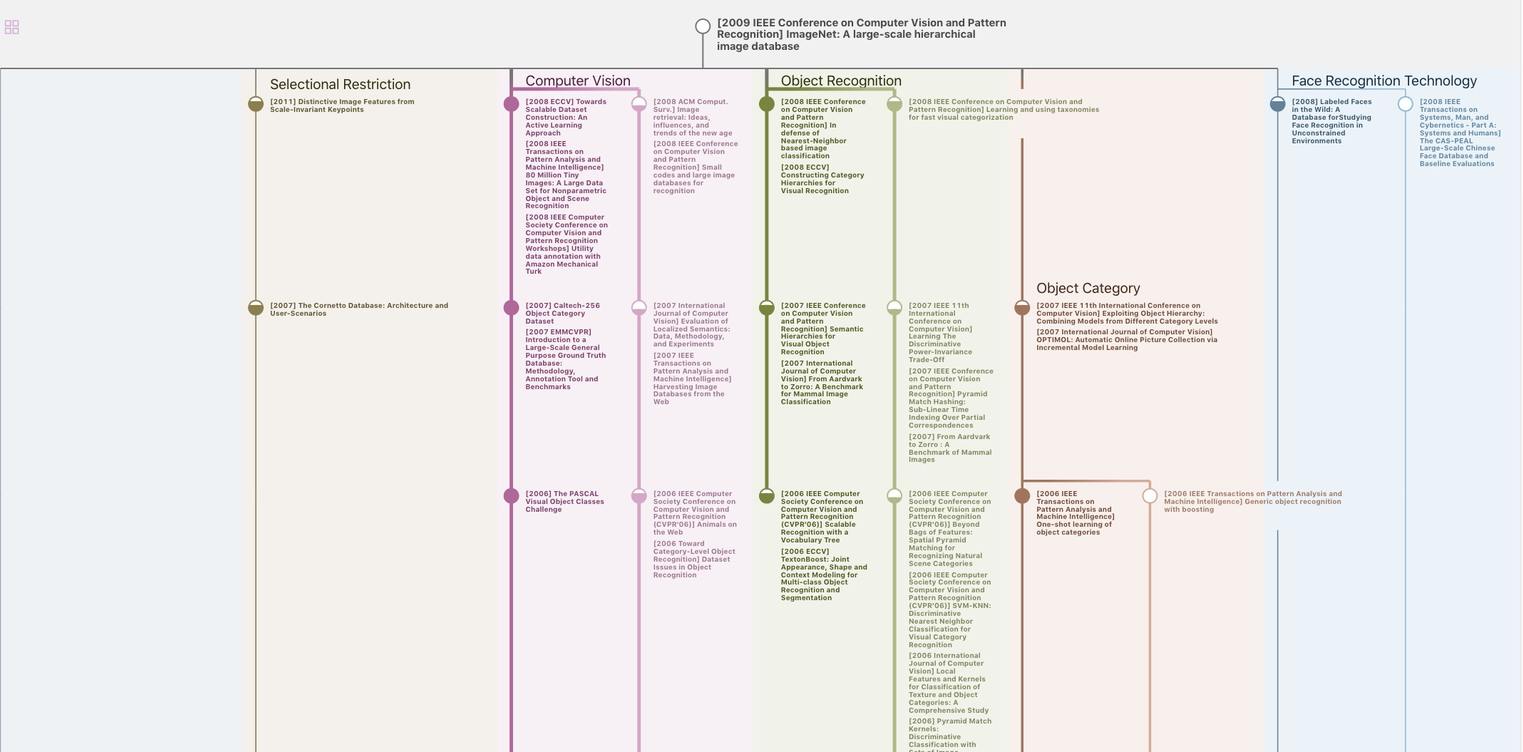
生成溯源树,研究论文发展脉络
Chat Paper
正在生成论文摘要