Bayesian multitemporal hyperspectral image unmixing using scale-dependent distribution
2023 4th International Seminar on Artificial Intelligence, Networking and Information Technology (AINIT)(2023)
摘要
The main changellage of multitemporal hyperspectral image (MTHS) unmixing is modeling the variation of the spectra. In this paper, by considering the spectral variability as an additional term of the endmember, a hierarchical bayesian MTHS unmixing model is established via multi-scaless wavelet analysis, in which the prior of the endmember and its variability is the core concern. The low frequency wavelet coefficients of the endmember and its variability are both designed according to their non-informative propotites. Furthermore, the interscale correlationship of high frequency coefficients of the endmember and its variability is fully exploited, resulting in the scale-dependent prior. In addition, the joint posterior distribution is inferred and solved by a Markov chain Monte Carlo (MCMC) sampling. Experimental results show that the propose unmixing method can accurately capture the variation of the spectra and estimate of the abundance.
更多查看译文
关键词
Multitemporal hyperspectral,bayesian unmixing,endmember variability,multi-scaless wavelet
AI 理解论文
溯源树
样例
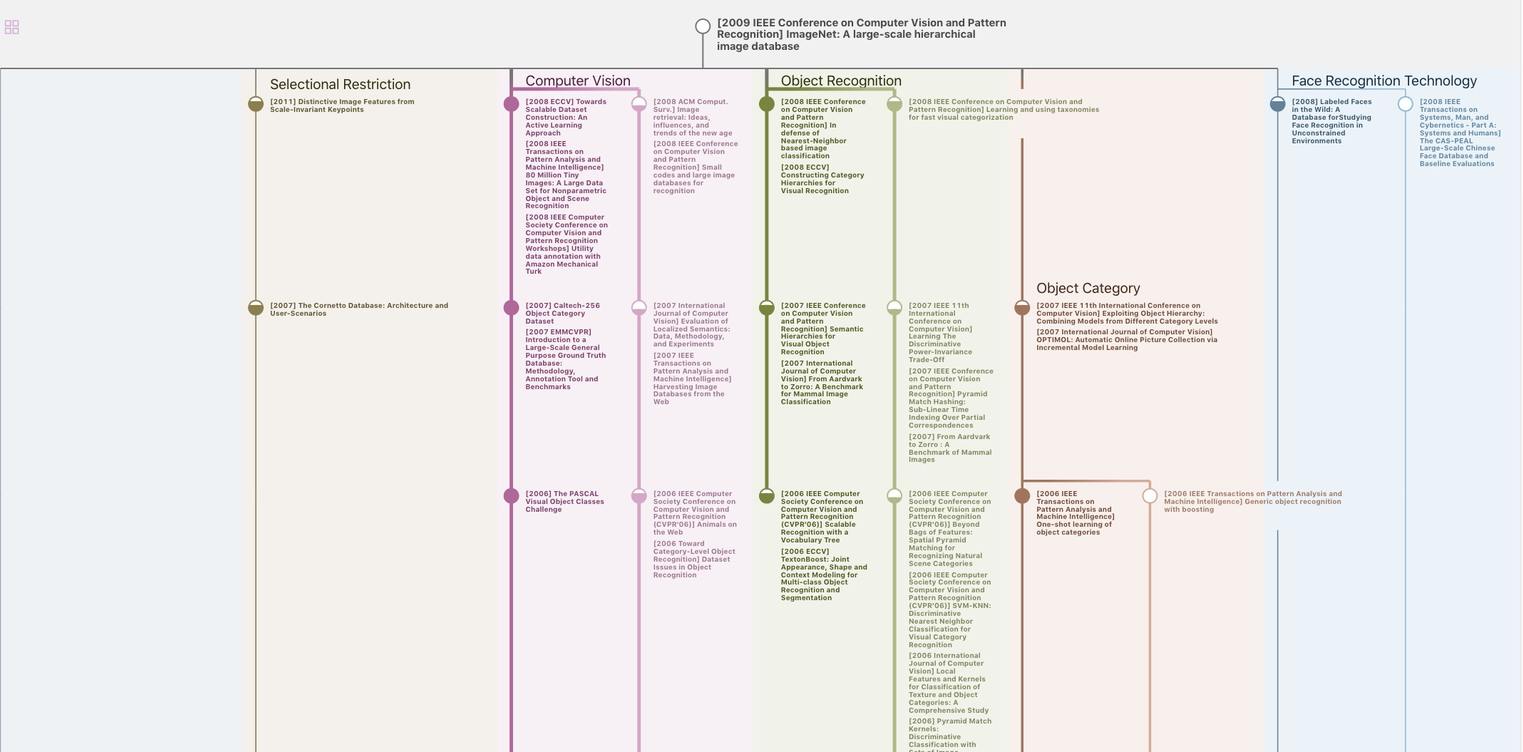
生成溯源树,研究论文发展脉络
Chat Paper
正在生成论文摘要