Point Density-aware Channel-wise Transformer for LiDAR 3D Object Detection
2023 IEEE International Conference on Mechatronics and Automation (ICMA)(2023)
摘要
We present a two-stage 3D object detection framework from point clouds, named Point Density-aware Channel-wise Transformer (PD-CT3D), which investigate the property of point density. This architecture uses 3D sparse CNN to effectively generate high-quality proposals at the first stage, then integrates the inherent property of point density and 3D CNN-based voxel features by density-aware proposal grid pooling. Specifically, each generated proposal from the first stage is divided into grids to aggregate corresponding voxel-wise features and raw point-based features for encoding the representative features from the whole scene. Subsequently, a channel-wise encoder-decoder transformer is adopted to extract the encoded density-aware features and decode them into a global representation for final refinement. Experiments on the widely used KITTI dataset show that the PD-CT3D achieves competitive performance among state-of-the-art methods.
更多查看译文
关键词
3D Object Detection,Point Cloud,Transformer
AI 理解论文
溯源树
样例
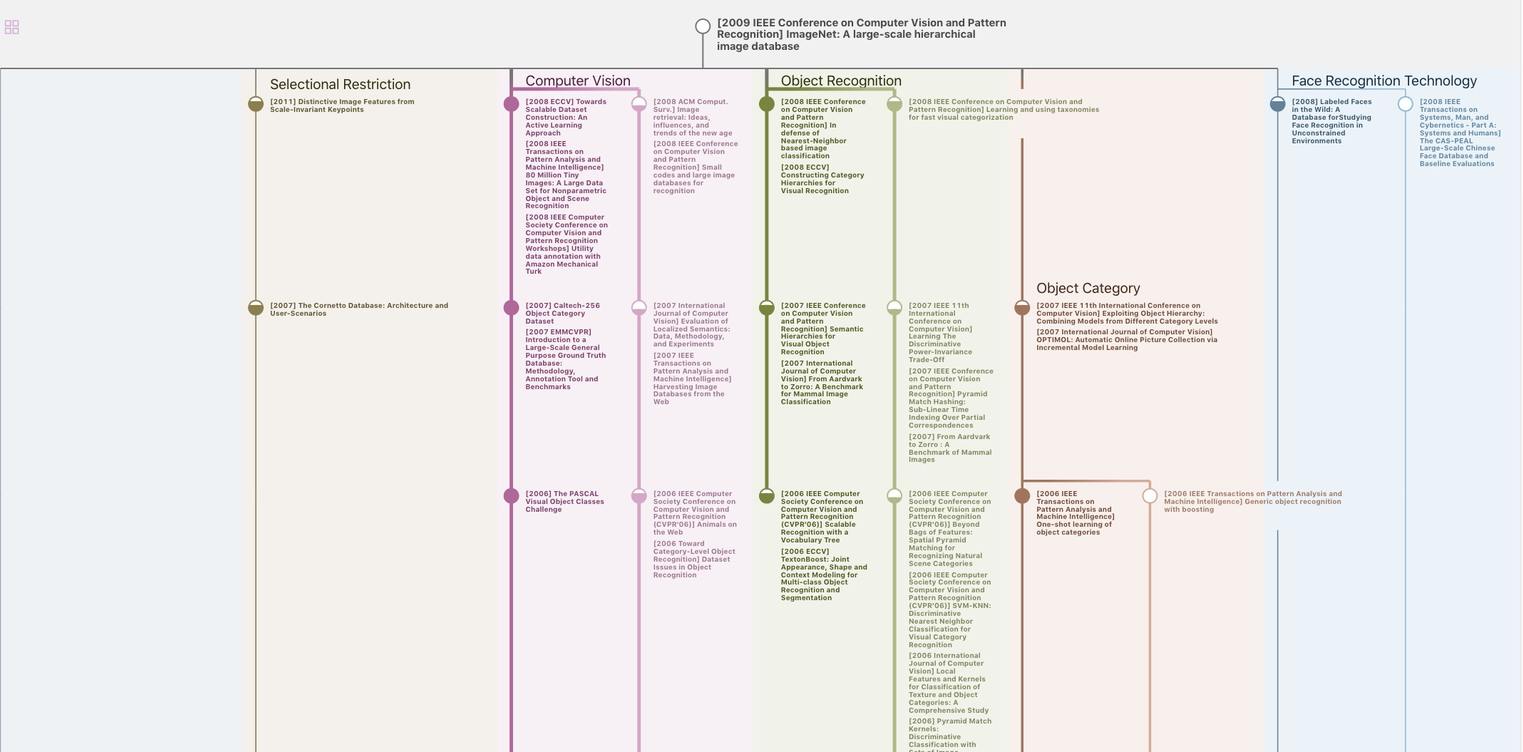
生成溯源树,研究论文发展脉络
Chat Paper
正在生成论文摘要