Optimal Parameters in Spiking Neurons Make Better Image Classification
2023 3rd International Symposium on Computer Technology and Information Science (ISCTIS)(2023)
摘要
In deep learning, there are many researches on image classification. However, for the traditional Artificial Neural Network (ANN), it is not suitable for some low-power edge devices because of the high energy consumption mainly for a large number of floating-point operations. As the third-generation neural network, Spiking Neural Network (SNN) can avoid high-precision floating-point multiplications due to its pulse mechanism, thereby achieving low power consumption. At the same time, SNN can extract spatio-temporal features and perform well on processing some event-based image sequences. However, formulating efficient and high-performance learning algorithms for SNN remains challenging. Most learning methods only learn the network weights and ignore the optimization of some parameters in the spiking neurons. In this paper, we draw inspiration from the observation that membrane-related parameters differ across brain regions and propose a decoupled spike parameters (DSP) training algorithm that simultaneously learns network weights and membrane potential time constant of SNN by solving a bilevel optimization problem. Our method achieves state-of-the-art on various classification tasks, including the CIFAR, DVS128 Gesture, and CIFAR10DVS datasets. Overall, DSP shows promise in image classification tasks for SNNs, providing a new paradigm for future high-performance SNN training.
更多查看译文
关键词
component,deep learning,image classification,spiking neural networks,bilevel optimization
AI 理解论文
溯源树
样例
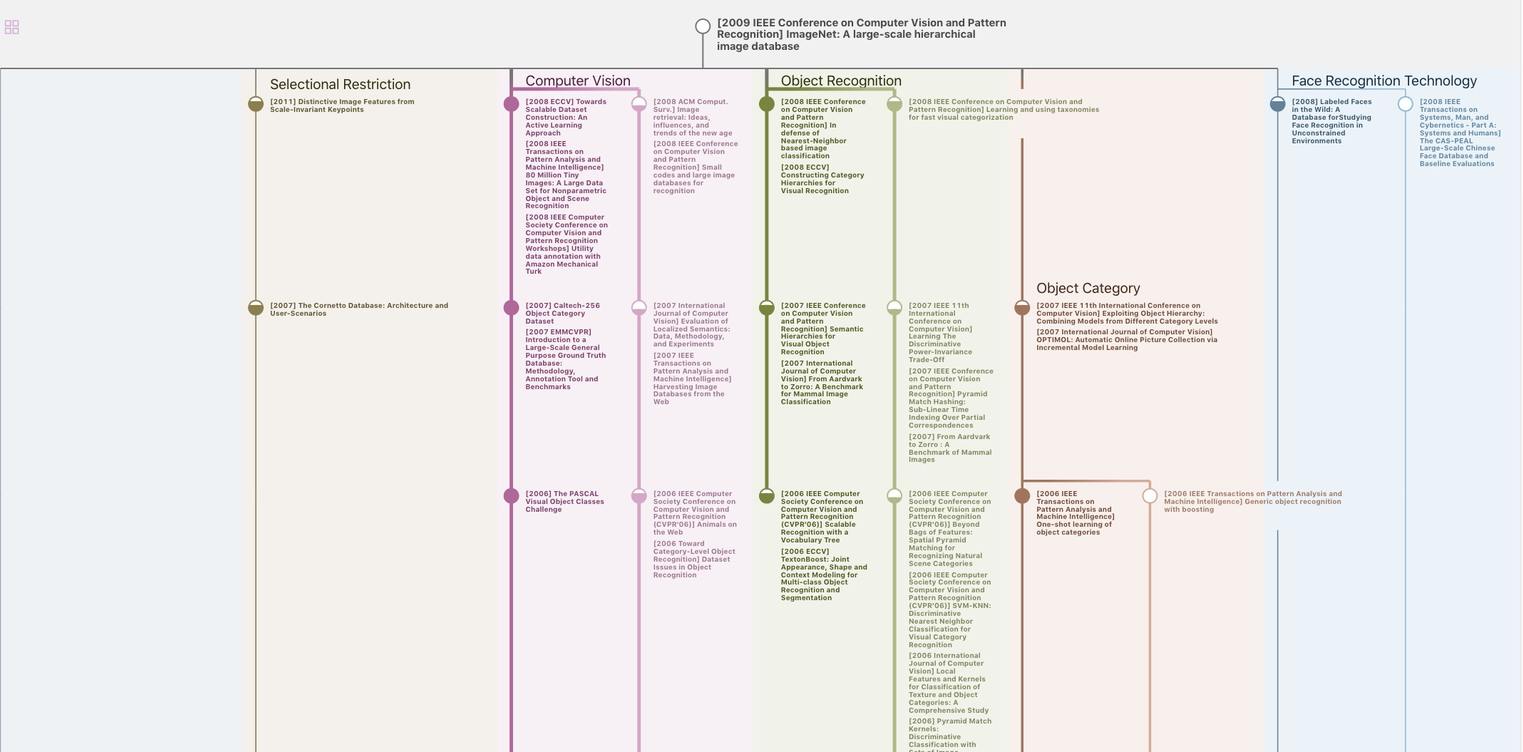
生成溯源树,研究论文发展脉络
Chat Paper
正在生成论文摘要