Imbalanced Radar Micro-Motion Target Classification Based on K-Means SMOTE and Deep Residual Network
2023 3rd International Symposium on Computer Technology and Information Science (ISCTIS)(2023)
摘要
In the practical application of radar target classification based on deep learning, there are problems such as incomplete and imbalanced radar datasets making it difficult for deep learning to leverage its advantages. Based on these problems, an imbalanced radar micro-motion target classification method based on k-means SMOTE and deep residual network is proposed. Firstly, based on the imbalance of various target samples collected in practice, in order to make full use of the micro-motion features of targets, the K-means SMOTE algorithm is proposed to optimize and balance training datasets. Then, accurate classification of micro-motion radar targets is achieved based on the residual network, which uses the ResNeXt101 network as the core structure. Finally, based on measured radar target data, experimental verification is conducted. The experimental results show that compared to traditional deep learning direct object classification methods, the algorithm proposed in this paper can more effectively address the problem of data imbalance and achieve higher classification accuracy.
更多查看译文
关键词
radar target classification,deep residual network,k-means SMOTE
AI 理解论文
溯源树
样例
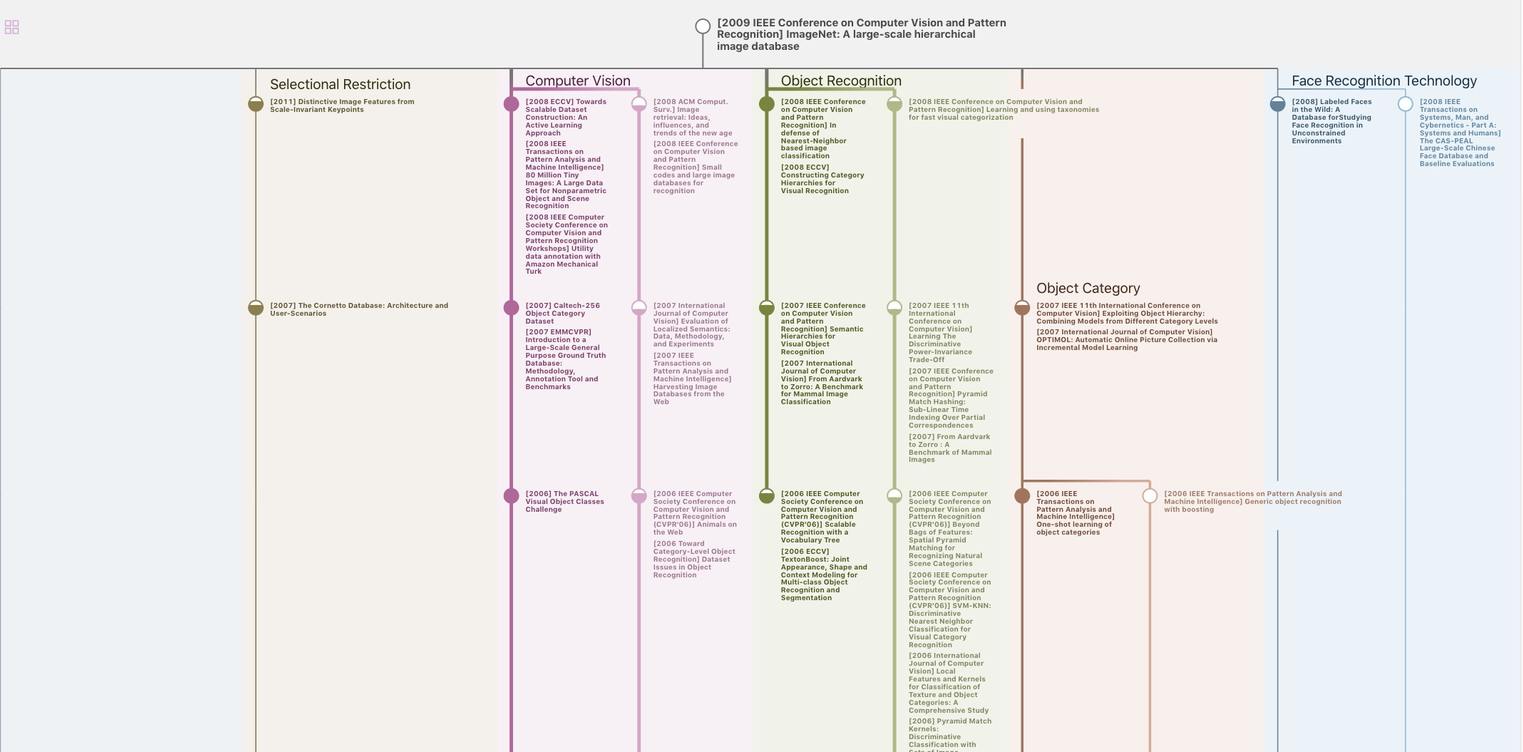
生成溯源树,研究论文发展脉络
Chat Paper
正在生成论文摘要