Post-training Quantization or Quantization-aware Training? That is the Question
2023 China Semiconductor Technology International Conference (CSTIC)(2023)
摘要
Quantization has been demonstrated to be one of the most effective model compression solutions that can potentially be adapted to support large models on a resource-constrained edge device while maintaining a minimal power budget. There are two forms of quantization: post-training quantization (PTQ) and quantization-aware training (QAT). The former starts from a trained model with floating-point computation and then gets quantized afterward, while the latter compensates for the quantization-related errors by training the neural network using the quantized version in the forward pass during training. Though QAT is able to produce accuracy benefits, it suffers from a long training process and less flexibility during deployment. Traditionally, researchers usually make the one-time bold decision between QAT and PTQ depending on the quantized bit-width and hardware requirement. In this work, we observed that even though the hardware cost is approximately the same for various quantization schemes, the sensitivity to training for each quantized layer is different. This leads to that certain scheme requires QAT more than others. We argue that it is necessary to look into this dimension by measuring the accuracy difference for each layer under QAT and PTQ conditions. In this paper, we introduce a methodology to provide a systematic and explainable way to quantity the tradeoffs between the quantization forms. This is especially beneficial for evaluating a layer-wise mixed-precision quantization (MPQ) scheme, where different bit-widths across are allowed and the search space is enormous.
更多查看译文
关键词
effective model compression solutions,floating-point computation,hardware requirement,layer-wise mixed-precision quantization,MPQ,neural network,one-time bold decision,post-training quantization,PTQ,QAT,quantization-aware training,quantization-related errors,quantized bit-width,resource-constrained edge device
AI 理解论文
溯源树
样例
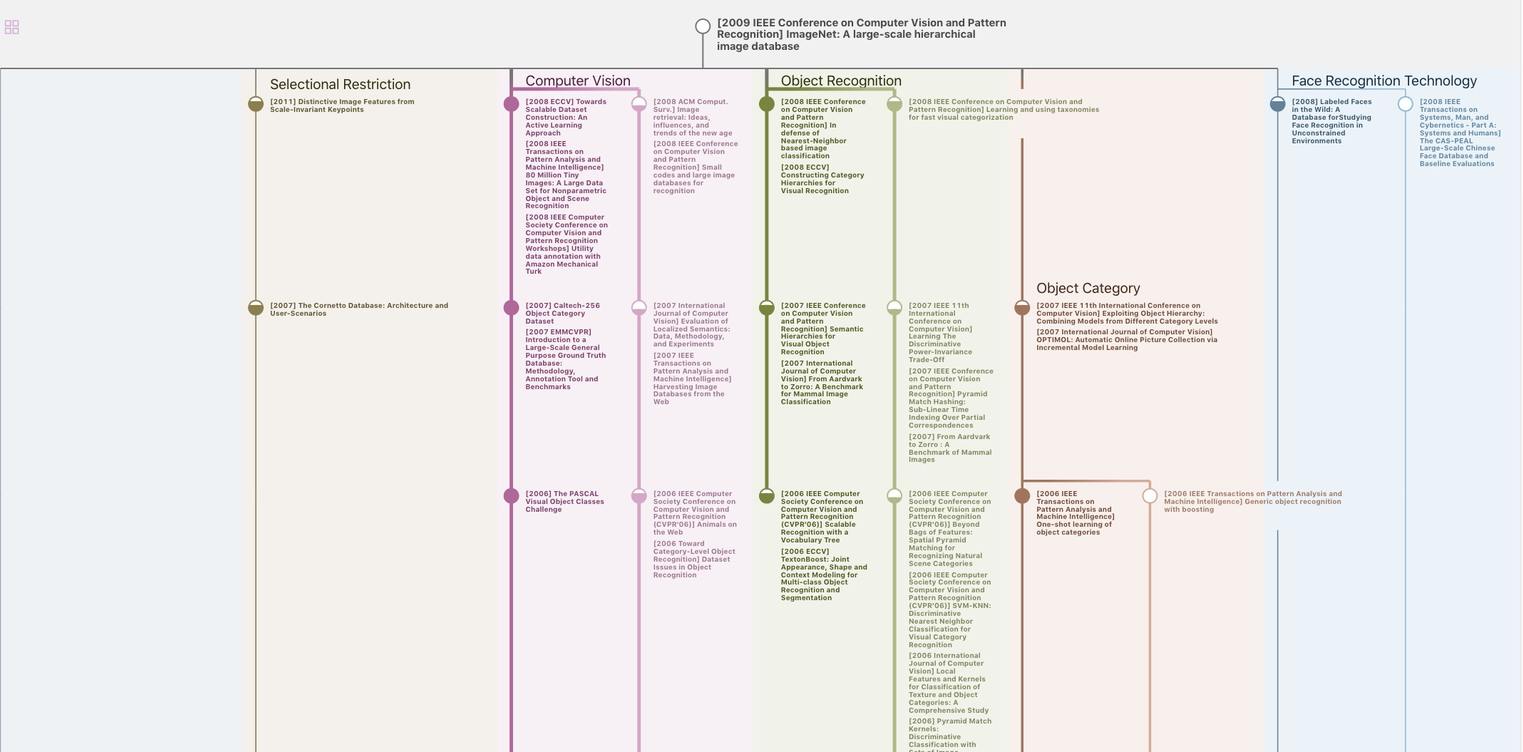
生成溯源树,研究论文发展脉络
Chat Paper
正在生成论文摘要