Machine Learning Classification of Riemannian Tangent Spaces Based on MI-BCI
2023 IEEE International Conference on Mechatronics and Automation (ICMA)(2023)
摘要
Motor imagery brain-computer interfaces (BCIs) have been extensively used in stroke rehabilitation, particularly in China where stroke incidence is high. The application of BCI to control prostheses and robotic arms has shown promising results in improving the quality of life for stroke patients. However, a major challenge in this field is improving the accuracy of motor imagery (MI) classification. Several previous studies have reported that stroke patients exhibit atypical patterns of even-trelated desynchronization (ERD) and event-related synchronization (ERS) activation during motor imagery (MI) tasks. It has been suggested that the brain regions affected by stroke may show reduced excitability and relative insensitivity to external interference compared to those of healthy individuals. Therefore, this study proposes that the classification accuracy of stroke patients in the same MI task would be higher compared to that of healthy volunteers. In this study, we employed a feature extraction algorithm that combines the Filter Bank Common Spatial Pattern (FBCSP) and Riemann geometric mean distance methods to compare the classification accuracy of five different classifiers, with the objective of identifying the most suitable classifier for our analysis. The obtained results revealed that the K-Nearest Neighbors (KNN) classifier yielded the highest accuracy, reaching 87.5% for stroke patients and 85% for healthy volunteers, respectively. In addition to accuracy, we also utilized Kappa values as evaluation metrics, which yielded Kappa results of 0.619 and 0.613 for stroke patients and healthy volunteers, respectively.
更多查看译文
关键词
BCI,Stroke,FBCSP,Riemannian GMD,Kappa score
AI 理解论文
溯源树
样例
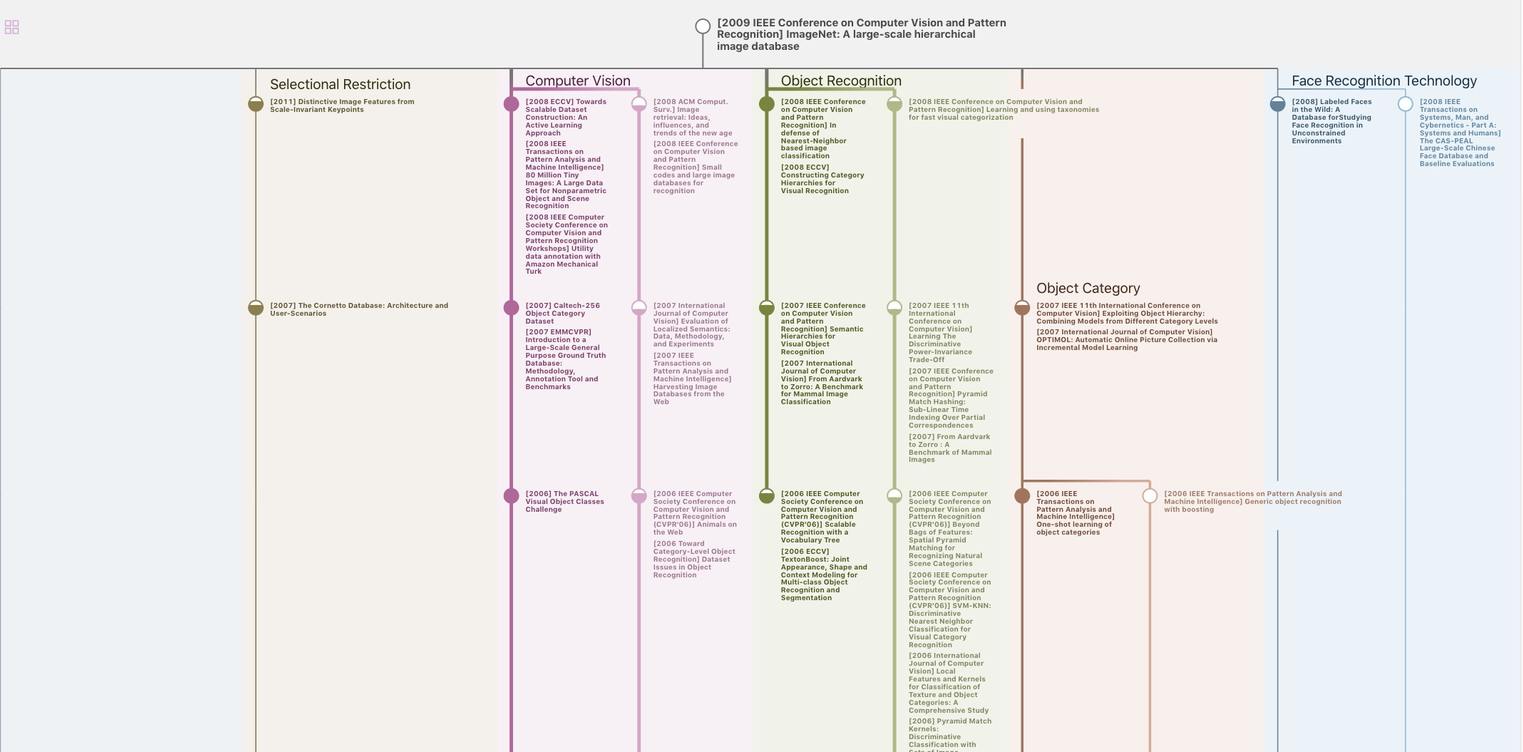
生成溯源树,研究论文发展脉络
Chat Paper
正在生成论文摘要