Learning Maximum Margin Channel Decoders for Non-linear Gaussian Channels
2022 IEEE International Symposium on Information Theory (ISIT)(2022)
摘要
The problem of learning a channel decoder for an unknown non-linear white Gaussian noise channel is considered. The learner is provided with a fixed codebook and a dataset comprised of n independent input-output samples of the channel, and is required to select a matrix for a nearest neighbor decoder with a linear kernel. The objective of maximizing the margin of the decoder is addressed. Accordingly, a regularized loss minimization problem with a codebook-related regularization term and a hinge-like loss function is developed, which is inspired by the support vector machine paradigm for classification problems. Expected generalization error bound for that hinge loss is provided for the solution of the regularized loss minimization, and shown to scale at a rate of O(1/(λn)), where λ is a regularization tradeoff parameter. In addition, a high probability uniform generalization error bound is provided for the hypothesis class, and shown to scale at a rate of $O(1/\sqrt n )$. A stochastic sub-gradient descent algorithm for solving the regularized loss minimization problem is proposed, and an optimization error bound is stated, which scales at a rate of $\tilde O(1/(\lambda T))$. The performance of the this algorithm is demonstrated by an example.
更多查看译文
关键词
fixed codebook,nearest neighbor decoder,linear kernel,regularized loss minimization problem,codebook-related regularization term,hinge-like loss function,support vector machine paradigm,classification problems,expected generalization error,hinge loss,regularization tradeoff parameter,high probability uniform generalization error,maximum margin channel decoders,nonlinear Gaussian channels,channel decoder,nonlinear white Gaussian noise channel
AI 理解论文
溯源树
样例
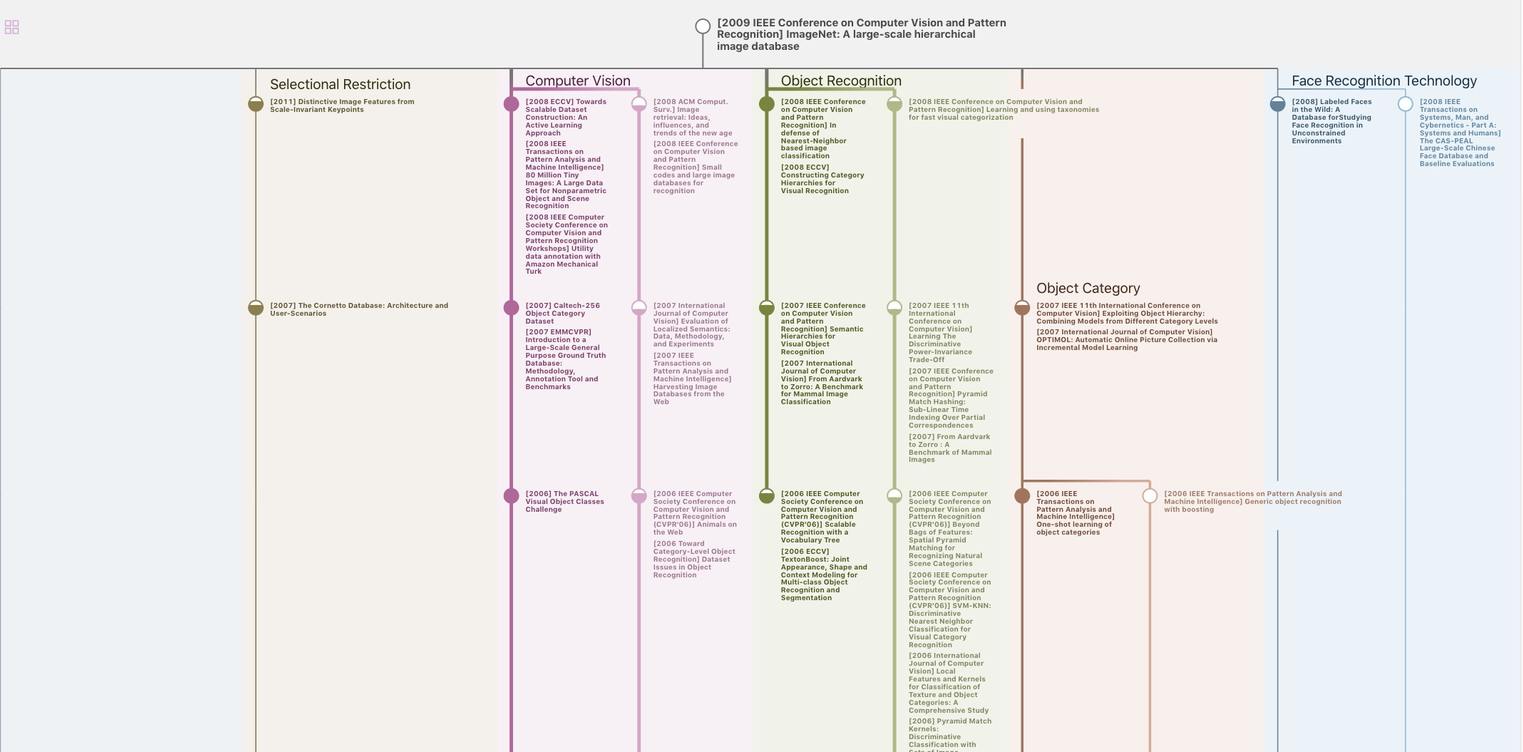
生成溯源树,研究论文发展脉络
Chat Paper
正在生成论文摘要